Inverse Value Iteration and $Q$ -Learning: Algorithms, Stability, and Robustness
IEEE Transactions on Neural Networks and Learning Systems(2024)
摘要
This article proposes a data-driven model-free inverse $Q$ -learning algorithm for continuous-time linear quadratic regulators (LQRs). Using an agent’s trajectories of states and optimal control inputs, the algorithm reconstructs its cost function that captures the same trajectories. This article first poses a model-based inverse value iteration scheme using the agent’s system dynamics. Then, an online model-free inverse $Q$ -learning algorithm is developed to recover the agent’s cost function only using the demonstrated trajectories. It is more efficient than the existing inverse reinforcement learning (RL) algorithms as it avoids the repetitive RL in inner loops. The proposed algorithms do not need initial stabilizing control policies and solve for unbiased solutions. The proposed algorithm’s asymptotic stability, convergence, and robustness are guaranteed. Theoretical analysis and simulation examples show the effectiveness and advantages of the proposed algorithms.
更多查看译文
关键词
Heuristic algorithms,Trajectory,Optimal control,Cost function,Q-learning,Mathematical models,Robustness,Convergence,inverse optimal control (IOC),inverse reinforcement learning (RL),model-free,robustness,stability
AI 理解论文
溯源树
样例
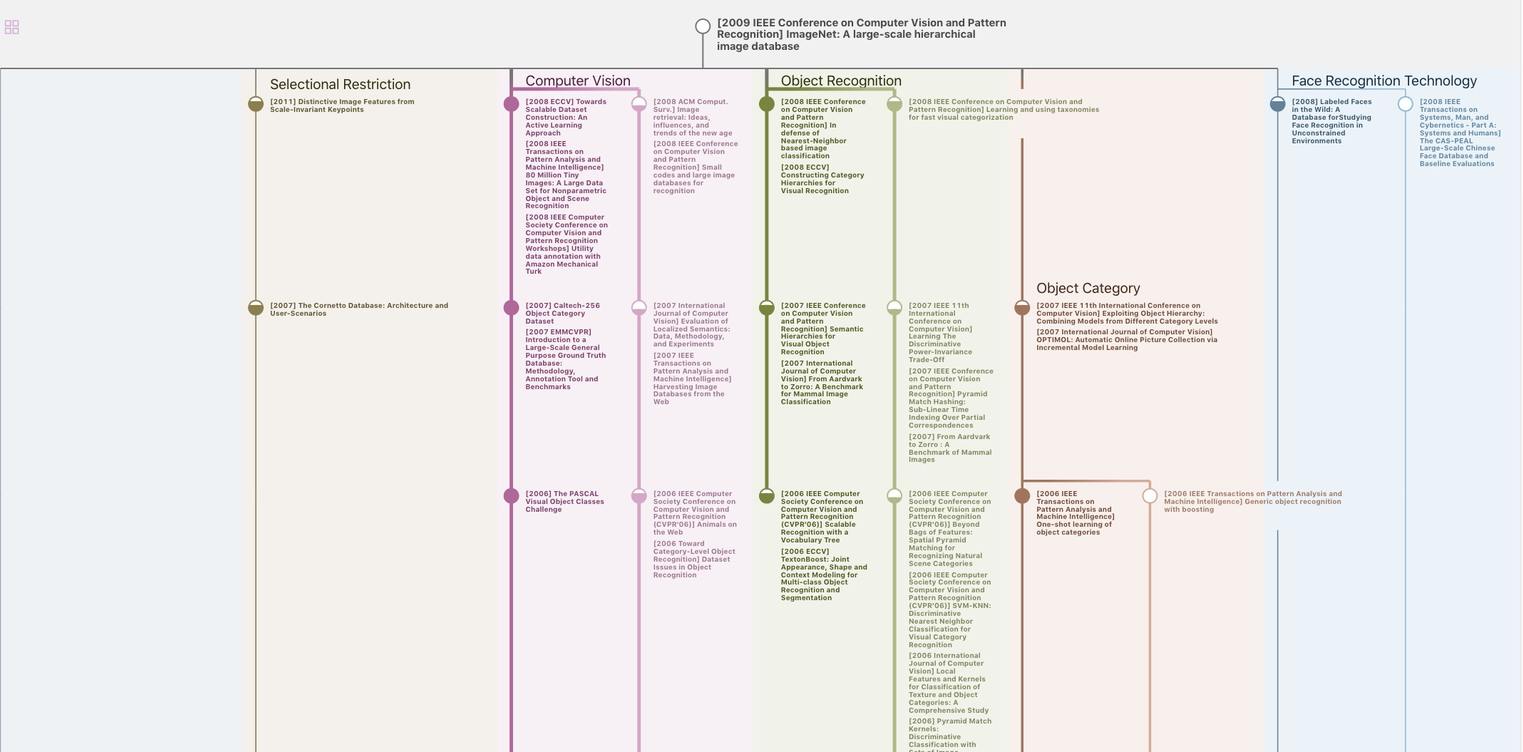
生成溯源树,研究论文发展脉络
Chat Paper
正在生成论文摘要