Contrastive Pre-training with Multi-level Alignment for Grounded Multimodal Named Entity Recognition.
International Conference on Multimedia Retrieval(2024)
摘要
Recently, Grounded Multimodal Named Entity Recognition (GMNER) task has been introduced to refine the Multimodal Named Entity Recognition (MNER) task.Existing MNER studies fall short in that they merely focus on extracting text-based entity-type pairs, often leading to entity ambiguities and failing to contribute to multimodal knowledge graph construction.In the GMNER task, the objective becomes more challenging: identifying named entities in text, determining their entity types, and locating their corresponding bounding boxes in linked images, necessitating precise alignment between the textual and visual information.We introduce a novel multi-level alignment pre-training method, engaging with both text-image and entity-object dimensions to foster deeper congruence between multimodal data.Specifically, we innovatively harness potential objects identified within images, aligning them with textual entity prompts, thereby generating refined soft pseudo-labels.These labels serve as self-supervised signals that pre-train the model to more accurately extract entities from textual input.To address misalignments that often plague modality integration, our method employs a sophisticated diffusion model that performs back-translation on the text to generate a corresponding visual representation, thus refining the model's multimodal interpretative accuracy.Empirical evidence from the GMNER dataset validates that our approach significantly outperforms existing state-of-the-art models.Moreover, the versatility of our pre-training process complements virtually all extant models, offering an additional avenue for augmenting their multimodal entity recognition acumen.
更多查看译文
AI 理解论文
溯源树
样例
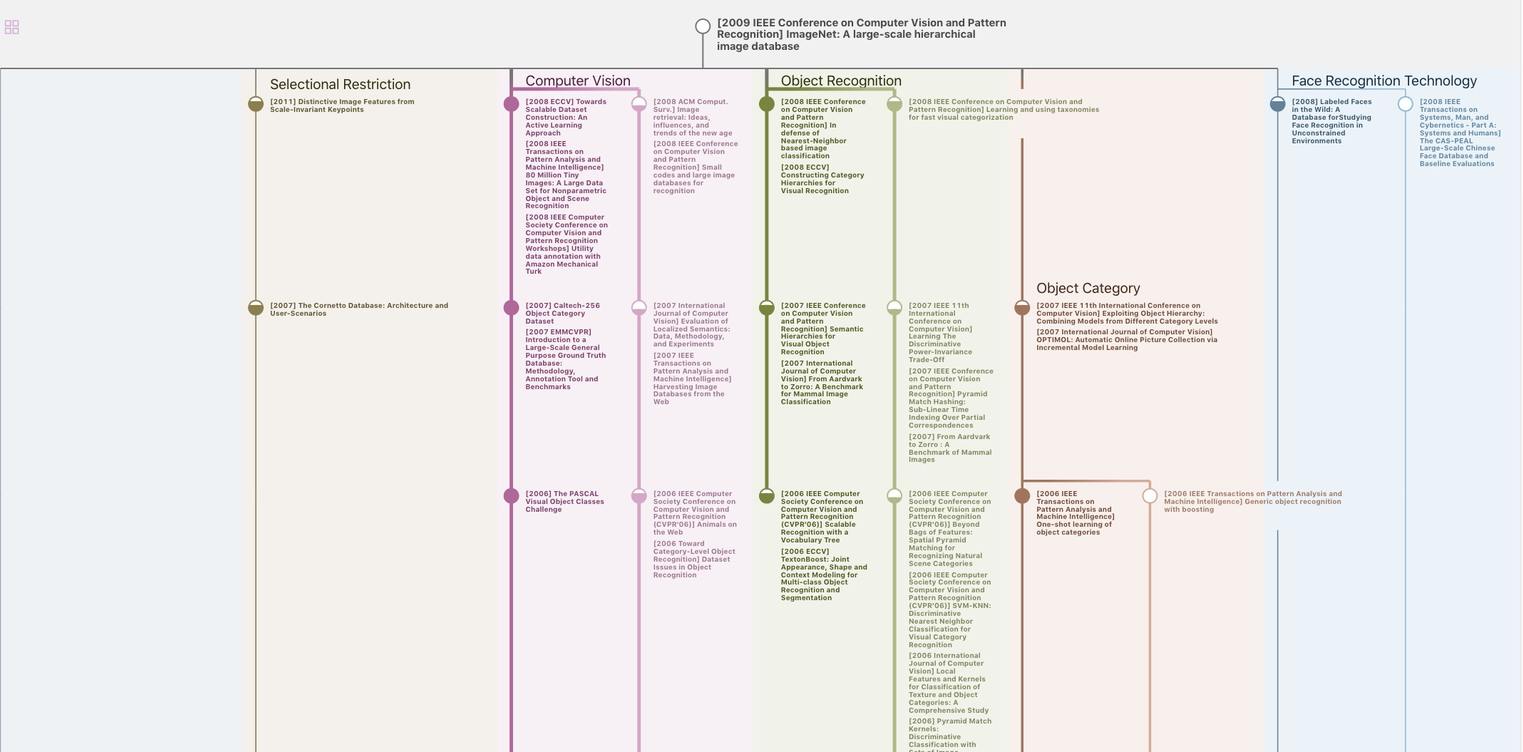
生成溯源树,研究论文发展脉络
Chat Paper
正在生成论文摘要