TM2B: Transformer-Based Motion-to-Box Network for 3D Single Object Tracking on Point Clouds
IEEE Robotics and Automation Letters(2024)
Abstract
3D single object tracking plays a crucial role in numerous applications such as autonomous driving. Recent trackers based on motion-centric paradigm perform well as they exploit motion cues to infer target relative motion across successive frames, which effectively overcome significant appearance variations of targets and distractors caused by occlusion. However, such a motion-centric paradigm tends to require multi-stage motion-to-box to refine the motion cues, which suffers from tedious hyper-parameter tuning and elaborate subtask designs. In this paper, we propose a novel transformer-based motion-to-box network (TM2B), which employs a learnable relation modeling transformer (LRMT) to generate accurate motion cues without multi-stage refinements. Our proposed LRMT contains two novel attention mechanisms: hierarchical interactive attention and learnable query attention. The former attention builds a learnable number-fixed sampling sets for each query on multi-scale feature maps, enabling each query to adaptively select prominent sampling elements, thus effectively encoding multi-scale features in a lightweight manner, while the latter calculates the weighted sum of the encoded features with learnable global query, enabling to extract valuable motion cues from all available features, thereby achieving accurate object tracking. Extensive experiments demonstrate that TM2B achieves state-of-the-art performance on KITTI, NuScenes and Waymo Open Dataset, while obtaining a significant improvement in inference speed over previous leading methods, achieving 56.8 FPS on a single NVIDIA 1080Ti GPU. The code is available at TM2B.
MoreTranslated text
Key words
3D single object tracking,Transformer,Motion-to-box
AI Read Science
Must-Reading Tree
Example
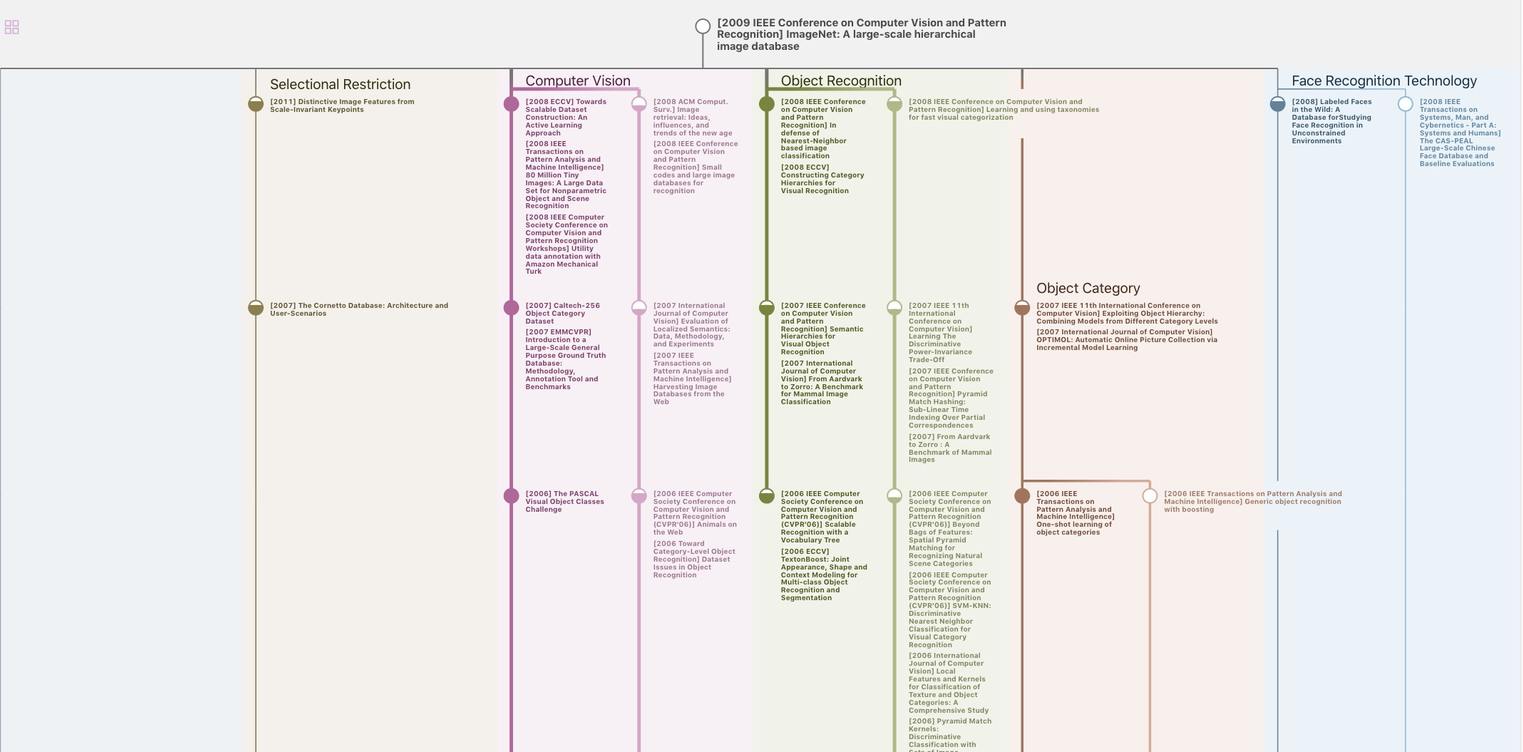
Generate MRT to find the research sequence of this paper
Chat Paper
Summary is being generated by the instructions you defined