An Aggregated Baseline Load Estimation Method Based on Graph Convolutional Networks Introducing Graph Structure Learning
2024 IEEE/IAS 60th Industrial and Commercial Power Systems Technical Conference (I&CPS)(2024)
摘要
Accurate aggregated baseline load (ABL) estimation is crucial for demand response implementation. Traditional ABL estimation methods (e.g., averaging, regression, etc.) ignore the spatial correlation between different customer load patterns, thus leading to large errors in the estimation results. The Graph Convolutional Networks (GCN)-based ABL estimation method solves the above problems to a certain extent, however, the graphs it uses to capture the spatial correlations between different customer load patterns are completely human-defined with strong subjectivity of the constructor, so the mining of spatial correlations by this method is still not sufficiently objective and adequate, and the accuracy of the estimation results needs to be further improved. Based on this, this paper proposes an ABL estimation method based on Graph Structure Learning (GSL) and GCN. The method firstly defines the nodes and edges of the graph artificially, and then learns to obtain the adjacency matrix of the graph using the GSL algorithm. The graph obtained in this way is less subjective by the constructor than the one completely defined by man, and thus more fully exploits the spatial correlation between the data, which in turn improves the estimation accuracy of the method. Case studies on a real load dataset verify the effectiveness and superiority of the proposed method.
更多查看译文
关键词
Demand response,Aggregated baseline load,Graph structure learning,Graph convolutional network,Adjacency matrix,Spatial correlation
AI 理解论文
溯源树
样例
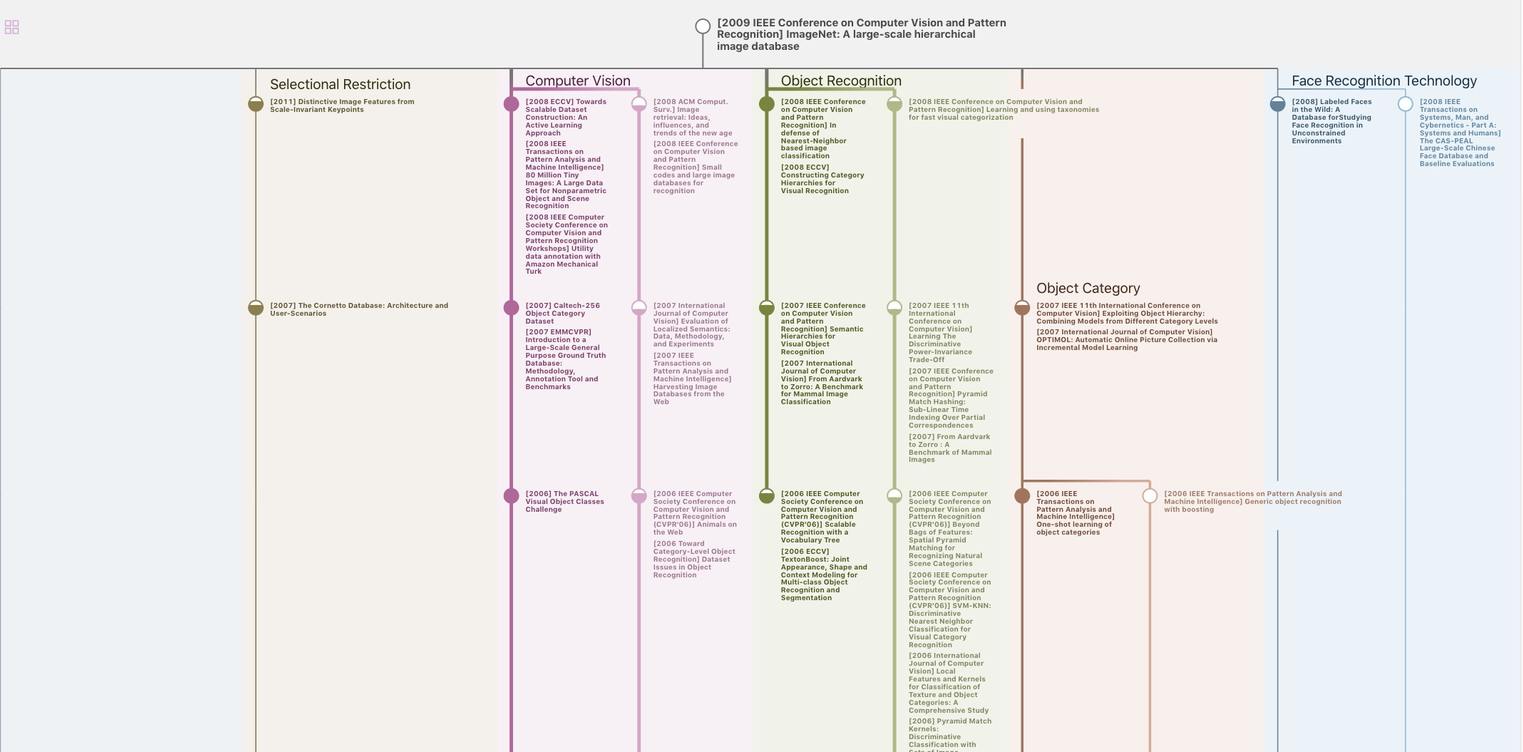
生成溯源树,研究论文发展脉络
Chat Paper
正在生成论文摘要