Validation of Non-invasive Detection of Hyperkalemia by Artificial Intelligence Enhanced Electrocardiography in High Acuity Settings.
CLINICAL JOURNAL OF THE AMERICAN SOCIETY OF NEPHROLOGY(2024)
Abstract
BACKGROUND:Artificial intelligence (AI) electrocardiogram (ECG) analysis can enable detection of hyperkalemia. In this validation, we assessed the algorithm's performance in two high acuity settings.METHODS:An emergency department (ED) cohort (February-August 2021) and a mixed intensive care unit (ICU) cohort (August 2017-February 2018) were identified and analyzed separately. For each group, pairs of laboratory-collected potassium and 12 lead ECGs obtained within four hours of each other were identified. The previously developed AI ECG algorithm was subsequently applied to leads I and II of the 12 lead ECGs to screen for hyperkalemia (potassium > 6.0 mEq/L).RESULTS:The ED cohort (N=40,128) had a mean age of 60 years, 48% were male, and 1% (N=351) had hyperkalemia. The area under the curve (AUC) of the AI-ECG to detect hyperkalemia was 0.88, with sensitivity, specificity, positive predictive value, negative predictive value and positive likelihood ratio of, 80%, 80%, 3%, 99.8% and 4.0, respectively, in the ED cohort. Low-eGFR (<30 ml/min) subanalysis yielded AUC, sensitivity, specificity, positive predictive value, negative predictive value and positive likelihood ratio of 0.83, 86%, 60%, 15%, 98% and 2.2, respectively, in the ED cohort. The ICU cohort (N=2,636) had a mean age of 65 years, 60% were male, and 3% (N=87) had hyperkalemia. The AUC for the AI-ECG was 0.88 and yielded sensitivity, specificity, positive predictive value, negative predictive value and positive likelihood ratio of 82%, 82%, 14%, 99% and 4.6, respectively in the ICU cohort. Low-eGFR subanalysis yielded AUC, sensitivity, specificity, positive predictive value, negative predictive value and positive likelihood ratio of 0.85, 88%, 67%, 29%, 97% and 2.7, respectively in the ICU cohort.CONCLUSION:The AI-ECG algorithm demonstrated a high negative predictive value, suggesting that it is useful for ruling out hyperkalemia, but a low positive predictive value, suggesting that it is insufficient for treating hyperkalemia.
MoreTranslated text
Key words
clinical nephrology,electrolytes,fluid, electrolyte, and acid-base disorders,patient-centered care
AI Read Science
Must-Reading Tree
Example
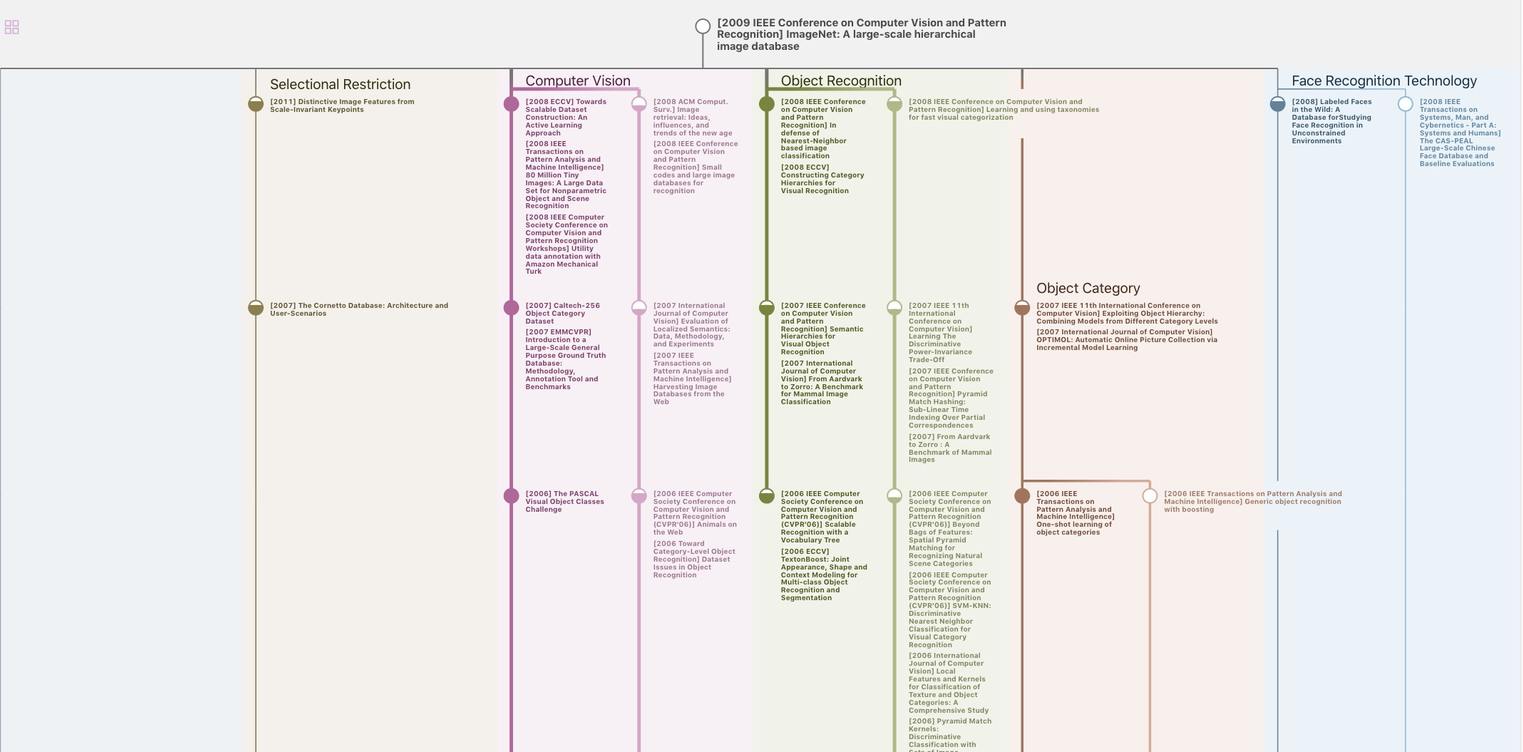
Generate MRT to find the research sequence of this paper
Chat Paper
Summary is being generated by the instructions you defined