Improving Selection Diversity Using Hybrid Graph-Based News Recommenders
User Modeling and User-Adapted Interaction(2024)
摘要
With the ever-growing amount of news, there is an increasing need for tools capable of filtering out and tailoring the content to the wants and needs of the reader. Over the last decade, researchers have noted that the inclusion of objectives such as diversity, novelty, and serendipity plays a vital role in further improving recommender systems’ perceived value and effectiveness. However, most of these studies limit themselves to a narrow definition of diversity; they only consider individual lists of recommendations, and the impact of diversity on the actual reading behavior of users is typically not examined. In this paper, we present a news recommender system that addresses this problem and aims to increase the diversity of content selected by the user. We propose a pre-filtering graph-based approach of extending the user profile to nudge him/her along a path toward unseen news topics. Results from an online experiment ( N = 338 ) show that (1) intra-list and selection diversity are significantly improved with negligible impact on accuracy and (2) that the use of diversity-optimized recommender systems can lead to an increase in user satisfaction.
更多查看译文
关键词
News recommender systems,Exposure diversity,Selection diversity,Nudging,Knowledge graphs
AI 理解论文
溯源树
样例
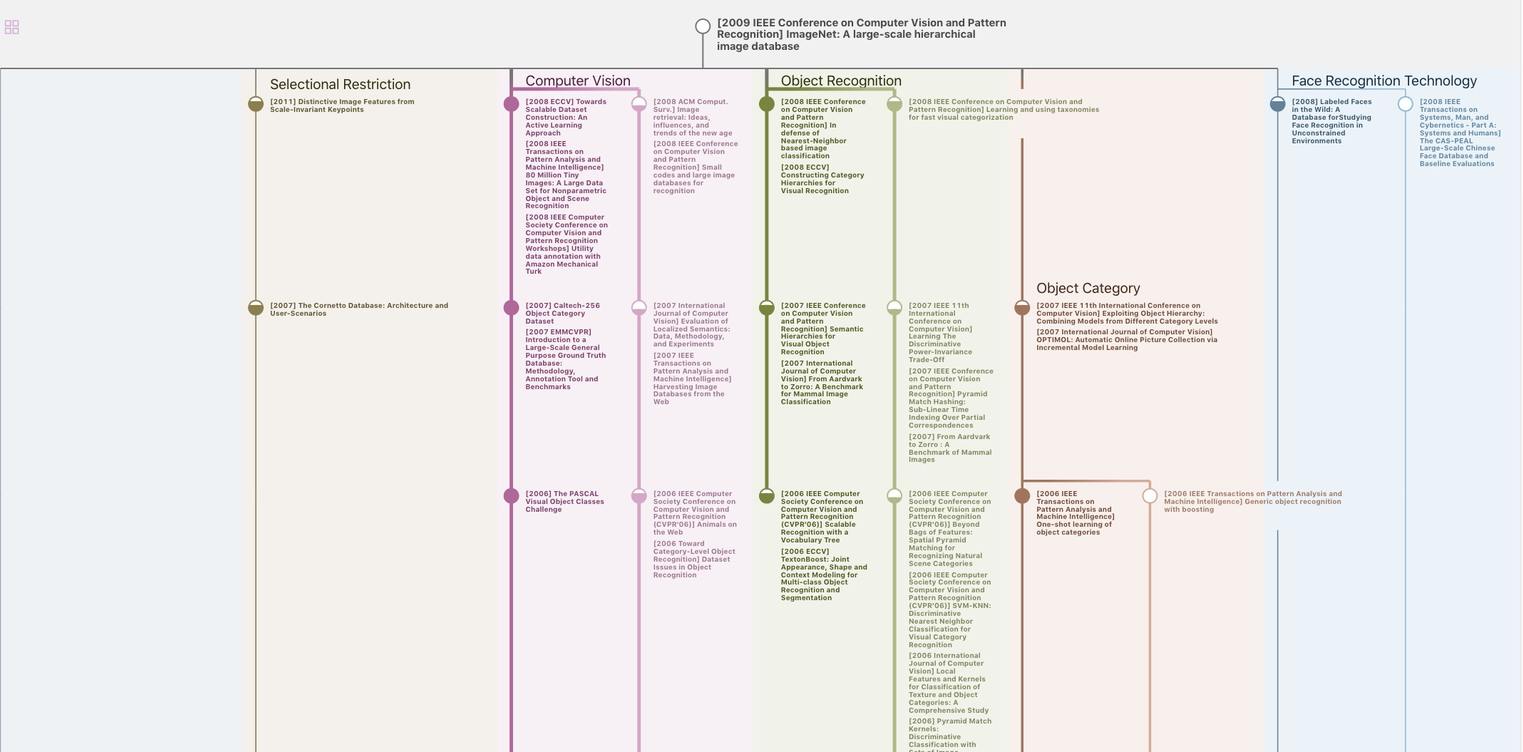
生成溯源树,研究论文发展脉络
Chat Paper
正在生成论文摘要