BrainLossNet: a Fast, Accurate and Robust Method to Estimate Brain Volume Loss from Longitudinal MRI
International Journal of Computer Assisted Radiology and Surgery(2024)
摘要
MRI-derived brain volume loss (BVL) is widely used as neurodegeneration marker. SIENA is state-of-the-art for BVL measurement, but limited by long computation time. Here we propose “BrainLossNet”, a convolutional neural network (CNN)-based method for BVL-estimation. BrainLossNet uses CNN-based non-linear registration of baseline(BL)/follow-up(FU) 3D-T1w-MRI pairs. BVL is computed by non-linear registration of brain parenchyma masks segmented in the BL/FU scans. The BVL estimate is corrected for image distortions using the apparent volume change of the total intracranial volume. BrainLossNet was trained on 1525 BL/FU pairs from 83 scanners. Agreement between BrainLossNet and SIENA was assessed in 225 BL/FU pairs from 94 MS patients acquired with a single scanner and 268 BL/FU pairs from 52 scanners acquired for various indications. Robustness to short-term variability of 3D-T1w-MRI was compared in 354 BL/FU pairs from a single healthy men acquired in the same session without repositioning with 116 scanners (Frequently-Traveling-Human-Phantom dataset, FTHP). Processing time of BrainLossNet was 2–3 min. The median [interquartile range] of the SIENA-BrainLossNet BVL difference was 0.10
更多查看译文
关键词
Magnetic resonance imaging,Brain volume loss,Convolutional neural network,Multiple sclerosis
AI 理解论文
溯源树
样例
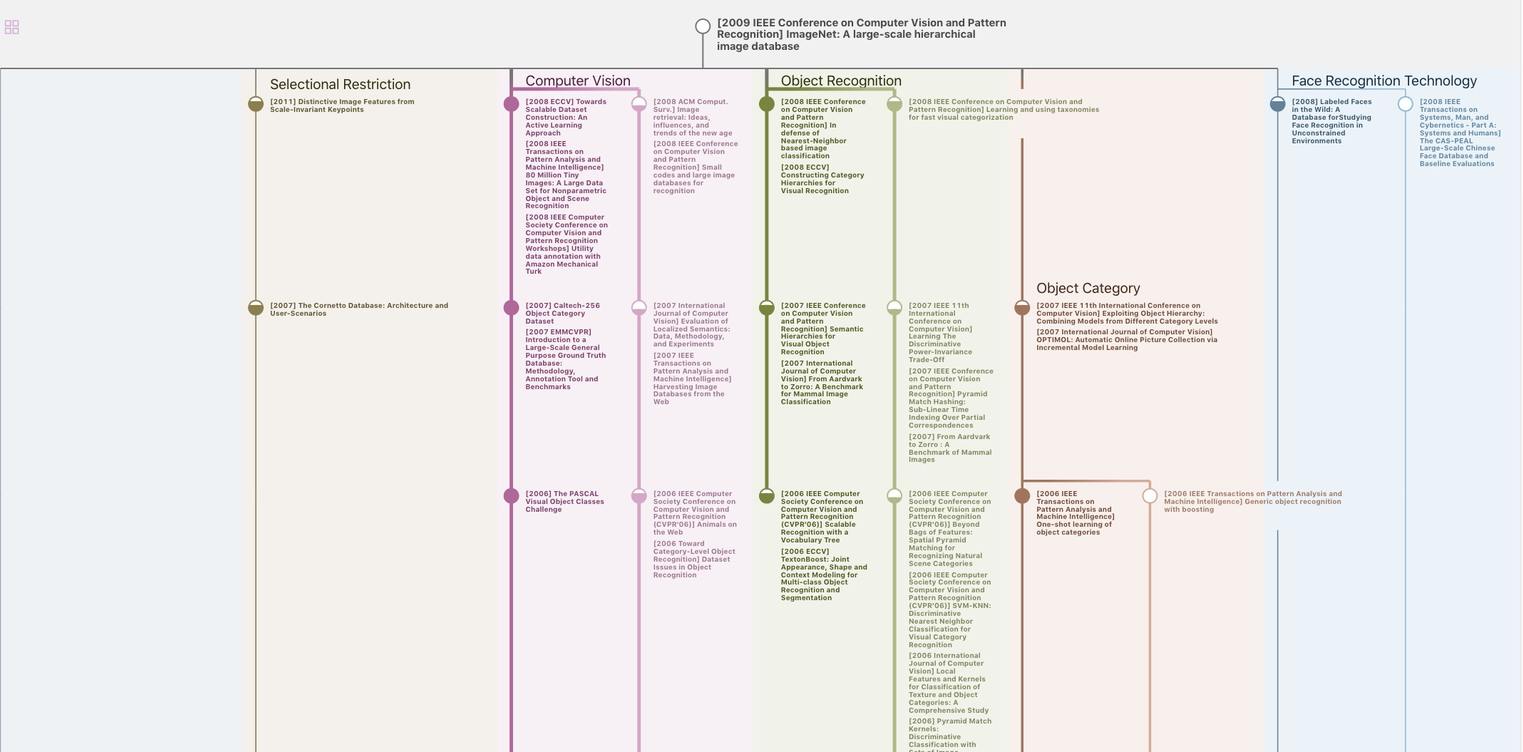
生成溯源树,研究论文发展脉络
Chat Paper
正在生成论文摘要