Multi-head Cascaded Swin Transformers with Attention to K-Space Sampling Pattern for Accelerated MRI Reconstruction
arXivorg(2022)
Abstract
Global correlations are widely seen in human anatomical structures due to similarity across tissues and bones. These correlations are reflected in magnetic resonance imaging (MRI) scans as a result of close-range proton density and T1/T2 parameters in tissues. Furthermore, to achieve accelerated MRI, k-space data are undersampled which causes global aliasing artifacts. Convolutional neural network (CNN) models are widely utilized for accelerated MRI reconstruction, but those models are limited in capturing global correlations due to the intrinsic locality of the convolution operation. The recent self-attention-based transformer models are capable of capturing global correlations among image features, however, the current contributions of transformer models for MRI reconstruction are minute. The existing contributions mostly provide CNN-transformer hybrid solutions and rarely leverage the physics of MRI. In this paper, we propose a physics-based stand-alone (convolution free) transformer model titled, the Multi-branch Cascaded Swin Transformers (McSTRA) for accelerated MRI reconstruction. McSTRA combines several interconnected MRI physics-related concepts with the transformer networks: it exploits global MR features via the shifted window self-attention mechanism; it extracts MR features belonging to different spectral components separately using a multi-branch setup; it iterates between intermediate de-aliasing and k-space correction via a cascaded network with data consistency in k-space and intermediate loss computations; furthermore, we propose a novel positional embedding generation mechanism to guide self-attention utilizing the point spread function corresponding to the undersampling mask. With the combination of all these components, we propose a consolidated robust solution for MRI reconstruction which shows improved performance by significantly outperforming state-of-the-art MRI reconstruction solutions both visually and quantitatively. Further experiments demonstrate the superior capabilities of McSTRA in combating adversarial conditions such as higher accelerations, noisy data, different undersampling protocols, out-of-distribution data
MoreTranslated text
AI Read Science
Must-Reading Tree
Example
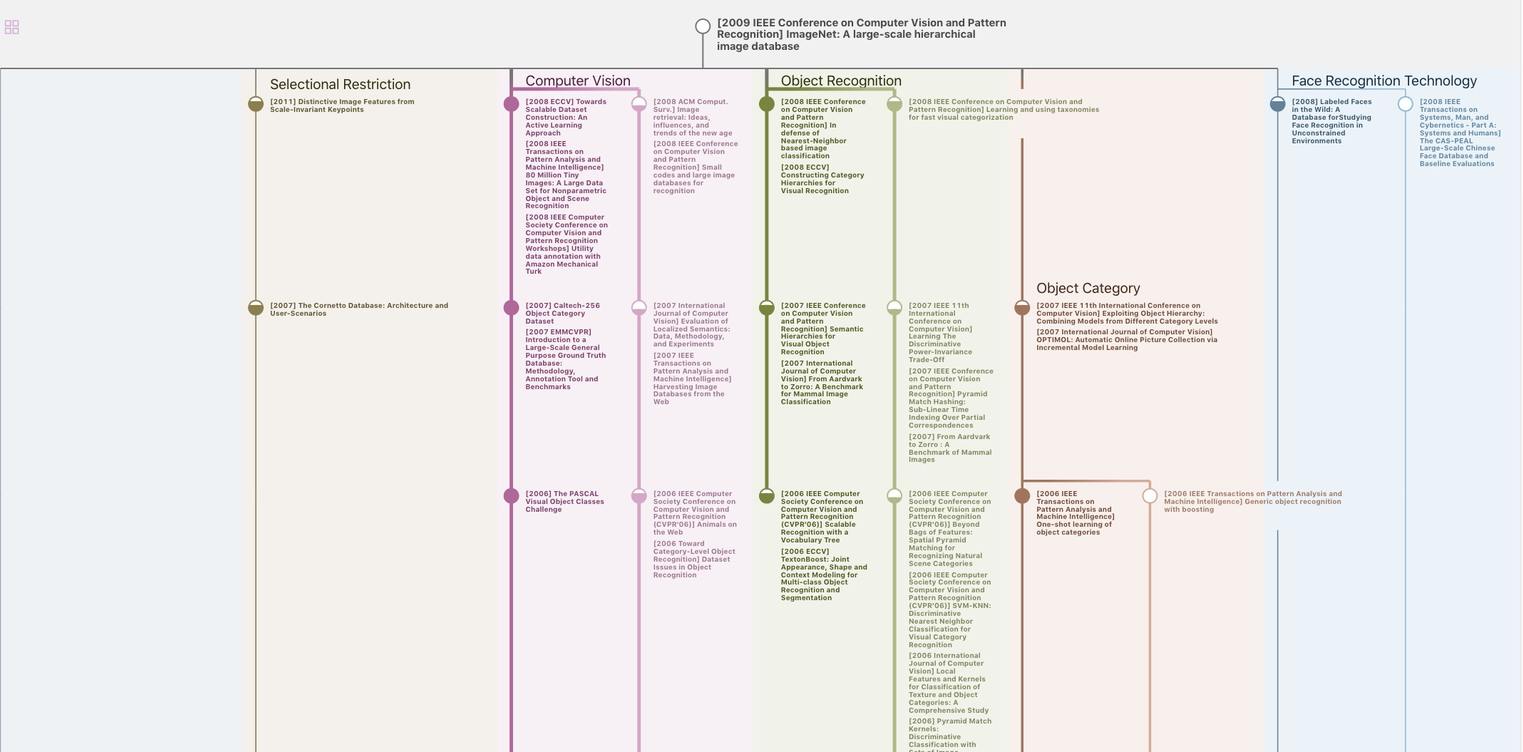
Generate MRT to find the research sequence of this paper
Chat Paper
Summary is being generated by the instructions you defined