High-Tc superconductor candidates proposed by machine learning
arxiv(2024)
摘要
We cast the relation between chemical compositions of solid-state materials
and their superconducting critical temperature (Tc) in terms of a statistical
learning problem with reduced complexity. Training of query-aware
similarity-based ridge regression models on experimental SuperCon data with
(implicit) and without (ambient) high pressure entries achieves average Tc
prediction errors of 10 K for unseen out-of-sample materials. Subsequent
utilization of the approach to scan 153k materials in the Materials Project
enables the ranking of candidates by Tc while taking into account thermodynamic
stability and small band gap. Stable top three high-Tc candidate materials with
large band gaps for implicit and ambient pressures are predicted to be
Cs2Sn(H2N)6 (324 K), CsH5N2 (315K), Rb2Sn(H2N)6 (305 K), and H15IrBr3N5 (189
K), H12OsN5Cl3O (161 K), B10H13I (151 K), respectively. Stable top three
high-Tc candidate materials with small band gaps for implicit and ambient
pressures are predicted to be RbLiH12Se3N4 (255 K), CeH14Cl3O7 (246 K),
Li(H3N)4 (234 K), and ReH30Ru2(NCl)10 (127 K), AlH18Ru(NF)6 (120 K), Sr(Li2P)2
(117 K), respectively.
更多查看译文
AI 理解论文
溯源树
样例
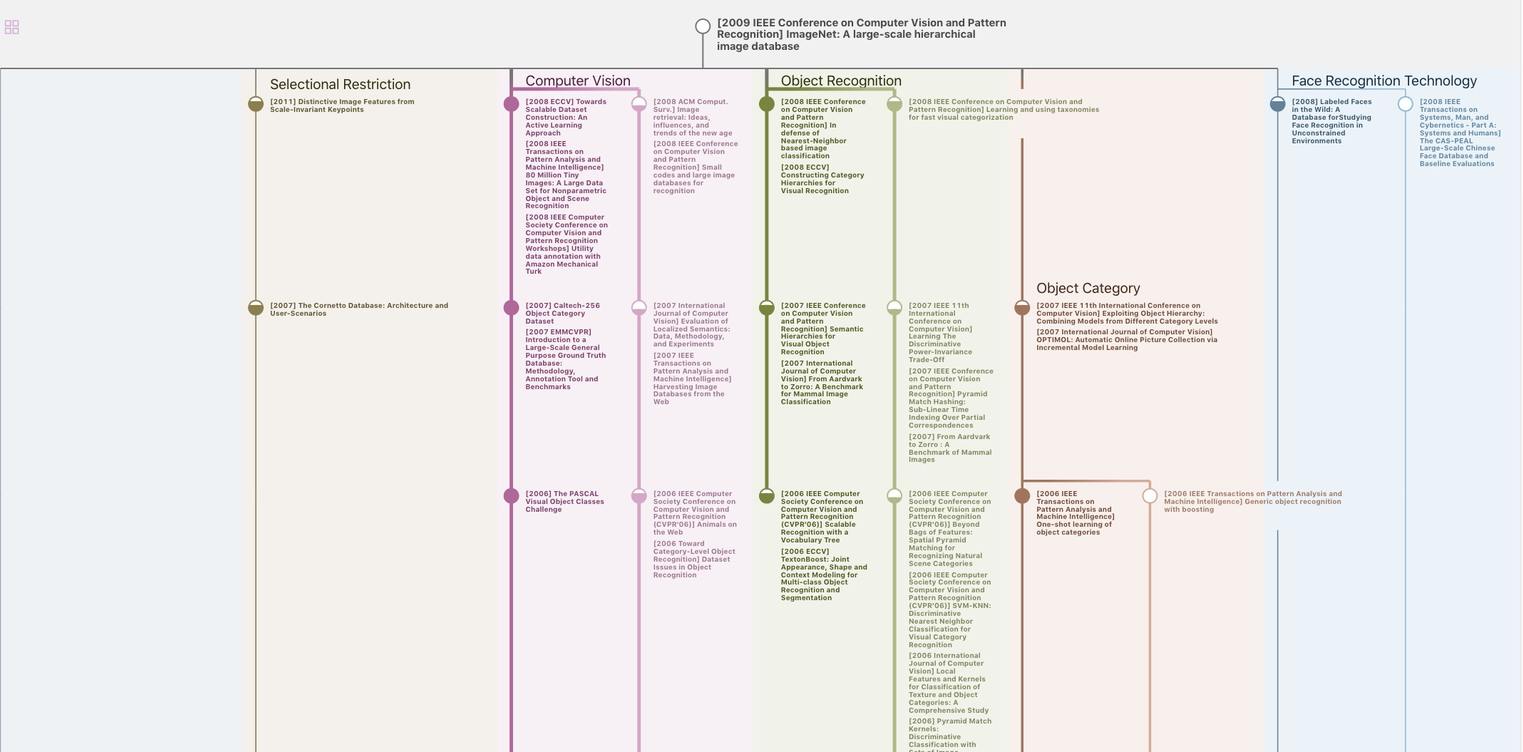
生成溯源树,研究论文发展脉络
Chat Paper
正在生成论文摘要