Robust Resource Bounds with Static Analysis and Bayesian Inference
Proceedings of the ACM on programming languages(2024)
Abstract
There are two approaches to automatically deriving symbolic worst-case resource bounds for programs: static analysis of the source code and data-driven analysis of cost measurements obtained by running the program. Static resource analysis is usually sound but incomplete. Data-driven analysis can always return a result, but its lack of robustness often leads to unsound results. This paper presents the design, implementation, and empirical evaluation of hybrid resource bound analyses that tightly integrate static analysis and data-driven analysis. The static analysis part builds on automatic amortized resource analysis (AARA), a state-of-the-art type-based resource analysis method that performs cost bound inference using linear optimization. The data-driven part is rooted in novel Bayesian modeling and inference techniques that improve upon previous data-driven analysis methods by reporting an entire probability distribution over likely resource cost bounds. A key innovation is a new type inference system called Hybrid AARA that coherently integrates Bayesian inference into conventional AARA, combining the strengths of both approaches. Hybrid AARA is proven to be statistically sound under standard assumptions on the runtime cost data. An experimental evaluation on a challenging set of benchmarks shows that Hybrid AARA (i) effectively mitigates the incompleteness of purely static resource analysis; and (ii) is more accurate and robust than purely data-driven resource analysis.
MoreTranslated text
Key words
Bayesian inference,data-driven analysis,hybrid analysis,resource analysis,static analysis,worst-case costs
AI Read Science
Must-Reading Tree
Example
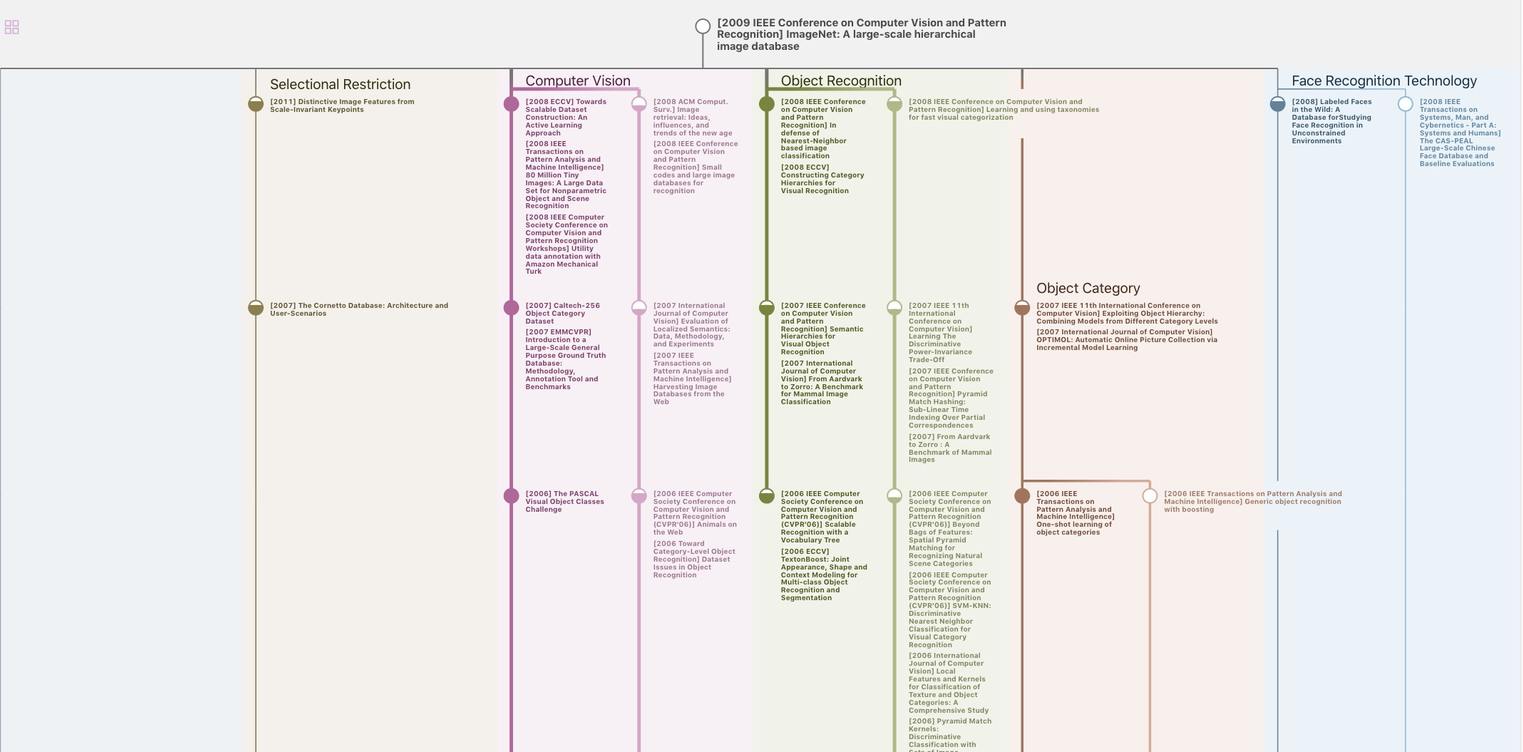
Generate MRT to find the research sequence of this paper
Chat Paper
Summary is being generated by the instructions you defined