Transient Electromagnetic Inversion: A Machine Learning Approach With CNN-LightGBM.
IEEE Trans. Geosci. Remote. Sens.(2024)
摘要
The inversion of transient electromagnetic (TEM) data presents a complex nonlinear problem, and traditional inversion methods encounter certain limitations. Data-driven machine learning techniques, such as convolutional neural network (CNN), provide an alternative approach to TEM inversion. However, conventional CNN faces challenges such as limited generalization capability. Here we propose a novel TEM inversion method called CNN-LightGBM that combines the strengths of CNN and the light gradient boosting machine (LightGBM). Specifically, CNN is employed to extract crucial features from TEM responses, which are then regressed using LightGBM to improve the reliability of the inversion results. The proposed method is tested on synthetic and measured data, and its performance is compared against existing methods. Synthetic tests demonstrate that, compared to CNN, the proposed model provides more reliable inversion results in the entire depth range, exhibiting superior generalization capability and noise robustness. Meanwhile, the inversion efficiency of this method is remarkably high, allowing for the inversion of 7200 points of TEM data in just one seconds on a common PC. Additionally, the successful application of the trained CNN-LightGBM in the inversion of measured data also proves the effectiveness of the method. The model can rapidly provide reliable inversion results and has tremendous potential for near real-time imaging of subsurface structures.
更多查看译文
关键词
Deep learning,Transient electromagnetic method,Inversion,Ensemble learning,Gradient boosting
AI 理解论文
溯源树
样例
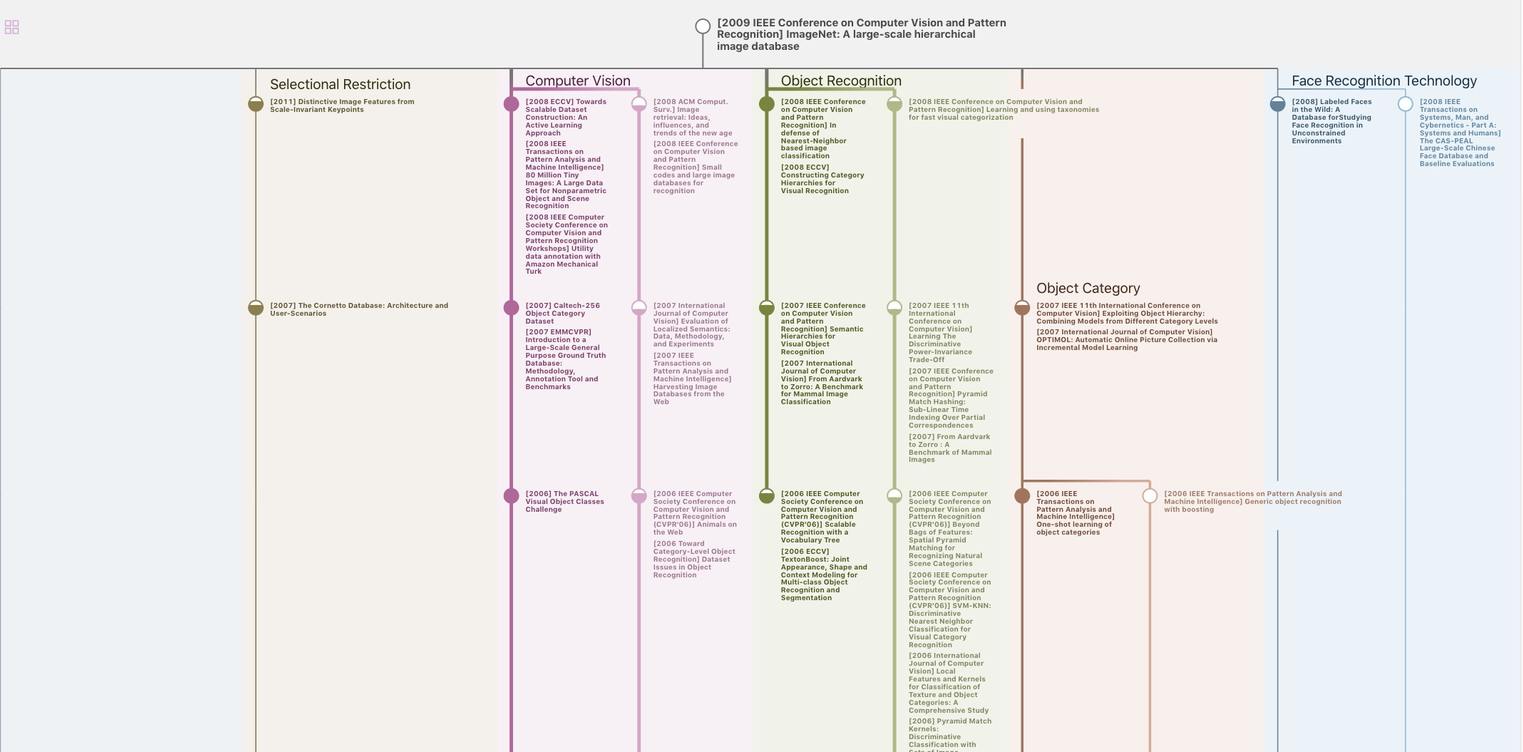
生成溯源树,研究论文发展脉络
Chat Paper
正在生成论文摘要