Online Supervised Training of Spaceborne Vision During Proximity Operations Using Adaptive Kalman Filtering
ICRA 2024(2024)
摘要
This work presents an Online Supervised Training (OST) method to enable robust vision-based navigation about a non-cooperative spacecraft. Spaceborne Neural Networks (NN) are susceptible to domain gap as they are primarily trained with synthetic images due to the inaccessibility of space. OST aims to close this gap by training a pose estimation NN online using incoming flight images during Rendezvous and Proximity Operations (RPO). The pseudo-labels are provided by an adaptive unscented Kalman filter where the NN is used in the loop as a measurement module. Specifically, the filter tracks the target’s relative orbital and attitude motion, and its accuracy is ensured by robust on-ground training of the NN using only synthetic data. The experiments on real hardware-in-the-loop trajectory images show that OST can improve the NN performance on the target image domain given that OST is performed on images of the target viewed from a diverse set of directions during RPO.
更多查看译文
关键词
Space Robotics and Automation,Deep Learning for Visual Perception,Continual Learning
AI 理解论文
溯源树
样例
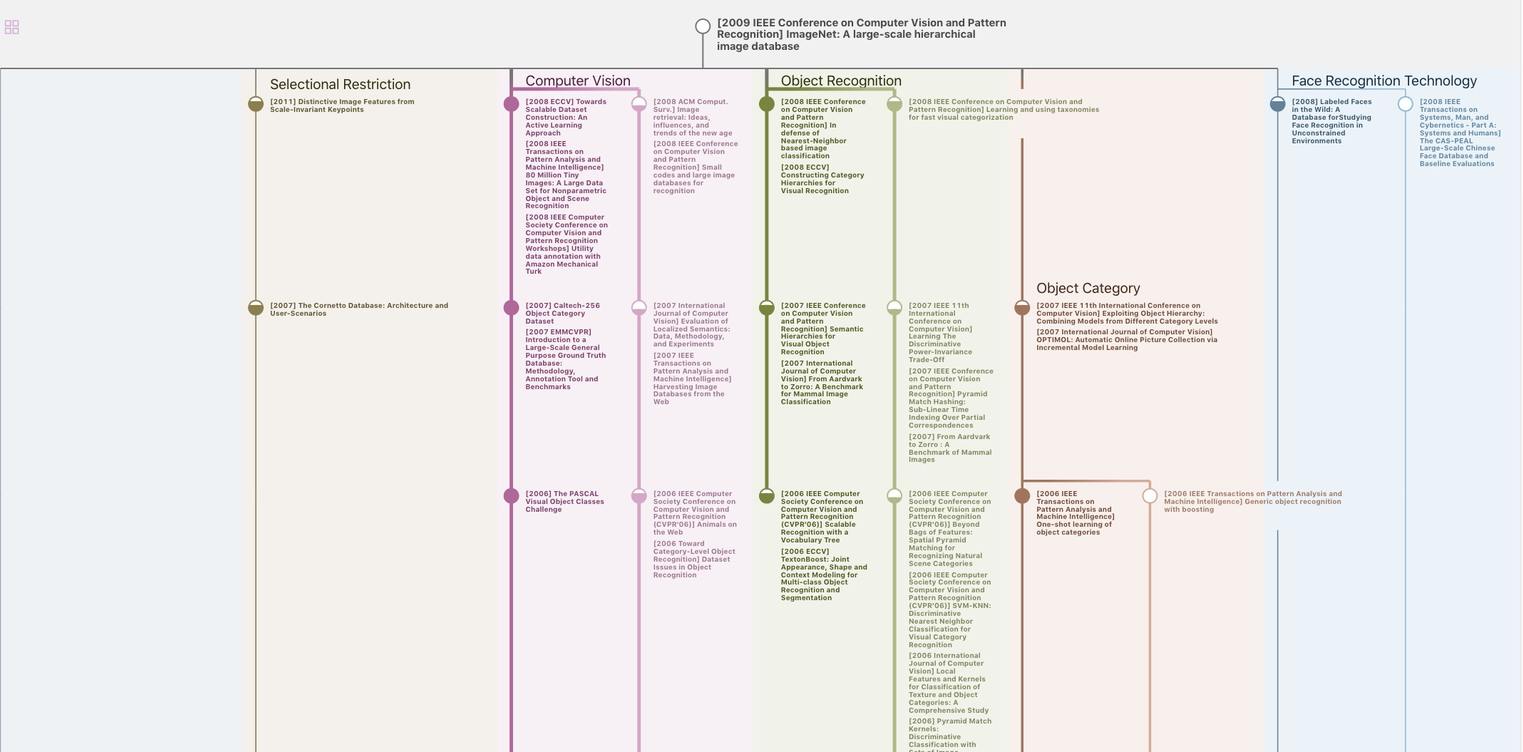
生成溯源树,研究论文发展脉络
Chat Paper
正在生成论文摘要