Increasing certainty in systems biology models using Bayesian multimodel inference
arxiv(2024)
摘要
Mathematical models are indispensable to the system biology toolkit for
studying the structure and behavior of intracellular signaling networks. A
common approach to modeling is to develop a system of equations that encode the
known biology using approximations and simplifying assumptions. As a result,
the same signaling pathway can be represented by multiple models, each with its
set of underlying assumptions, which opens up challenges for model selection
and decreases certainty in model predictions. Here, we use Bayesian multimodel
inference to develop a framework to increase certainty in systems biology
models. Using models of the extracellular regulated kinase (ERK) pathway, we
first show that multimodel inference increases predictive certainty and yields
predictors that are robust to changes in the set of available models. We then
show that predictions made with multimodel inference are robust to data
uncertainties introduced by decreasing the measurement duration and reducing
the sample size. Finally, we use multimodel inference to identify a new model
to explain experimentally measured sub-cellular location-specific ERK activity
dynamics. In summary, our framework highlights multimodel inference as a
disciplined approach to increasing the certainty of intracellular signaling
activity predictions.
更多查看译文
AI 理解论文
溯源树
样例
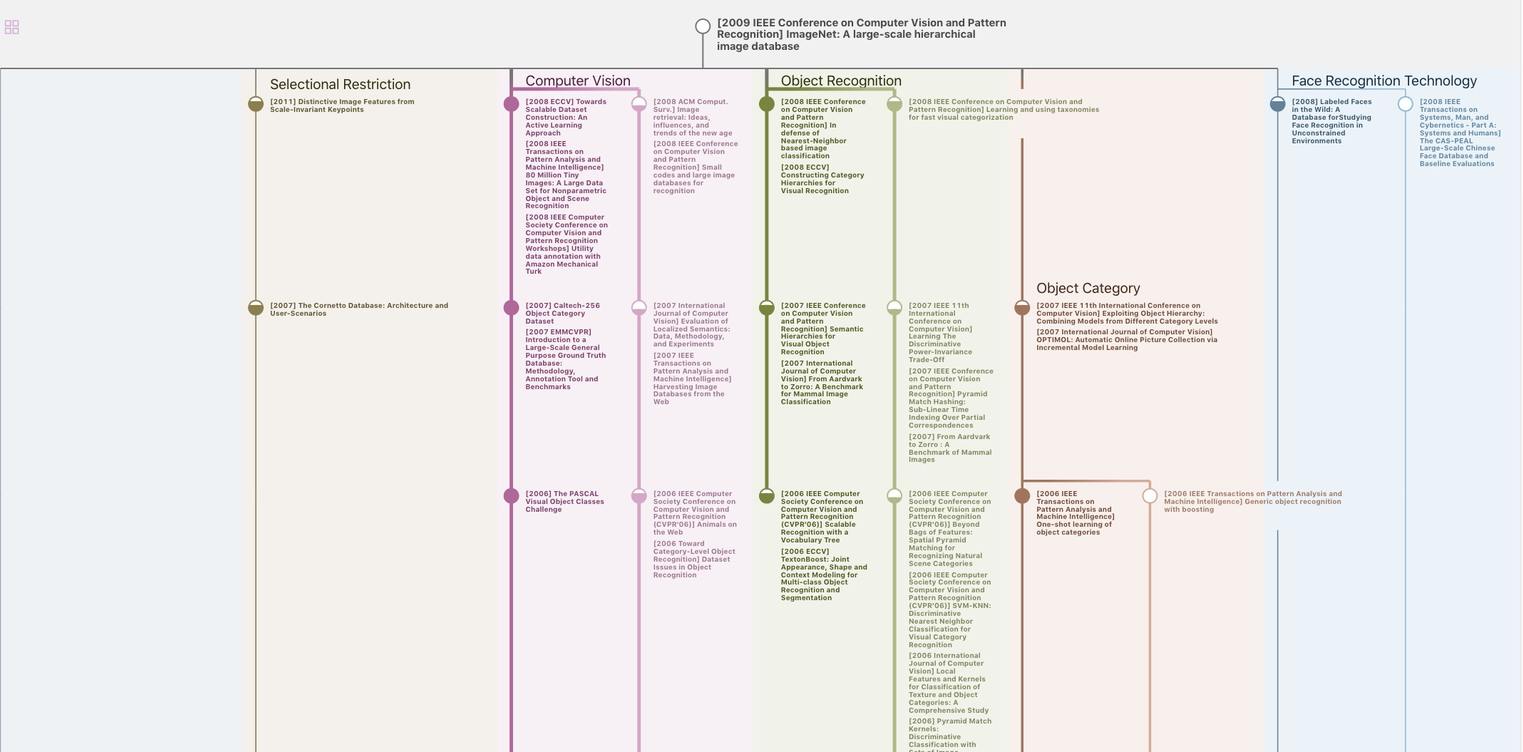
生成溯源树,研究论文发展脉络
Chat Paper
正在生成论文摘要