GPT4Rec: Graph Prompt Tuning for Streaming Recommendation
SIGIR 2024(2024)
摘要
In the realm of personalized recommender systems, the challenge of adaptingto evolving user preferences and the continuous influx of new users and itemsis paramount. Conventional models, typically reliant on a static training-testapproach, struggle to keep pace with these dynamic demands. Streamingrecommendation, particularly through continual graph learning, has emerged as anovel solution. However, existing methods in this area either rely onhistorical data replay, which is increasingly impractical due to stringent dataprivacy regulations; or are inability to effectively address the over-stabilityissue; or depend on model-isolation and expansion strategies. To tackle thesedifficulties, we present GPT4Rec, a Graph Prompt Tuning method for streamingRecommendation. Given the evolving user-item interaction graph, GPT4Rec firstdisentangles the graph patterns into multiple views. After isolating specificinteraction patterns and relationships in different views, GPT4Rec utilizeslightweight graph prompts to efficiently guide the model across varyinginteraction patterns within the user-item graph. Firstly, node-level promptsare employed to instruct the model to adapt to changes in the attributes orproperties of individual nodes within the graph. Secondly, structure-levelprompts guide the model in adapting to broader patterns of connectivity andrelationships within the graph. Finally, view-level prompts are innovativelydesigned to facilitate the aggregation of information from multipledisentangled views. These prompt designs allow GPT4Rec to synthesize acomprehensive understanding of the graph, ensuring that all vital aspects ofthe user-item interactions are considered and effectively integrated.Experiments on four diverse real-world datasets demonstrate the effectivenessand efficiency of our proposal.
更多查看译文
AI 理解论文
溯源树
样例
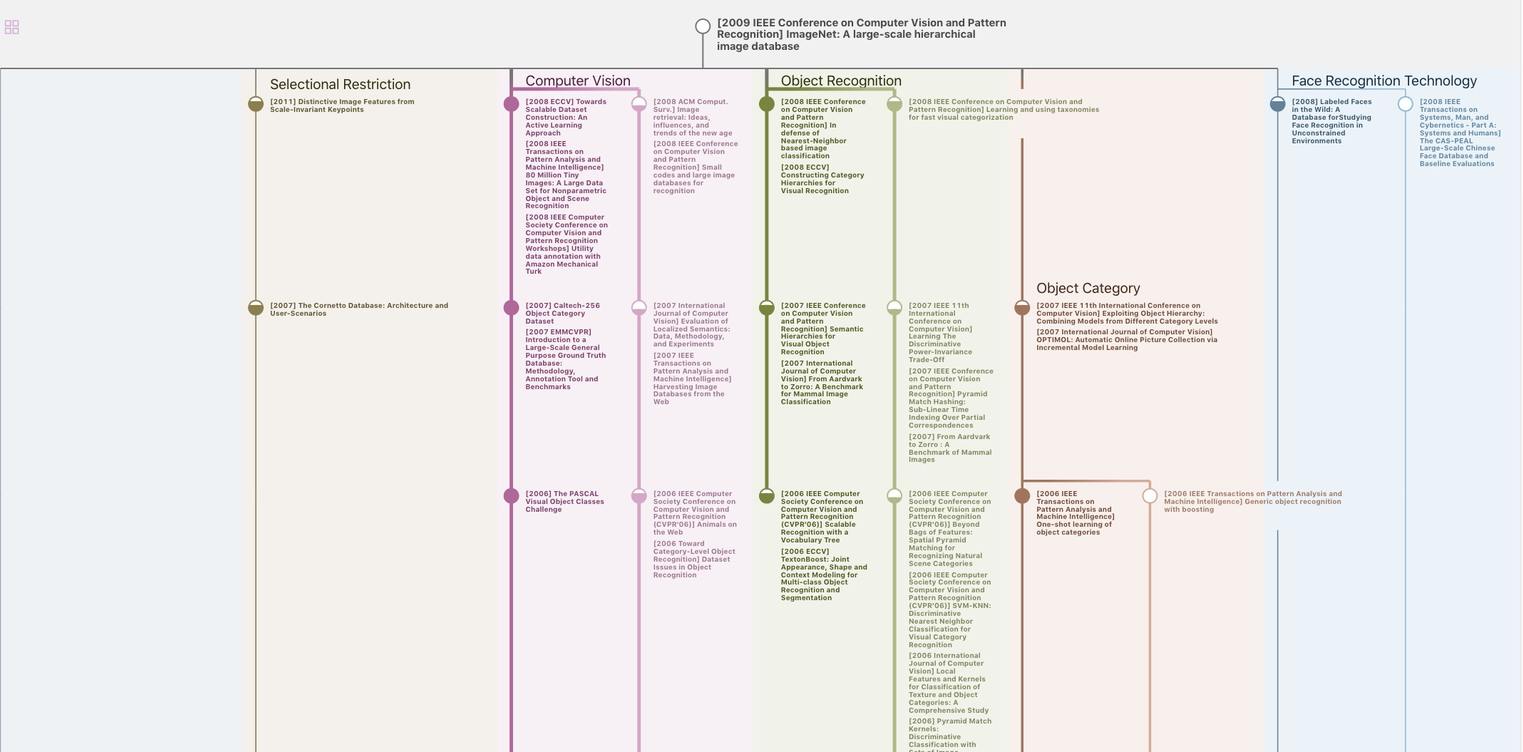
生成溯源树,研究论文发展脉络
Chat Paper
正在生成论文摘要