Privacy-Preserving Naive Bayesian Classification for Health Monitoring Systems
IEEE TRANSACTIONS ON INDUSTRIAL INFORMATICS(2024)
摘要
As the Internet of Medical Things booms, the cloud-assisted health monitoring service has attracted extensive attention. The medical institutions often use the Na & iuml;ve Bayesian classification technology to establish the medical inference models. These models can be outsourced to cloud servers, allowing the remote users without models to utilize well-performing models for medical diagnosis. Existing Na & iuml;ve Bayesian secure outsourcing schemes mostly use heavy cryptographic primitives or pure additive secret sharing (ASS) technology. In this article, we use searchable encryption technology combined with ASS to design a privacy-preserving Na & iuml;ve Bayesian classification scheme that can protect the medical institutions' models, the users' medical data, and the final inference result made by cloud servers. Compared with the state-of-the-art, our scheme further reduces the number of communications between the user and the cloud server and reduces the computation complexity of cloud servers from O(dtf) to O(dt). We provide the formal security analysis to show that our scheme ensures the necessary security. Through experiments on multiple datasets, we show that our scheme can efficiently handle the classification requests in the test dataset in less than 100 ms.
更多查看译文
关键词
Cryptography,Medical diagnostic imaging,Bayes methods,Servers,Monitoring,Computational modeling,Cloud computing,Health monitoring service,machine learning,Na & iuml,ve Bayesian classifier (NBC),privacy preserving
AI 理解论文
溯源树
样例
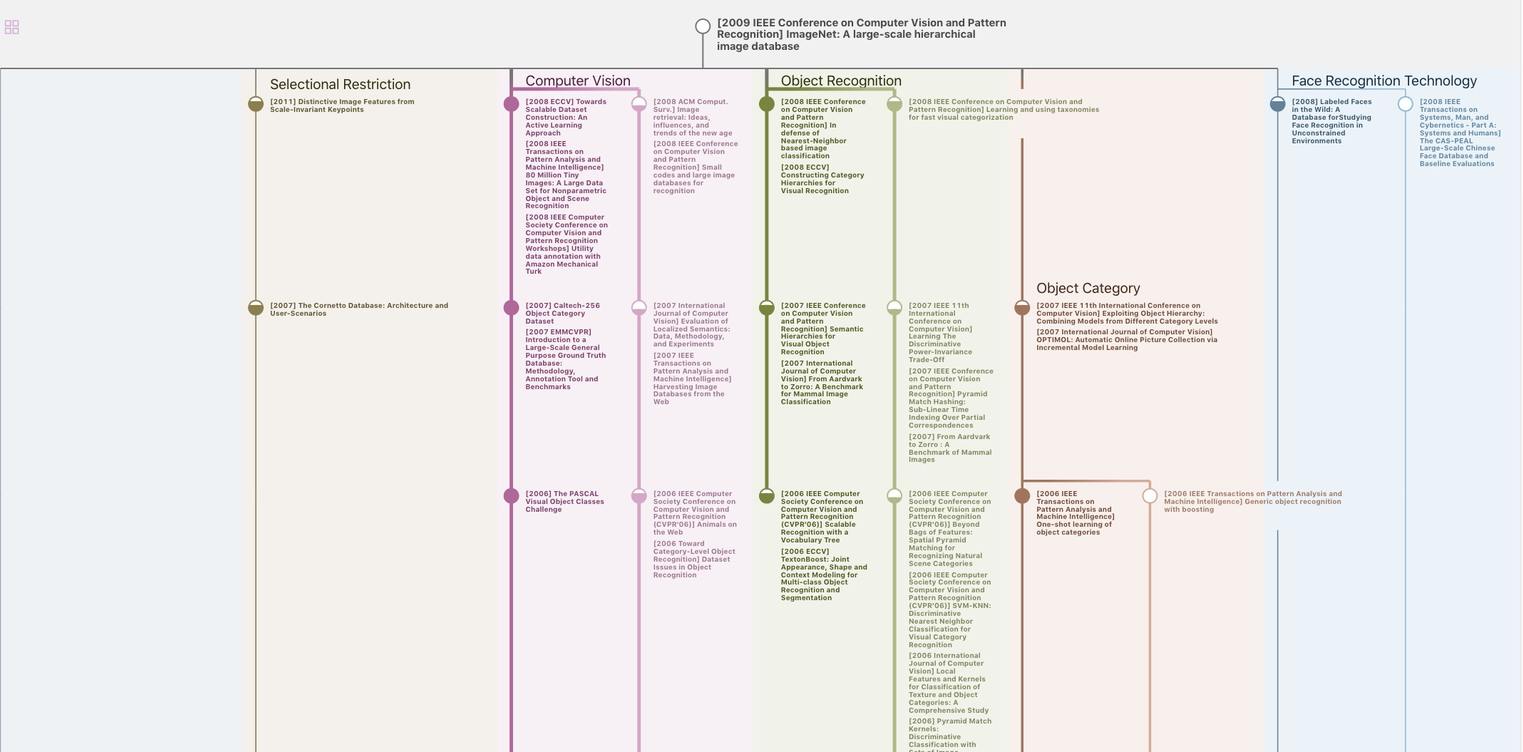
生成溯源树,研究论文发展脉络
Chat Paper
正在生成论文摘要