Functional Effects of Mutations in Proteins Can Be Predicted and Interpreted by Guided Selection of Sequence Covariation Information.
Proceedings of the National Academy of Sciences of the United States of America(2024)
摘要
Predicting the effects of one or more mutations to the in vivo or in vitro properties of a wild-type protein is a major computational challenge, due to the presence of epistasis, that is, of interactions between amino acids in the sequence. We introduce a computationally efficient procedure to build minimal epistatic models to predict mutational effects by combining evolutionary (homologous sequence) and few mutational-scan data. Mutagenesis measurements guide the selection of links in a sparse graphical model, while the parameters on the nodes and the edges are inferred from sequence data. We show, on 10 mutational scans, that our pipeline exhibits performances comparable to state-of-the-art deep networks trained on many more data, while requiring much less parameters and being hence more interpretable. In particular, the identified interactions adapt to the wild-type protein and to the fitness or biochemical property experimentally measured, mostly focus on key functional sites, and are not necessarily related to structural contacts. Therefore, our method is able to extract information relevant for one mutational experiment from homologous sequence data reflecting the multitude of structural and functional constraints acting on proteins throughout evolution.
更多查看译文
关键词
inference of sparse graphical Potts models,fitness prediction,multiple sequence alignments,mutational scans,functional networks
AI 理解论文
溯源树
样例
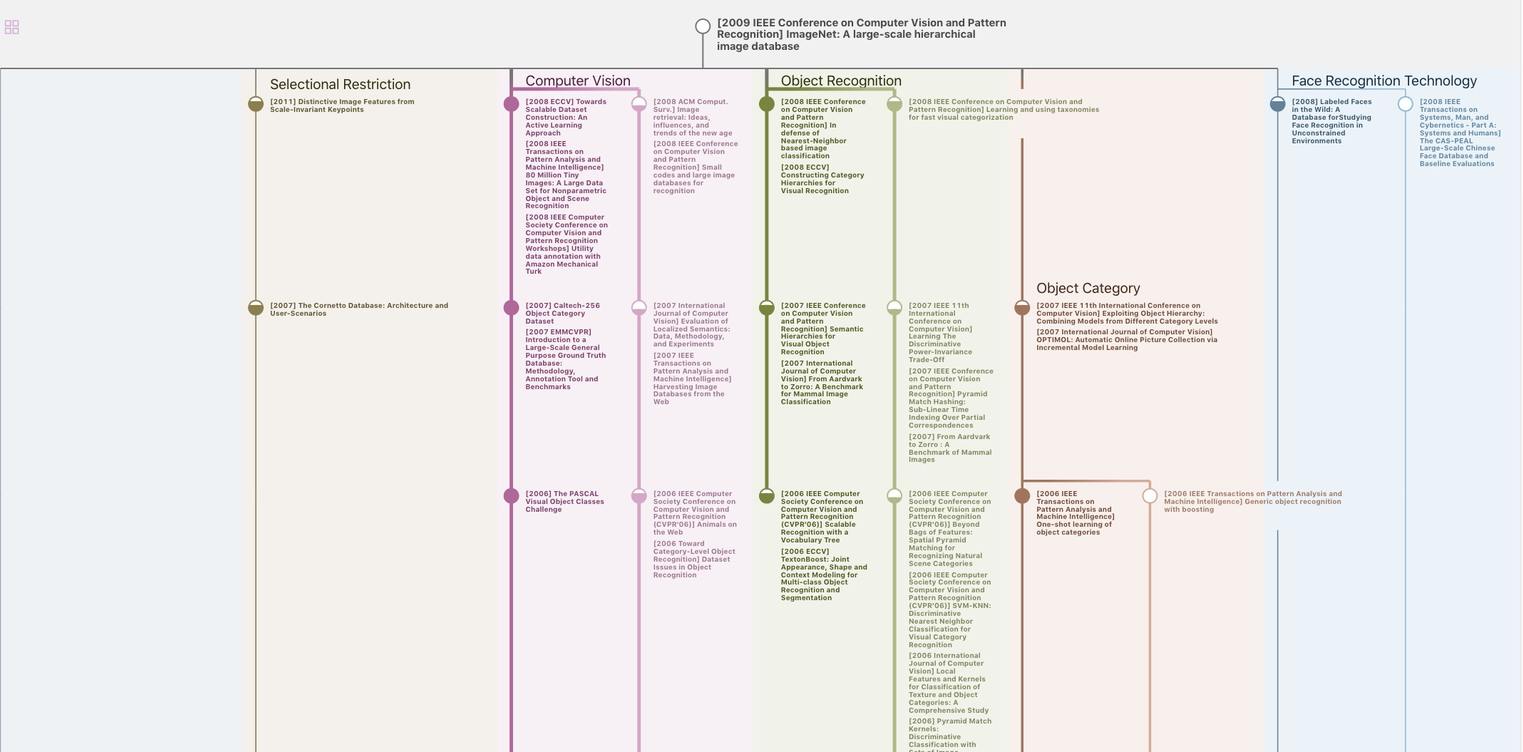
生成溯源树,研究论文发展脉络
Chat Paper
正在生成论文摘要