UniHead: Unifying Multi-Perception for Detection Heads
IEEE TRANSACTIONS ON NEURAL NETWORKS AND LEARNING SYSTEMS(2024)
摘要
The detection head constitutes a pivotal component within object detectors, tasked with executing both classification and localization functions. Regrettably, the commonly used parallel head often lacks omni perceptual capabilities, such as deformation perception (DP), global perception (GP), and cross-task perception (CTP). Despite numerous methods attempting to enhance these abilities from a single aspect, achieving a comprehensive and unified solution remains a significant challenge. In response to this challenge, we develop an innovative detection head, termed UniHead, to unify three perceptual abilities simultaneously. More precisely, our approach: 1) introduces DP, enabling the model to adaptively sample object features; 2) proposes a dual-axial aggregation transformer (DAT) to adeptly model long-range dependencies, thereby achieving GP; and 3) devises a cross-task interaction transformer (CIT) that facilitates interaction between the classification and localization branches, thus aligning the two tasks. As a plug-and-play method, the proposed UniHead can be conveniently integrated with existing detectors. Extensive experiments on the COCO dataset demonstrate that our UniHead can bring significant improvements to many detectors. For instance, the UniHead can obtain + 2.7 AP gains in RetinaNet, + 2.9 AP gains in FreeAnchor, and + 2.1 AP gains in GFL. The code is available at https://github.com/zht8506/UniHead.
更多查看译文
关键词
Detection head,object detection,transformer,unifying multi-perception
AI 理解论文
溯源树
样例
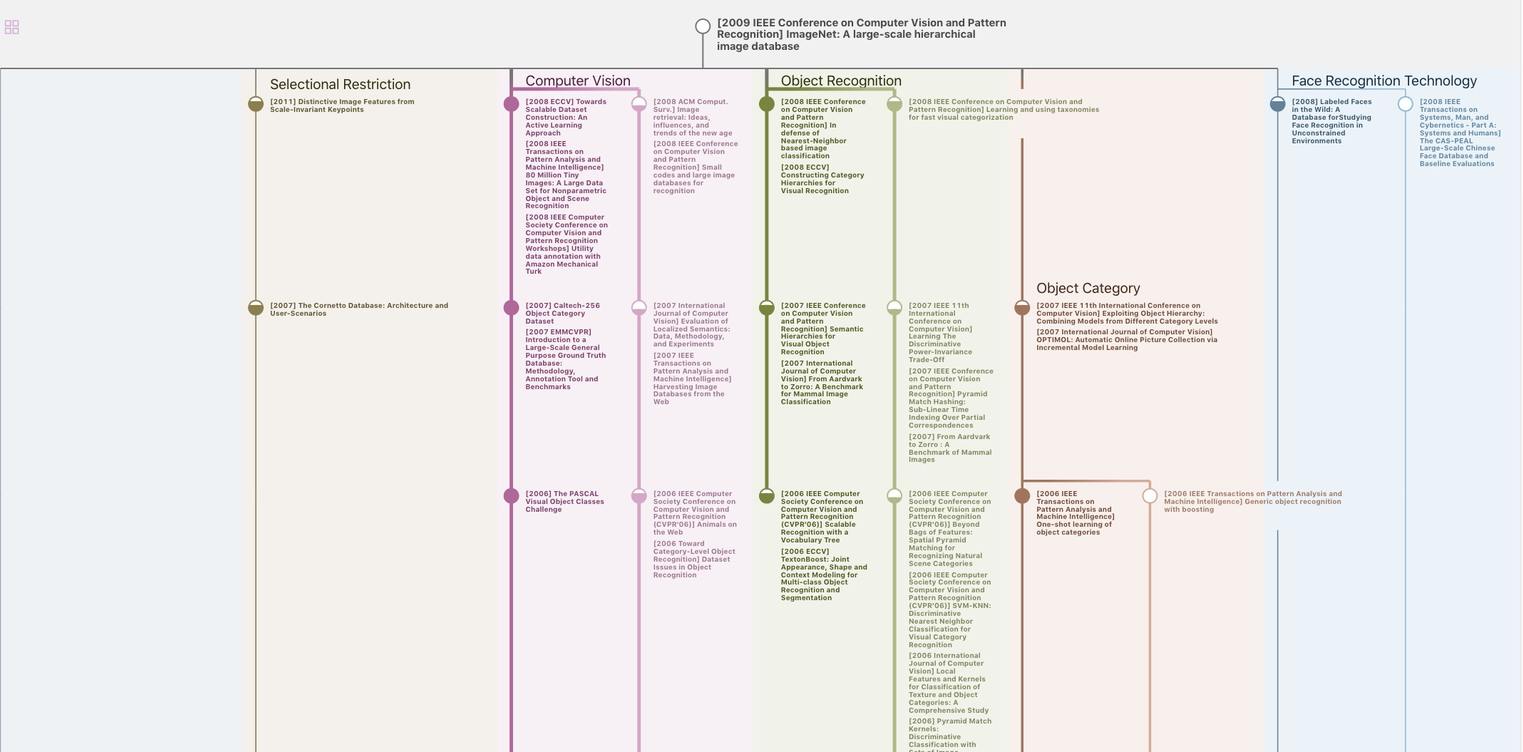
生成溯源树,研究论文发展脉络
Chat Paper
正在生成论文摘要