Temporal Dynamics and Physical Priori Multimodal Network for Rehabilitation Physical Training Evaluation
IEEE journal of biomedical and health informatics(2024)
摘要
Sensor-based rehabilitation physical training assessment methods have attracted significant attention in refined evaluation scenarios. A refined rehabilitation evaluation method combines the expertise of clinicians with advanced sensor-based technology to capture and analyze subtle movement variations often unobserved by traditional subjective methods. Current approaches center on either body postures or muscle strength, which lack more sophisticated analysis features of muscle activation and coordination, thereby hindering analysis efficacy in deep rehabilitation feature exploration. To address this issue, we present a multimodal network algorithm that integrates surface electromyography (sEMG) and stress distribution signals. The algorithm considers the physical knowledge a priori to interpret the current rehabilitation stage and efficiently handles temporal dynamics arising from diverse user profiles in an online setting. Besides, we verified the performance of this model using a learned-nonuse phenomenon assessment task in 24 subjects, achieving an accuracy of 94.7%. Our results surpass those of conventional feature-based, distance-based, and ensemble baseline models, highlighting the advantages of incorporating multimodal information rather than relying solely on unimodal data. Moreover, the proposed model presents a network design solution for rehabilitation physical training that requires deep bioinformatic features and can potentially assist real-time and home-based physical training work.
更多查看译文
关键词
Rehabilitation physical training,flexible sensors,multimodal fusion,bioinformatics,deep learning
AI 理解论文
溯源树
样例
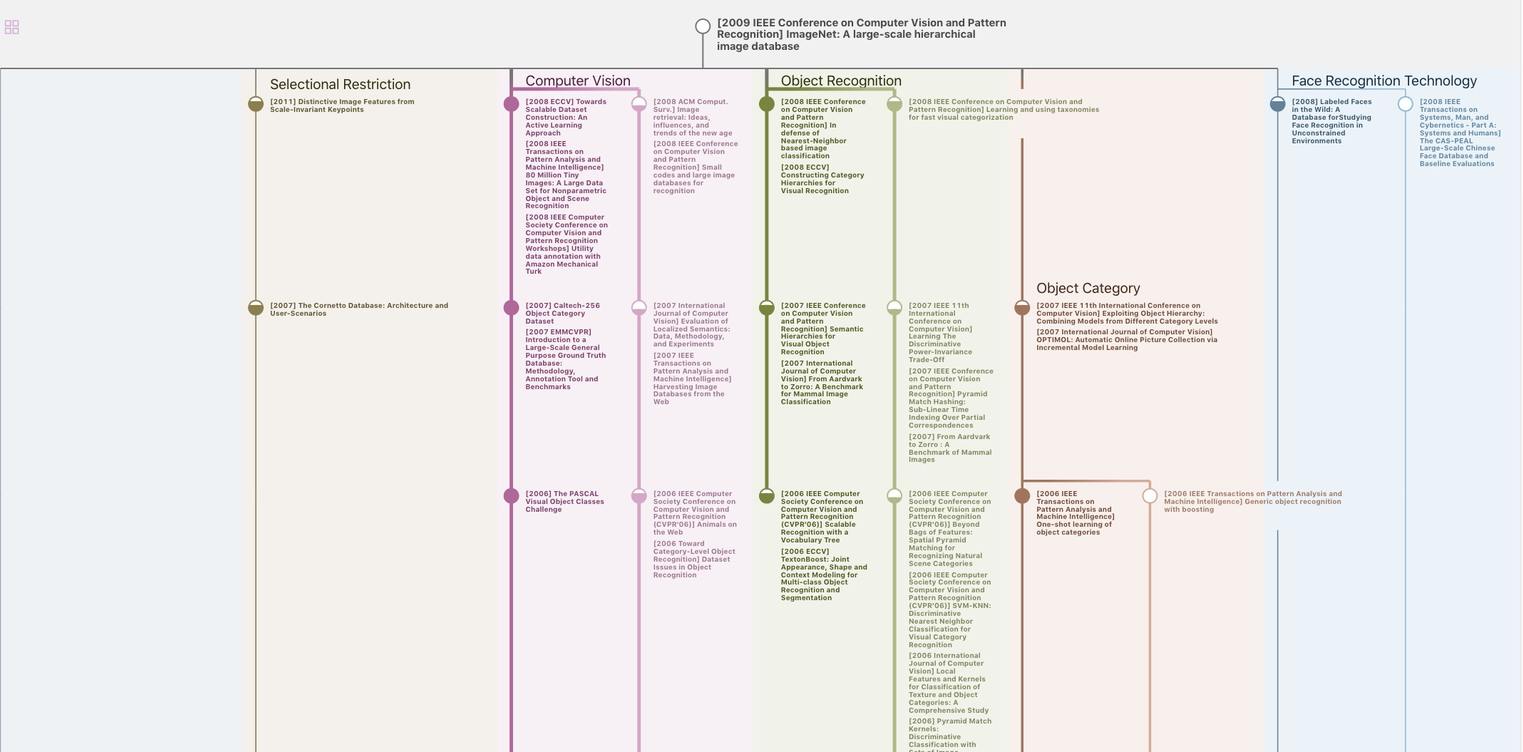
生成溯源树,研究论文发展脉络
Chat Paper
正在生成论文摘要