PETNet-Coincident Particle Event Detection using Spiking Neural Networks.
Neuro Inspired Computational Elements Workshop(2024)
摘要
Spiking neural networks (SNN) hold the promise of being a more biologically plausible, low-energy alternative to conventional artificial neural networks. Their time-variant nature makes them particularly suitable for processing time-resolved, sparse binary data. In this paper, we investigate the potential of leveraging SNNs for the detection of photon coincidences in positron emission tomography (PET) data. PET is a medical imaging technique based on injecting a patient with a radioactive tracer and detecting the emitted photons. One central post-processing task for inferring an image of the tracer distribution is the filtering of invalid hits occurring due to e.g. absorption or scattering processes. Our approach, coined PETNet, interprets the detector hits as a binary-valued spike train and learns to identify photon coincidence pairs in a supervised manner. We introduce a dedicated multi-objective loss function and demonstrate the effects of explicitly modeling the detector geometry on simulation data for two use-cases. Our results show that PETNet can outperform the state-of-the-art classical algorithm with a maximal coincidence detection F
1
of 95.2%. At the same time, PETNet is able to predict photon coincidences up to 36 times faster than the classical approach, highlighting the great potential of SNNs in particle physics applications.
更多查看译文
关键词
Spiking neural networks,positron emission tomography,particle coincidence detection,supervised denoising
AI 理解论文
溯源树
样例
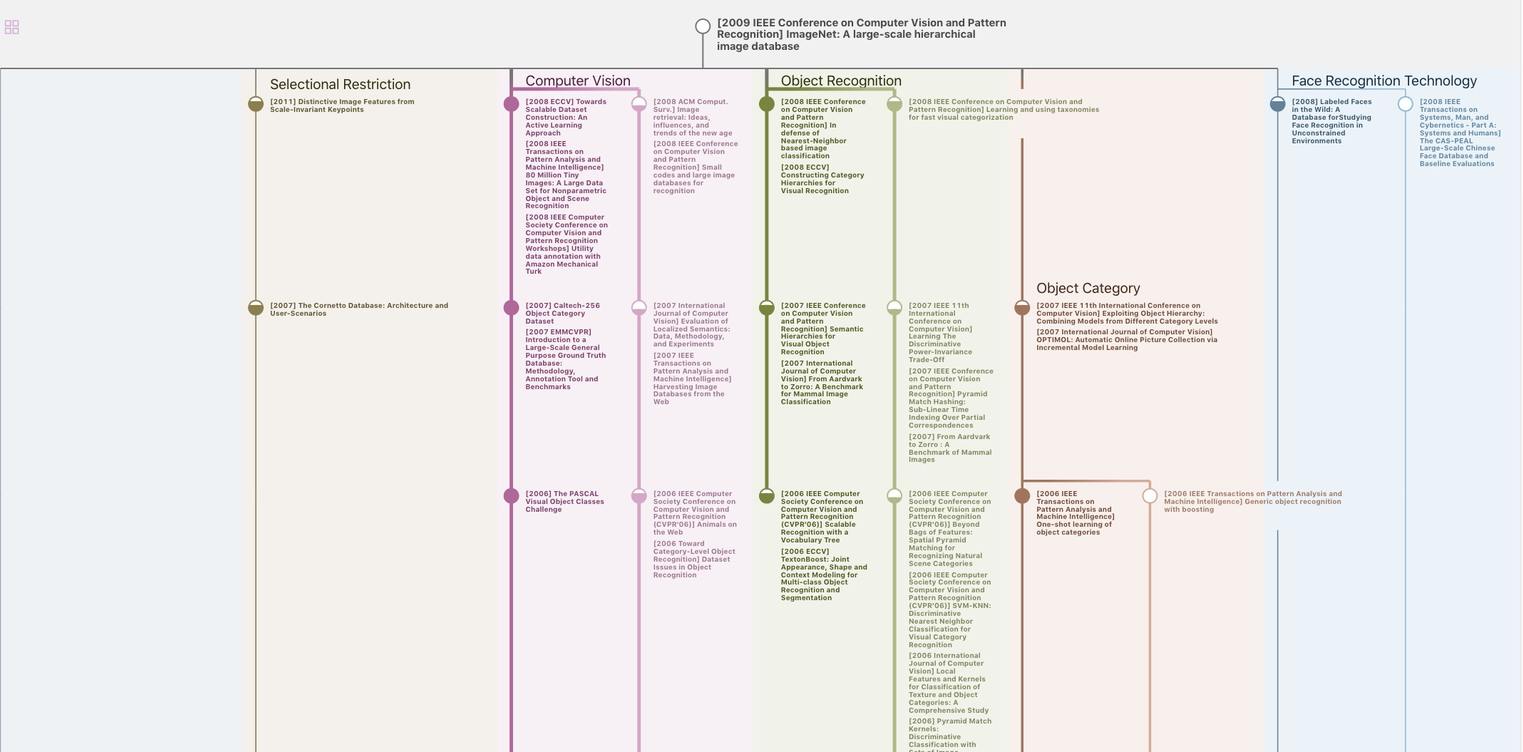
生成溯源树,研究论文发展脉络
Chat Paper
正在生成论文摘要