Sparse Graph Tensor Learning for Multi-View Spectral Clustering
IEEE Transactions on Emerging Topics in Computational Intelligence(2024)
摘要
Multi-view spectral clustering has achieved impressive performance by learning multiple robust and meaningful similarity graphs for clustering. Generally, the existing literatures often construct multiple similarity graphs by certain similarity measure (e.g. the Euclidean distance), which lack the desired ability to learn sparse and reliable connections that carry critical information in graph learning while preserving the low-rank structure. Regarding the challenges, a novel Sparse Graph Tensor Learning for Multi-view Spectral Clustering (SGTL) method is designed in this paper, where multiple similarity graphs are seamlessly coupled with the cluster indicators and constrained with a low-rank graph tensor. Specifically, a novel graph learning paradigm is designed by establishing an explicit theoretical connection between the similarity matrices and the cluster indicator matrices, in order that the constructed similarity graphs enjoy the desired block diagonal and sparse property for learning a small portion of reliable links. Then, we stack multiple similarity matrices into a low-rank graph tensor to better preserve the low-rank structure of the reliable links in graph learning, where the key knowledge conveyed by singular values from different views is explicitly considered. Extensive experiments on several benchmark datasets demonstrate the superiority of SGTL.
更多查看译文
关键词
Multi-view clustering,similarity learning graph,low-rank graph tensor,key knowledge,reliable links
AI 理解论文
溯源树
样例
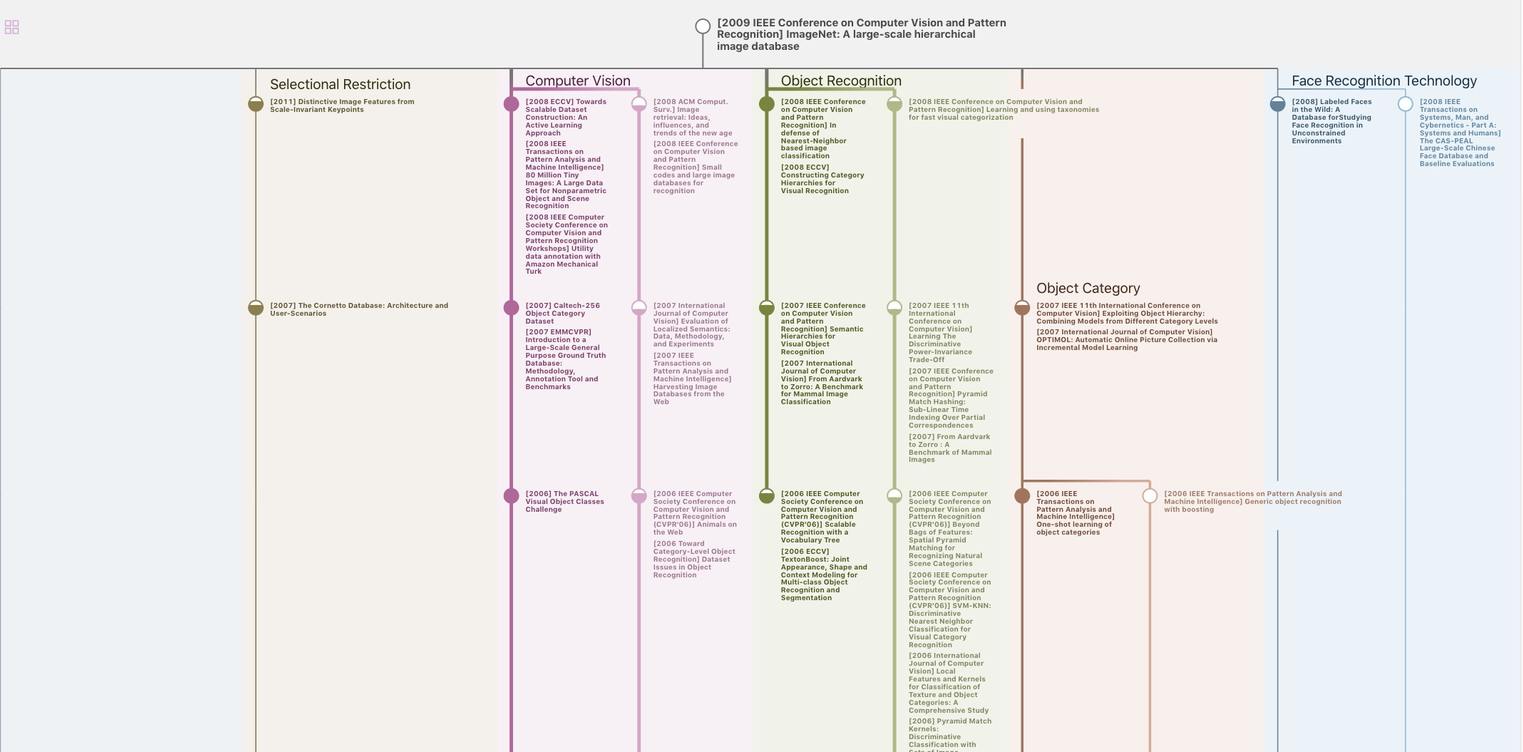
生成溯源树,研究论文发展脉络
Chat Paper
正在生成论文摘要