Classification of Breast Lesions Using Mammary Sinograms and Deep Learning
PATTERN RECOGNITION, MCPR 2024(2024)
Abstract
Breast cancer is a disease that affects many women worldwide. Therefore, early detection of breast lesions is essential for effective diagnosis and treatment. In this context, digital breast tomosynthesis (DBT) is a promising technique for improving lesion detection compared to conventional mammography. However, image reconstruction can lead to a loss of information. Sinograms provide sufficient information for identifying and localizing lesions while reducing the noise and artifacts associated with the image reconstruction process. In this paper, we propose to employ image processing and deep learning techniques, especially convolutional neural networks (CNN), to identify patterns and extract relevant information from DBT sinograms. The objective is to facilitate diagnosis and treatment through the analysis of sinograms, allowing the identification of patterns and the extraction of useful information for continuous monitoring and evaluation of treatment over time. The results show that the ResNet50 and ResNet18 models have demonstrated a solid performance in classifying breast lesions from sinograms, with outstanding results in accuracy, recall, and F1-score of 94.96%, supporting their efficacy in this task. It is essential to highlight that although there are studies on sinograms in other fields, their application in diagnosing breast lesions has not yet been explored.
MoreTranslated text
Key words
sinograms,DBT,detection,classification,CNN,breast lesions
求助PDF
上传PDF
View via Publisher
AI Read Science
AI Summary
AI Summary is the key point extracted automatically understanding the full text of the paper, including the background, methods, results, conclusions, icons and other key content, so that you can get the outline of the paper at a glance.
Example
Background
Key content
Introduction
Methods
Results
Related work
Fund
Key content
- Pretraining has recently greatly promoted the development of natural language processing (NLP)
- We show that M6 outperforms the baselines in multimodal downstream tasks, and the large M6 with 10 parameters can reach a better performance
- We propose a method called M6 that is able to process information of multiple modalities and perform both single-modal and cross-modal understanding and generation
- The model is scaled to large model with 10 billion parameters with sophisticated deployment, and the 10 -parameter M6-large is the largest pretrained model in Chinese
- Experimental results show that our proposed M6 outperforms the baseline in a number of downstream tasks concerning both single modality and multiple modalities We will continue the pretraining of extremely large models by increasing data to explore the limit of its performance
Upload PDF to Generate Summary
Must-Reading Tree
Example
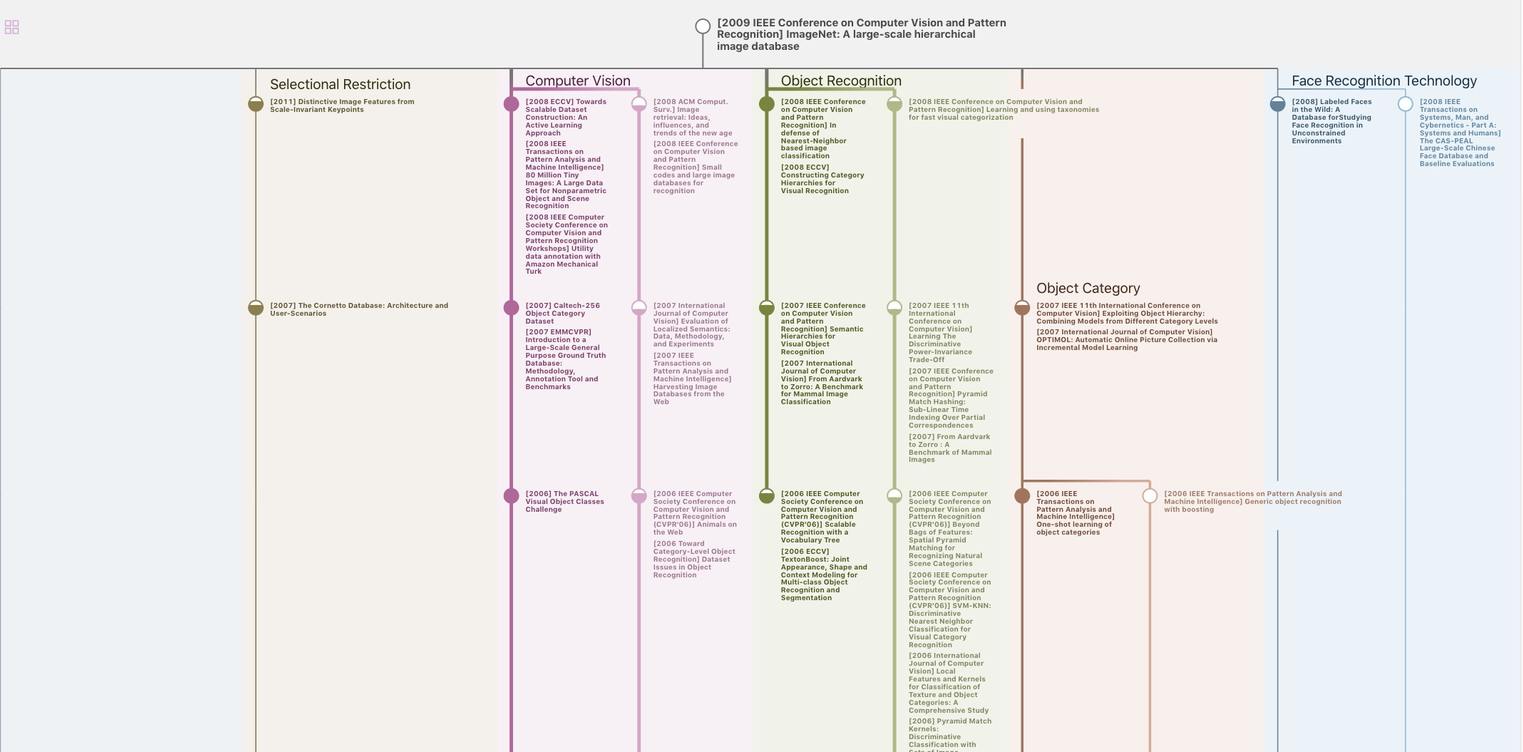
Generate MRT to find the research sequence of this paper
Data Disclaimer
The page data are from open Internet sources, cooperative publishers and automatic analysis results through AI technology. We do not make any commitments and guarantees for the validity, accuracy, correctness, reliability, completeness and timeliness of the page data. If you have any questions, please contact us by email: report@aminer.cn
Chat Paper