FLoCoRA: Federated learning compression with low-rank adaptation
CoRR(2024)
摘要
Low-Rank Adaptation (LoRA) methods have gained popularity in efficient
parameter fine-tuning of models containing hundreds of billions of parameters.
In this work, instead, we demonstrate the application of LoRA methods to train
small-vision models in Federated Learning (FL) from scratch. We first propose
an aggregation-agnostic method to integrate LoRA within FL, named FLoCoRA,
showing that the method is capable of reducing communication costs by 4.8
times, while having less than 1
classification task with a ResNet-8. Next, we show that the same method can be
extended with an affine quantization scheme, dividing the communication cost by
18.6 times, while comparing it with the standard method, with still less than
1
represents a strong baseline for message size reduction, even when compared to
conventional model compression works, while also reducing the training memory
requirements due to the low-rank adaptation.
更多查看译文
AI 理解论文
溯源树
样例
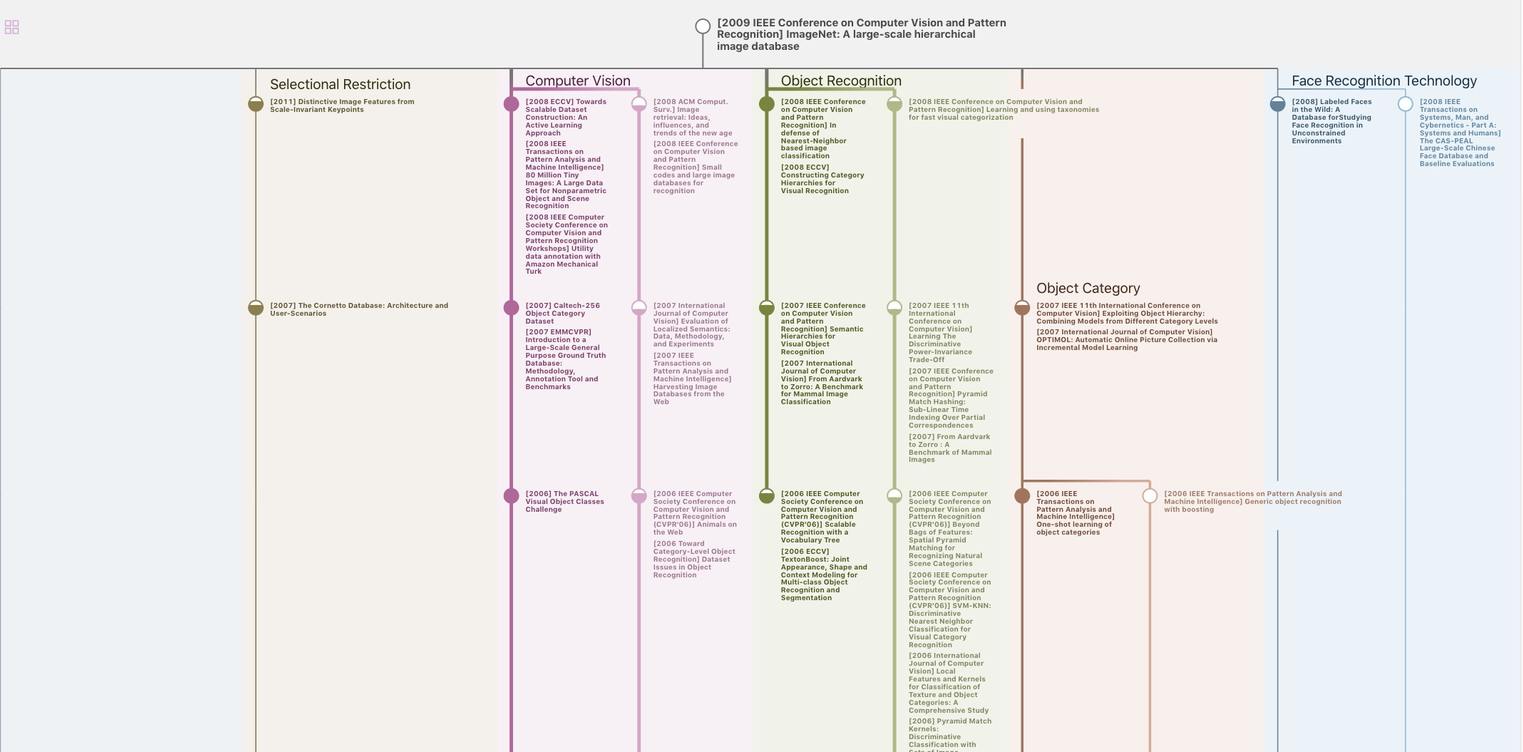
生成溯源树,研究论文发展脉络
Chat Paper
正在生成论文摘要