Adaptive Graph Spatial-Temporal Attention Networks for Long Lead ENSO Prediction
Expert Systems with Applications(2024)
摘要
El Niño-Southern Oscillation (ENSO) is a crucial factor in global climate change, which can lead to disasters such as floods, droughts, and heavy rainfall worldwide. Although accurate long-lead prediction of ENSO is essential, the intricate time-varying spatial correlations make it challenging. In this paper, we propose a novel deep learning architecture, called Adaptive Graph Spatial-Temporal Attention Network (AGSTAN), to model the extensive spatial–temporal interactions in ENSO. We first develop a dynamic spatial graph module with self-attention mechanism, wherein the structure is adapted to the characteristics of the evolving states. Furthermore, we introduce the long-term temporal sequence module to capture long-range dependency between time-series outputs and inputs, and generate accurate long lead predictions with only one forward step. To validate its efficacy and robustness, we conducted experiments on the simulated and historical climate datasets. Results showcase that AGSTAN possesses superior performance in ENSO prediction, outperforming contemporary deep learning models and boasting a 23-month forecasting horizon with heightened correlation and minimized bias.
更多查看译文
关键词
El Niño-Southern Oscillation,Long-term prediction,Gynamic spatial graph,Long-term temporal sequence
AI 理解论文
溯源树
样例
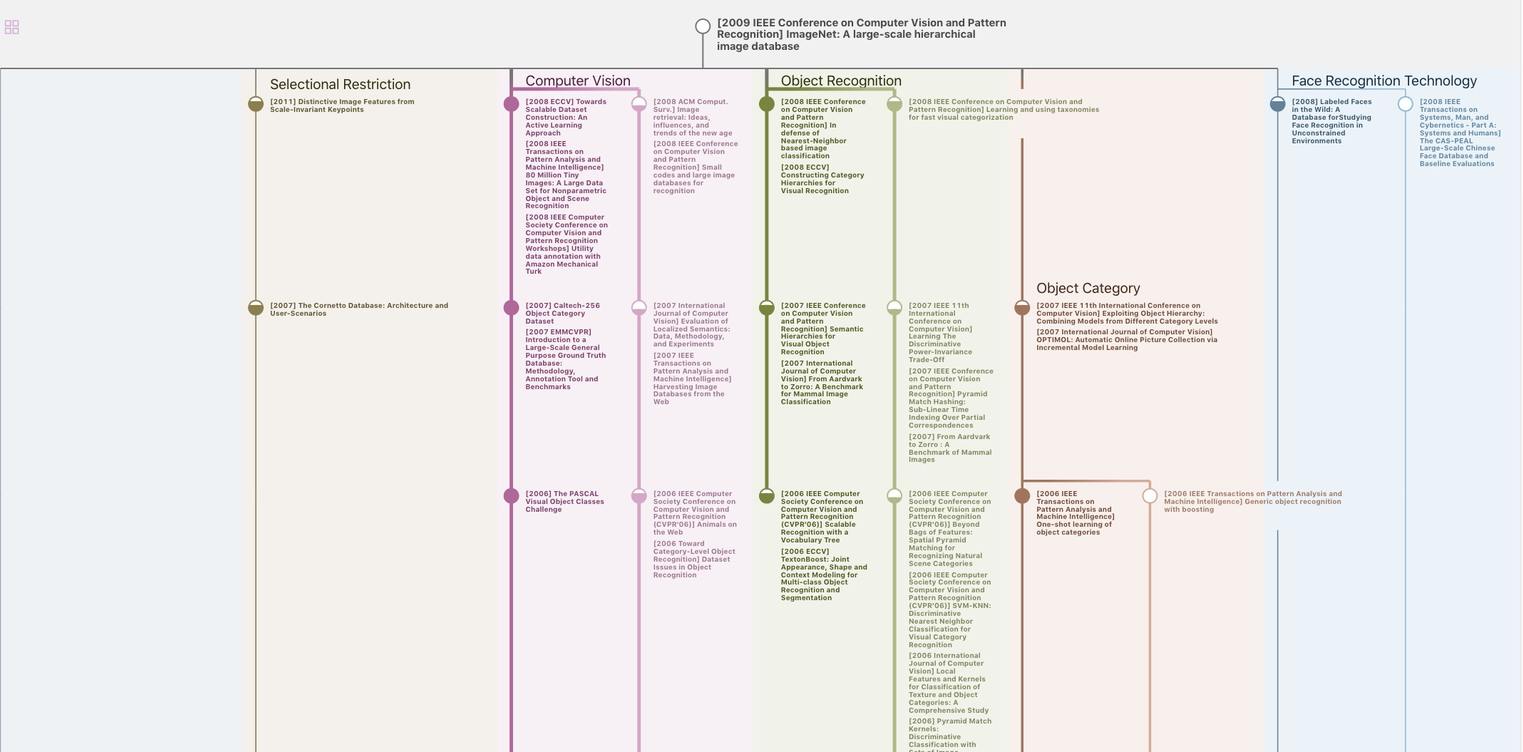
生成溯源树,研究论文发展脉络
Chat Paper
正在生成论文摘要