Developing Adaptive Solutions for Accurate Pneumonia Diagnosis via Chest X-Ray Analysis
2024 Third International Conference on Distributed Computing and Electrical Circuits and Electronics (ICDCECE)(2024)
摘要
Chest radiography serves as a cost-effective and powerful investigative method, providing vital respiratory information crucial for pneumonia detection. Recent advancements in artificial intelligence (AI) have led to the development of AI systems by researchers and radiologists capable of accurately diagnosing pneumonia from chest X-ray images using deep neural networks. However, the robustness and adaptability of these systems, trained using empirical risk minimization (ERM), still pose significant challenges. ERM lacks the capability to discern environment-specific spurious features and consider confounders, leading to a scenario where systems appear accurate but fail when tested in new hospital settings. To address this challenge and achieve a more robust and adaptable diagnosis of pneumonia, we propose two key ideas: firstly, replacing ERM with a robust training routine such as Invariant Risk Minimization (IRM) and virtual adversarial training (v-REx) to eliminate spurious features; and secondly, introducing a novel modular architecture that separately encodes invariant features in a self-supervised manner and style confounders. We validate the impact of each contribution individually through two experiments conducted on real-world data, aiming to enhance the reliability and generalizability of pneumonia diagnosis systems. Proposed method achieves the accuracy of 90.23 % for different datasets.
更多查看译文
关键词
pneumonia,chest X-ray images,artificial intelligence,accuracy,diagnosis
AI 理解论文
溯源树
样例
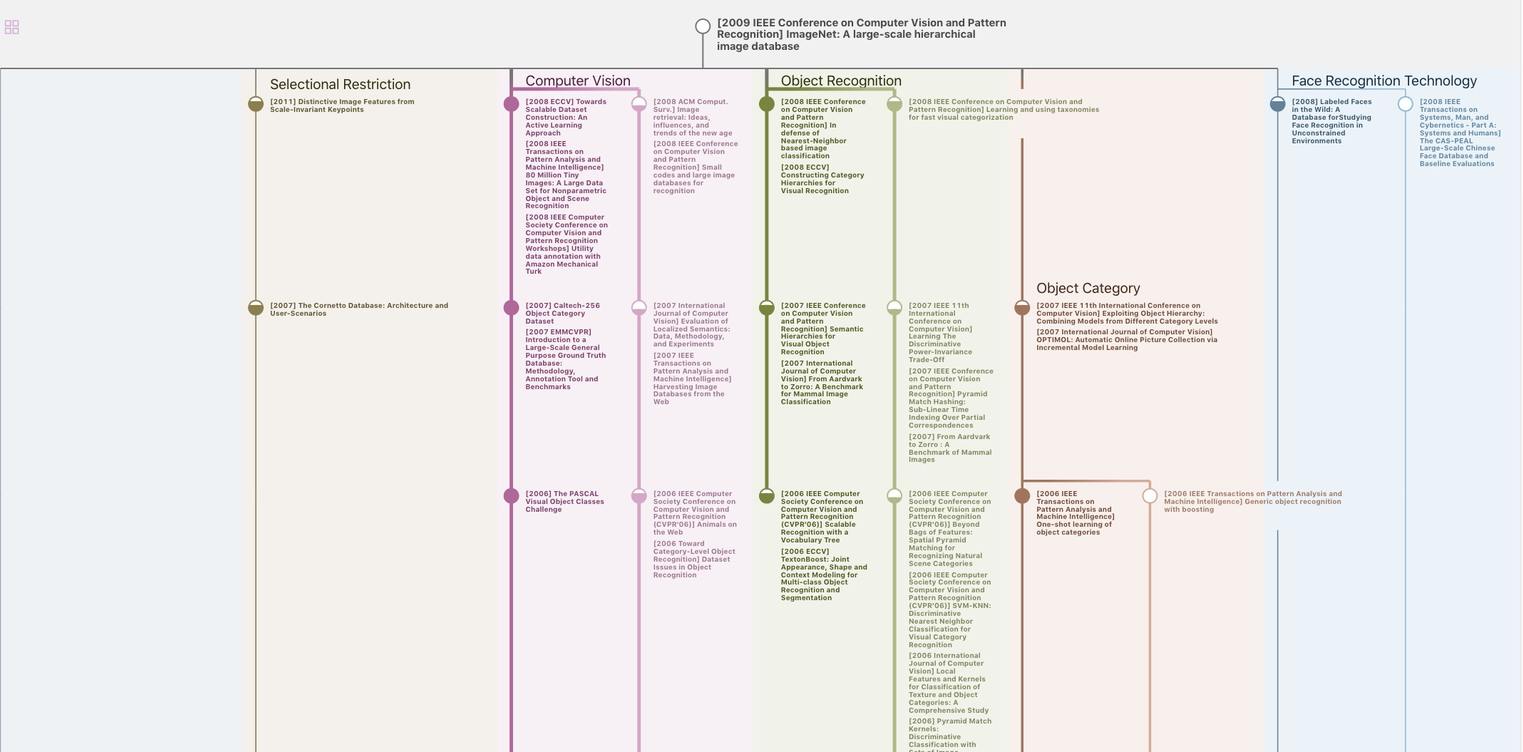
生成溯源树,研究论文发展脉络
Chat Paper
正在生成论文摘要