Concept-skill Transferability-based Data Selection for Large Vision-Language Models
CoRR(2024)
Abstract
Instruction tuning, or supervised finetuning on extensive task-specific data,
is necessary for Large Vision-Language Models (LVLMs) to generalize well across
a broad range of vision-language (VL) tasks. However, training on large VL
datasets can become prohibitively expensive. In this work, we introduce
COINCIDE, an effective and scalable data selection technique that uses a small
model as a reference model to select visual instruction tuning data for
efficient finetuning of a target LVLM, focusing on diversity and
transferability. Specifically, we cluster the training data using internal
activations from a small model, which identifies VL concept-skill compositions
needed by a target LVLM. We then sample data from these diverse clusters by
considering their density and transferability, or the ability to transfer well
to other concept-skill compositions. This approach ensures the diversity of
these compositions, which is vital for LVLM generalization. Extensive
experiments demonstrate that COINCIDE achieves superior performance and data
selection efficiency against 8 strong baselines on two distinct datasets:
LLaVA-1.5 and Vision-Flan. Using only 20
achieves performance comparable to the LVLM finetuned on the whole dataset,
with 70
our method achieves superior results with only 16.7
MoreTranslated text
AI Read Science
Must-Reading Tree
Example
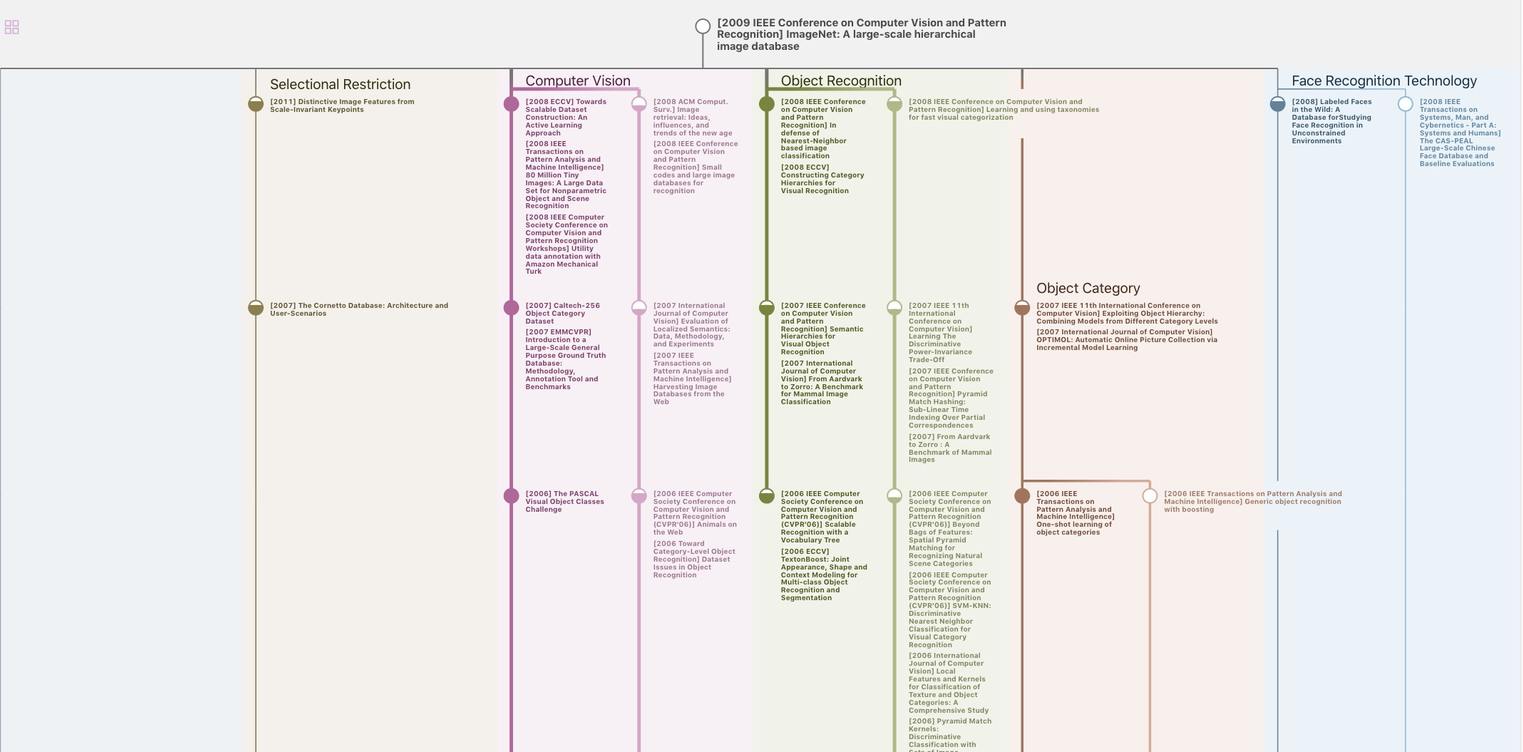
Generate MRT to find the research sequence of this paper
Chat Paper
Summary is being generated by the instructions you defined