HHGNN: Heterogeneous Hypergraph Neural Network for Traffic Agents Trajectory Prediction in Grouping Scenarios
ICRA 2024(2024)
Abstract
In many intelligent transportation systems, predicting the future motion of heterogeneous traffic participants is a fundamental but challenging task due to various factors encompassing the agents' dynamic states, interactions with neighboring agents and surrounding traffic infrastructures, and their stochastic and multi-modal natural behavior tendencies. However, existing approaches have limitations as they either focus solely on static, pairwise interactions, ignoring interactions of varied granularity, or fail to tackle agents' heterogeneity. In this paper, instead of focusing solely on pairwise interactions, we propose a Heterogenous Hypergraph Graph Neural Network (HHGNN) based motion prediction model that leverages the nature of hypergraph to encode the groupwise interactions among traffic participants. Moreover, we propose the type-aware two-level hypergraph message passing module (TTHMS) with learnable hyperedge-type embeddings to model the intra-group and inter-group level interactions among heterogeneous traffic agents (e.g., vehicles, pedestrians, and cyclists). Besides, We integrate a scene context fusion layer in TTHMS to incorporate the scene context. Comparison and ablation experiments on the Waymo Open Motion Dataset (WOMD) demonstrate HHGNN's effectiveness within the motion prediction task.
MoreTranslated text
Key words
Autonomous Vehicle Navigation,Autonomous Agents,AI-Based Methods
AI Read Science
Must-Reading Tree
Example
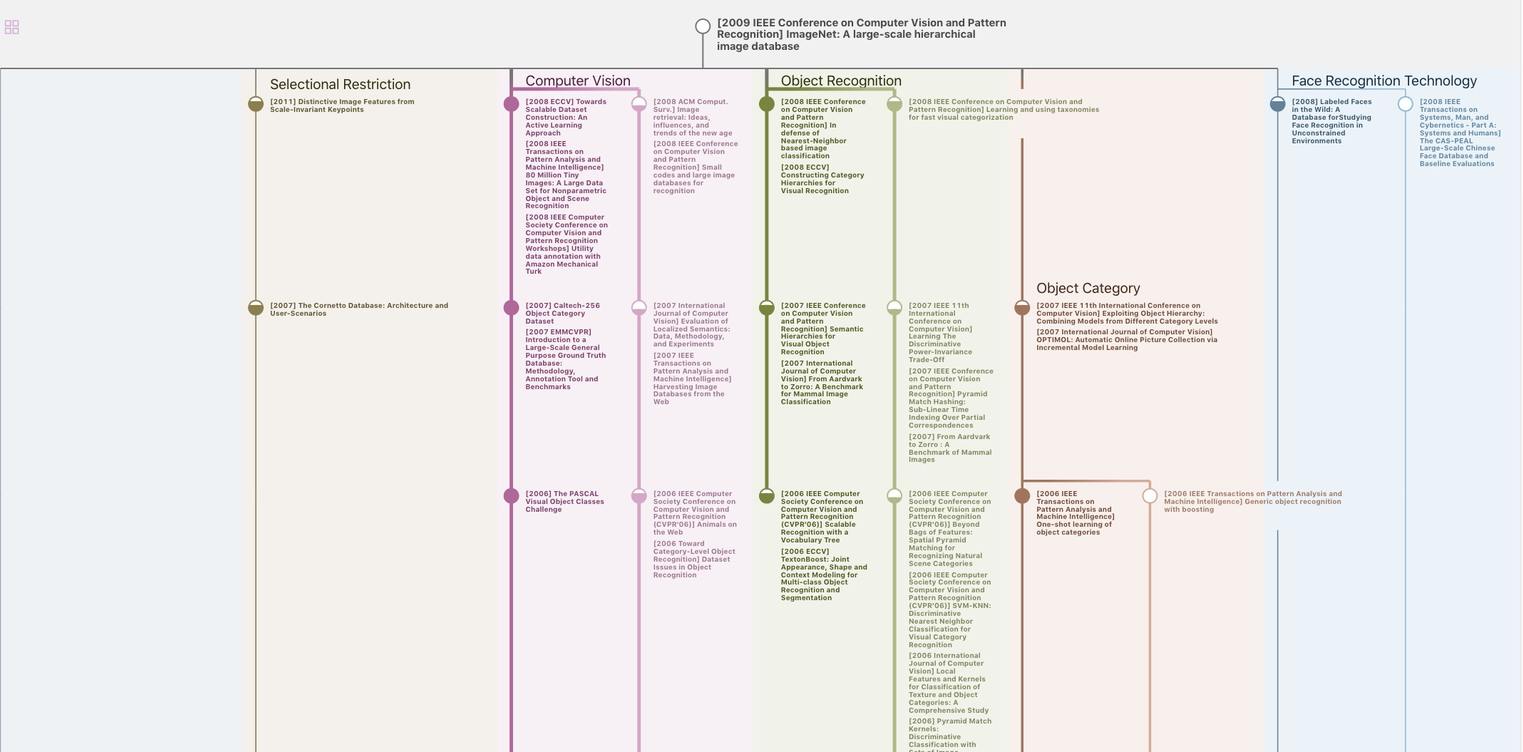
Generate MRT to find the research sequence of this paper
Chat Paper
Summary is being generated by the instructions you defined