Unpaired MRI Super Resolution with Contrastive Learning
2024 IEEE International Symposium on Biomedical Imaging (ISBI)(2024)
摘要
Magnetic resonance imaging (MRI) is crucial for enhancing diagnostic accuracy in clinical settings. However, the inherent long scan time of MRI restricts its widespread applicability. Deep learning-based image super-resolution (SR) methods exhibit promise in improving MRI resolution without additional cost. Due to lacking of aligned high-resolution (HR) and low-resolution (LR) MRI image pairs, unsupervised approaches are widely adopted for SR reconstruction with unpaired MRI images. However, these methods still require a substantial number of HR MRI images for training, which can be difficult to acquire. To this end, we propose an unpaired MRI SR approach that employs contrastive learning to enhance SR performance with limited HR training data. Empirical results presented in this study underscore significant enhancements in the peak signal-to-noise ratio and structural similarity index, even when a paucity of HR images is available. These findings accentuate the potential of our approach in addressing the challenge of limited HR training data, thereby contributing to the advancement of MRI in clinical applications.
更多查看译文
关键词
Magnetic resonance imaging,super-resolution,contrastive learning,unsupervised,limited HR training data
AI 理解论文
溯源树
样例
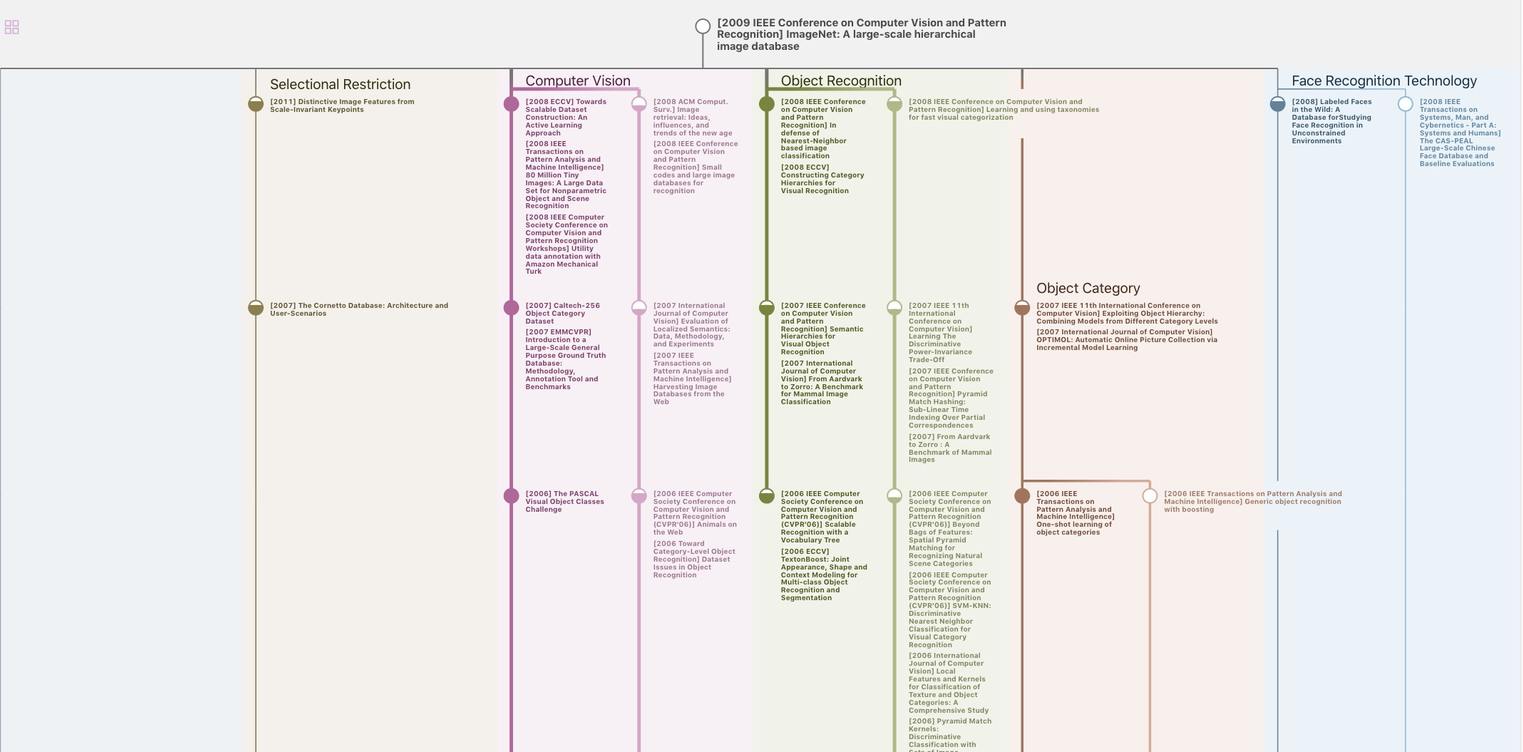
生成溯源树,研究论文发展脉络
Chat Paper
正在生成论文摘要