44. Combining Crop Growth Modeling, Active Sensing and Machine Learning to Improve In-Season Nitrogen Management of Maize
Precision Agriculture(2023)
摘要
This study aimed to develop and evaluate an in-season nitrogen (N) management strategy for maize (Zea mays L.) by combining crop growth modeling, active canopy sensing and machine learning. At V8 stage, the CERES-Maize model was first used with weather data fusion to predict in-season economic optimal side-dress N rate (EOSN). Active canopy sensing data was fused with other related data using machine learning regression to diagnose maize N status. The predicted EOSN was adjusted based on estimated maize N status. Compared to the predicted EOSN based on modeling only, the recommended EOSN based on the new strategy showed higher correlation (R2=0.70) and lower difference (10.36 kg/ha) with the measured values. It is concluded that the combination of crop growth modeling, active canopy sensing and machine learning is a promising strategy for in-season site-specific N management of maize.
更多查看译文
AI 理解论文
溯源树
样例
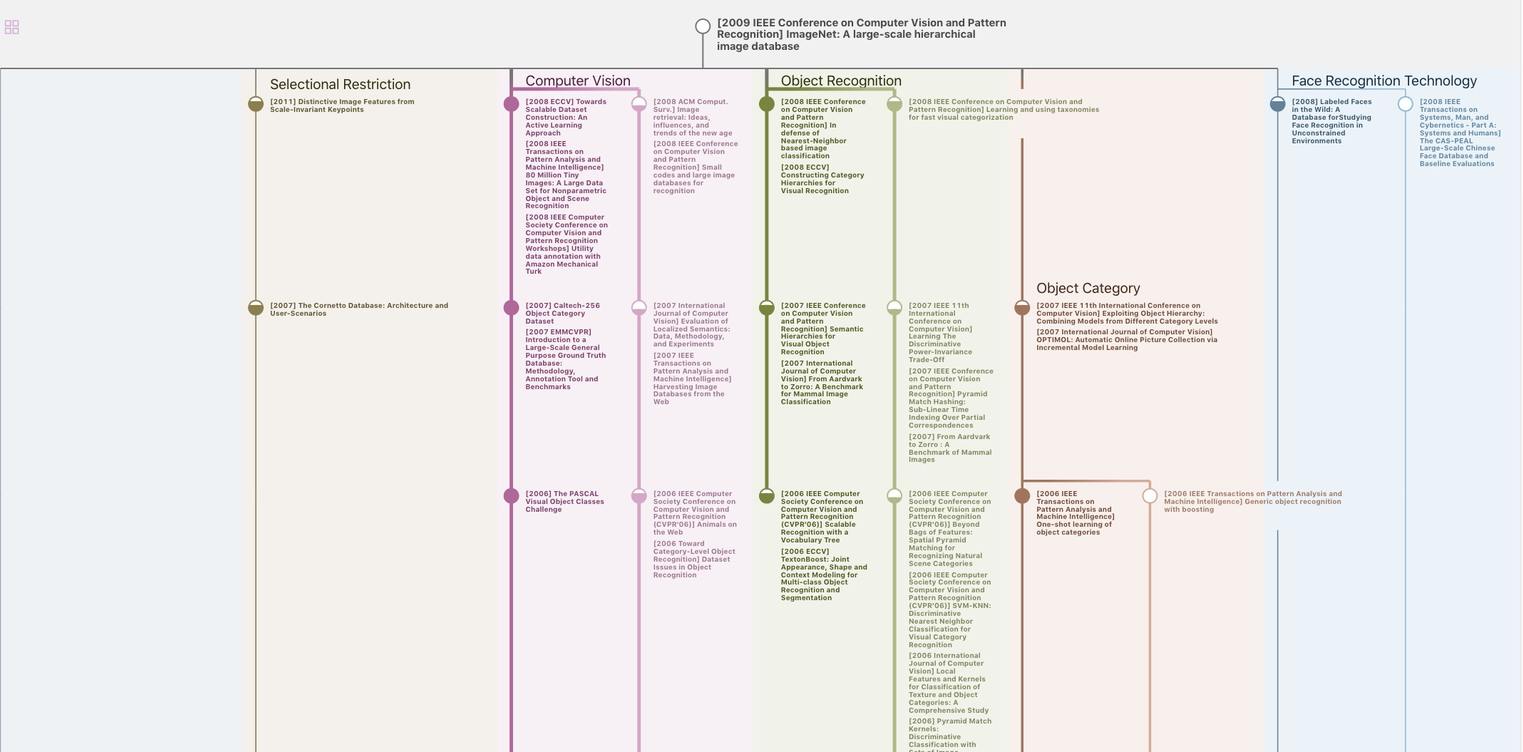
生成溯源树,研究论文发展脉络
Chat Paper
正在生成论文摘要