PRoDeliberation: Parallel Robust Deliberation for End-to-End Spoken Language Understanding
CoRR(2024)
摘要
Spoken Language Understanding (SLU) is a critical component of voiceassistants; it consists of converting speech to semantic parses for taskexecution. Previous works have explored end-to-end models to improve thequality and robustness of SLU models with Deliberation, however these modelshave remained autoregressive, resulting in higher latencies. In this work weintroduce PRoDeliberation, a novel method leveraging a Connectionist TemporalClassification-based decoding strategy as well as a denoising objective totrain robust non-autoregressive deliberation models. We show thatPRoDeliberation achieves the latency reduction of parallel decoding (2-10ximprovement over autoregressive models) while retaining the ability to correctAutomatic Speech Recognition (ASR) mistranscriptions of autoregressivedeliberation systems. We further show that the design of the denoising trainingallows PRoDeliberation to overcome the limitations of small ASR devices, and weprovide analysis on the necessity of each component of the system.
更多查看译文
AI 理解论文
溯源树
样例
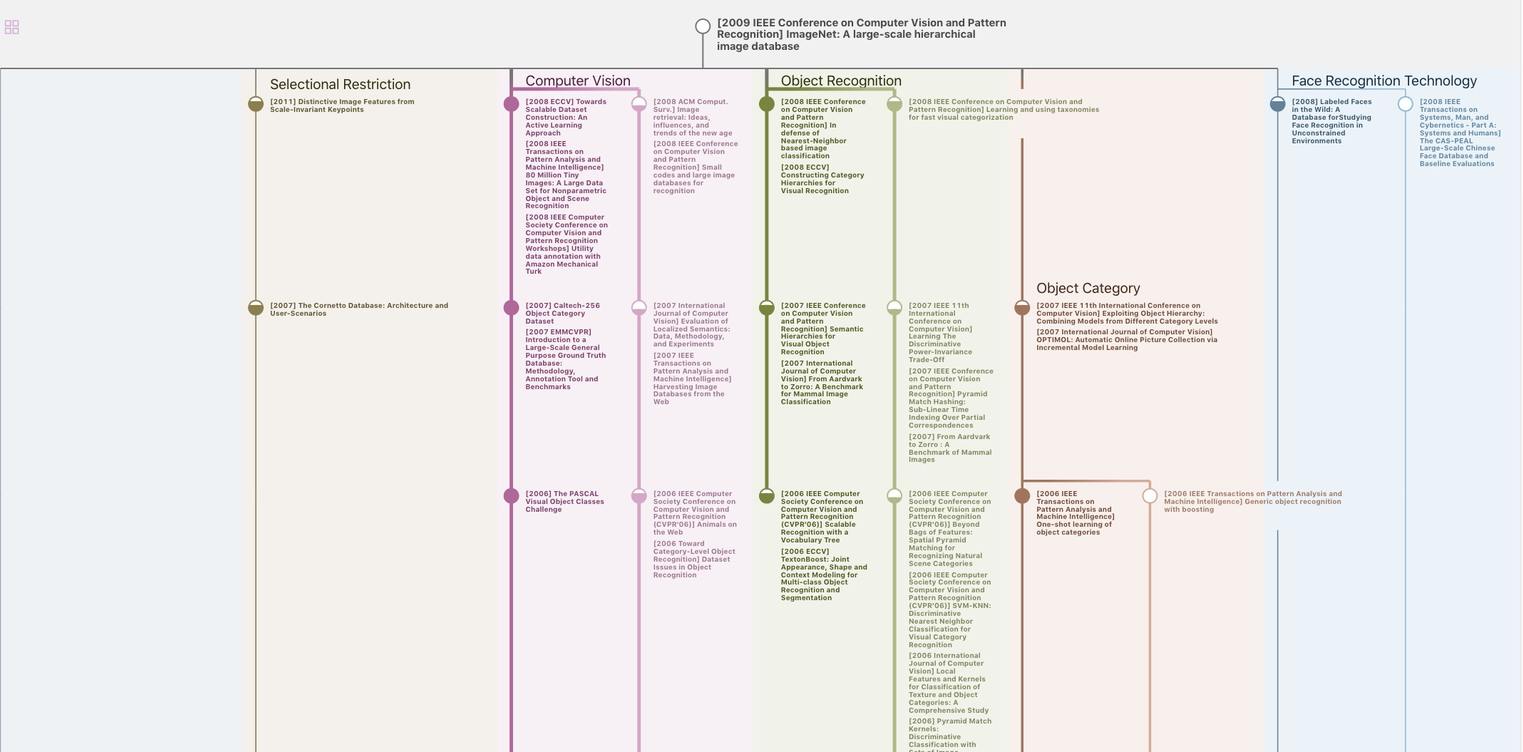
生成溯源树,研究论文发展脉络
Chat Paper
正在生成论文摘要