Dynamic-Obstacle Relative Localization Using Motion Segmentation with Event Cameras
2024 International Conference on Unmanned Aircraft Systems (ICUAS)(2024)
摘要
The ability to detect and localize dynamic obstacles within a robot's surroundings while navigating low-light environments is crucial for ensuring robot safety and the continuity of its mission. Event cameras excel in capturing motion within scenes clearly without motion blur, due to their asynchronous nature. These sensors are distinguished by their ability to trigger events with microsecond temporal resolution, possess a high dynamic range, and achieve low latency. In this work, we introduce a framework for a drone equipped with an event camera, named E-DoRL. This framework is specifically designed to address the challenge of detecting and localizing dynamic obstacles that are not previously known, ensuring safe navigation. E-DoRL processes raw event streams to estimate the relative position between a moving robot and dynamic obstacles. It employs a Graph Transformer Neural Network (GTNN) to extract spatiotemporal correlations from event streams, identifying active event pixels of moving objects without prior knowledge of scene topology or camera motion. Based on these identifications, E-DoRL is designed to determine the relative position of moving obstacles with respect to a dynamic unmanned aerial vehicle (UAV). E-DoRL outperformed state-of-the-art frame-based object tracking algorithms in good light scenarios (100 lux), by achieving 59.7% and 25.9% reduction in the mean absolute error (MAE) associated with the X and Y estimates, respectively. Additionally, when tested under much lower light illuminance (0.8 lux), E-DoRL consistently maintained its performance without any degradation, as opposed to image-based techniques that are highly sensitive to lighting conditions.
更多查看译文
关键词
Dynamic Vision Sensor,Neural Network,Spatiotemporal,Transformer,Light Conditions,Mean Absolute Error,Low Light,Unmanned Aerial Vehicles,Tracking Algorithm,Object Tracking,High Dynamic Range,Motion Blur,Good Light,Camera Motion,Dynamic Obstacles,Event Stream,Deep Learning,Dynamic Environment,Transformation Matrix,Model Discrimination,Inertial Frame,Learning-based Algorithms,Low Light Conditions,Robot Navigation,Onboard Sensors,Image-based Methods,Pinhole Camera Model,Optical Flow,Camera Frame,2D Plane
AI 理解论文
溯源树
样例
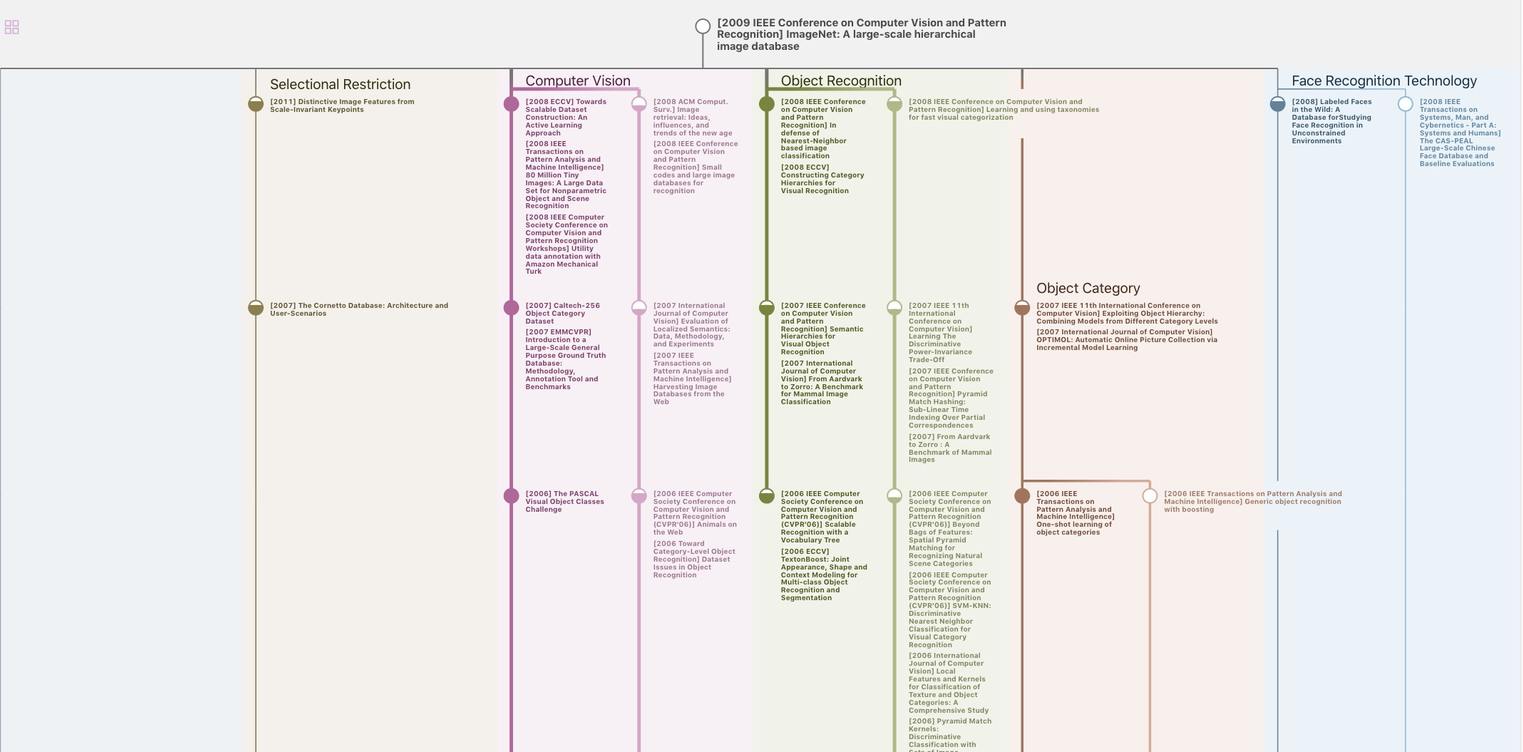
生成溯源树,研究论文发展脉络
Chat Paper
正在生成论文摘要