Personalized Federated Learning Based on Feature Fusion
2024 27th International Conference on Computer Supported Cooperative Work in Design (CSCWD)(2024)
摘要
Federated learning enables distributed clients to collaborate on training while storing their data locally to protect client privacy. However, due to the heterogeneity of data, models, and devices, the final global model may need to perform better for tasks on each client. Communication bottlenecks, data heterogeneity, and model heterogeneity have been common challenges in federated learning. In this work, we considered a label distribution skew problem, a type of data heterogeneity easily overlooked. In the context of classification, we propose a personalized federated learning approach called pFedPM. In our process, we replace traditional gradient uploading with feature uploading, which helps reduce communication costs and allows for heterogeneous client models. These feature representations play a role in preserving privacy to some extent. We use a hyperparameter a to mix local and global features, which enables us to control the degree of personalization. We also introduced a relation network as an additional decision layer, which provides a non-linear learnable classifier to predict labels. Experimental results show that, with an appropriate setting of a, our scheme outperforms several recent FL methods on MNIST, FEMNIST, and CRIFAR10 datasets and achieves fewer communications.
更多查看译文
关键词
Federated learning,label distribution skew,features
AI 理解论文
溯源树
样例
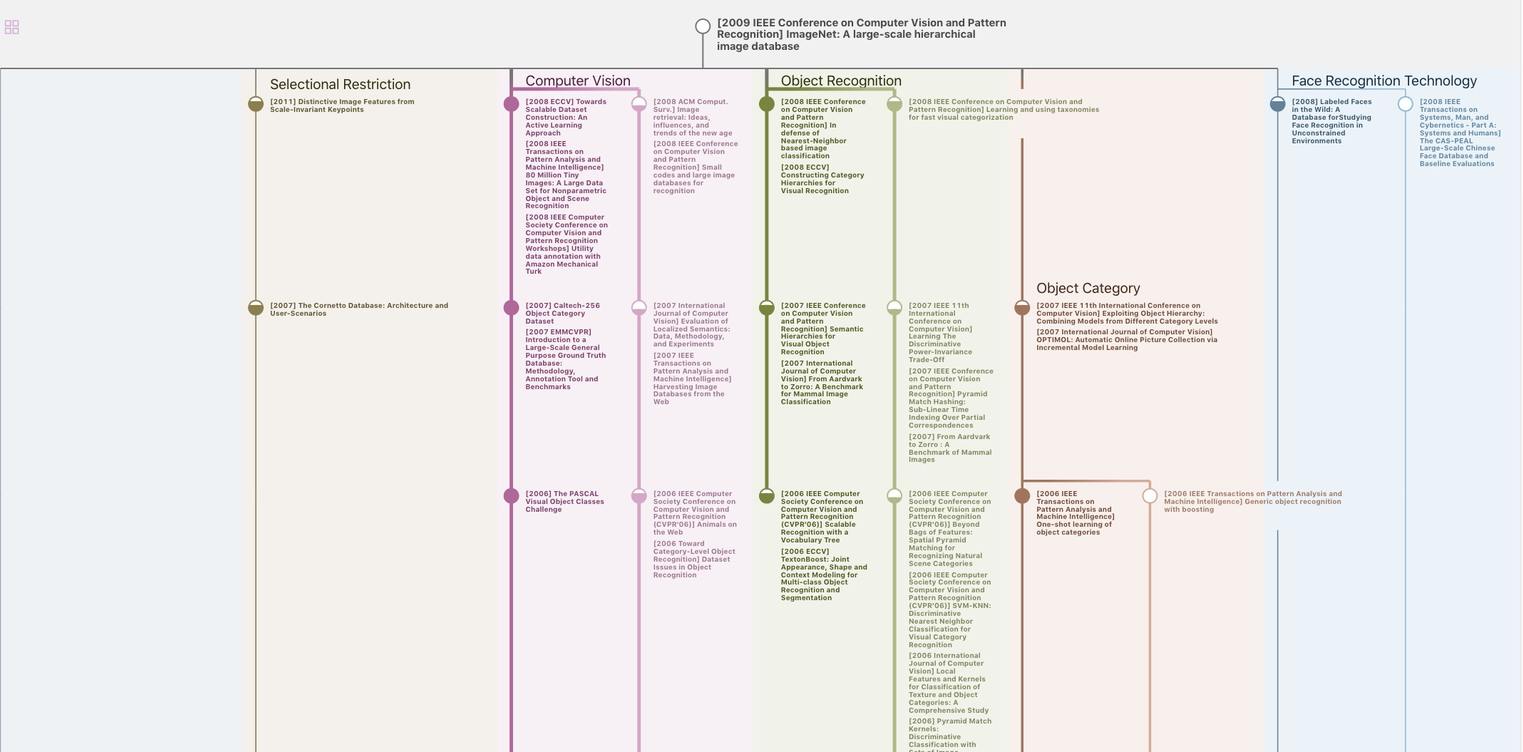
生成溯源树,研究论文发展脉络
Chat Paper
正在生成论文摘要