Global Human-guided Counterfactual Explanations for Molecular Properties Via Reinforcement Learning
KDD 2024(2024)
摘要
Counterfactual explanations of Graph Neural Networks (GNNs) offer a powerful way to understand data that can naturally be represented by a graph structure. Furthermore, in many domains, it is highly desirable to derive data-driven global explanations or rules that can better explain the high-level properties of the models and data in question. However, evaluating global counterfactual explanations is hard in real-world datasets due to a lack of human-annotated ground truth, which limits their use in areas like molecular sciences. Additionally, the increasing scale of these datasets provides a challenge for random search-based methods. In this paper, we develop a novel global explanation model RLHEX for molecular property prediction. It aligns the counterfactual explanations with human-defined principles, making the explanations more interpretable and easy for experts to evaluate. RLHEX includes a VAE-based graph generator to generate global explanations and an adapter to adjust the latent representation space to human-defined principles. Optimized by Proximal Policy Optimization (PPO), the global explanations produced by RLHEX cover 4.12% more input graphs and reduce the distance between the counterfactual explanation set and the input set by 0.47% on average across three molecular datasets. RLHEX provides a flexible framework to incorporate different human-designed principles into the counterfactual explanation generation process, aligning these explanations with domain expertise. The code and data are released at https://github.com/dqwang122/RLHEX.
更多查看译文
关键词
Graph Neural Networks,Counterfactual Explanations,Reinforcement Learning,Global Explanations,Molecular Properties
AI 理解论文
溯源树
样例
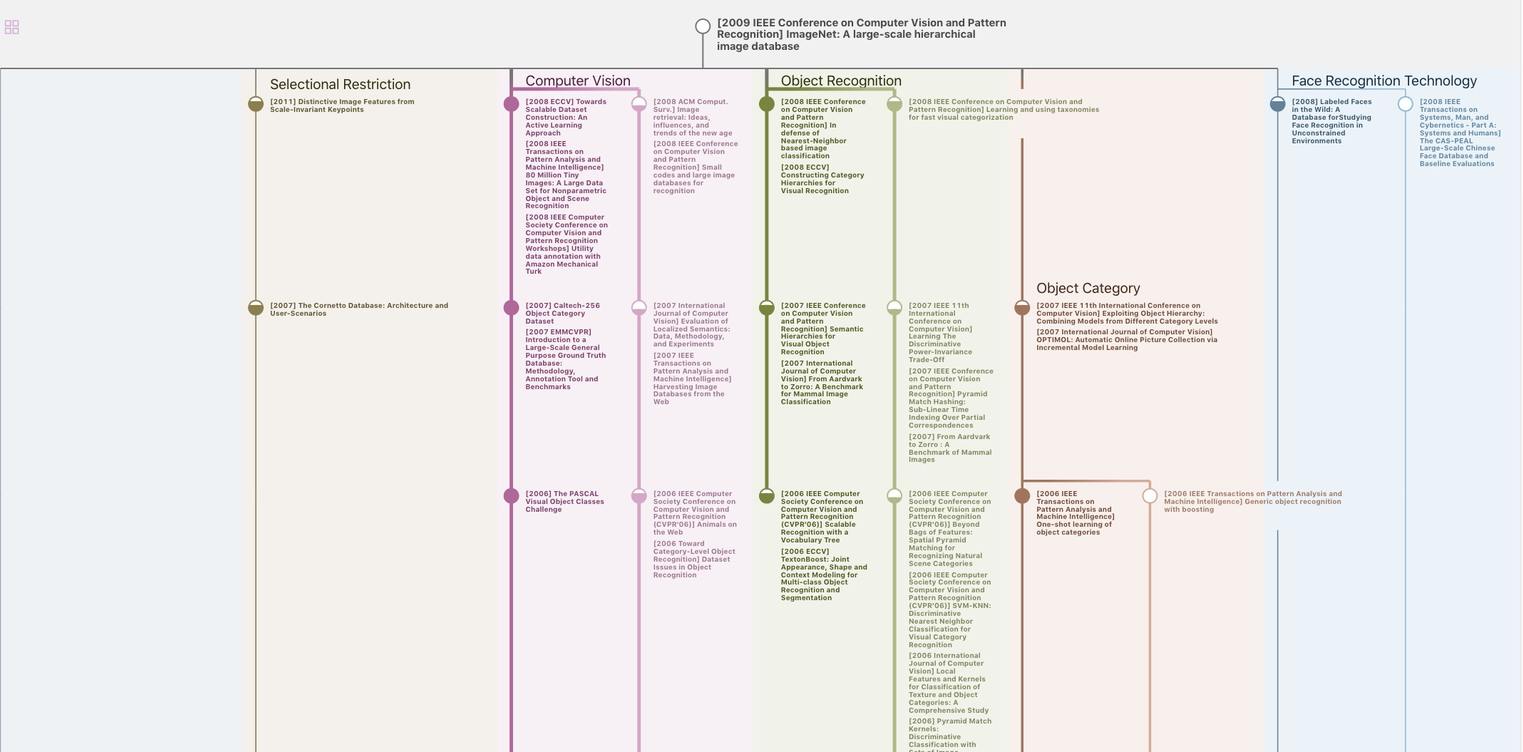
生成溯源树,研究论文发展脉络
Chat Paper
正在生成论文摘要