Learning to Floorplan like Human Experts via Reinforcement Learning.
Design, Automation, and Test in Europe(2024)
摘要
Deep reinforcement learning (RL) has gained popularity for automatically generating placements in modern chip design. However, the visual style of the fioorplans generated by these RL models is significantly different from the manual layouts' style, for RL placers usually only adopt metrics like wirelength and routing congestion as the reward in reinforcement learning, ignoring the complex and fine-grained layout experience of human experts. In this paper, we propose a placement scorer to rate the quality of layouts and apply abnormal detection to the fioorplanning task. In addition, we add the output of this scorer as a part of the reward for reinforcement learning of the placement process. Experimental results on ISPD 2005 benchmark show that our proposed placement quality scorer can evaluate the layouts according to human craft style efficiently, and that adding this scorer into reinforcement learning reward helps generating placements with shorter wirelength than previous methods for some circuit designs.
更多查看译文
关键词
Floorplan,Reinforcement Leaning,Abnormal Detection,Placement Scorer
AI 理解论文
溯源树
样例
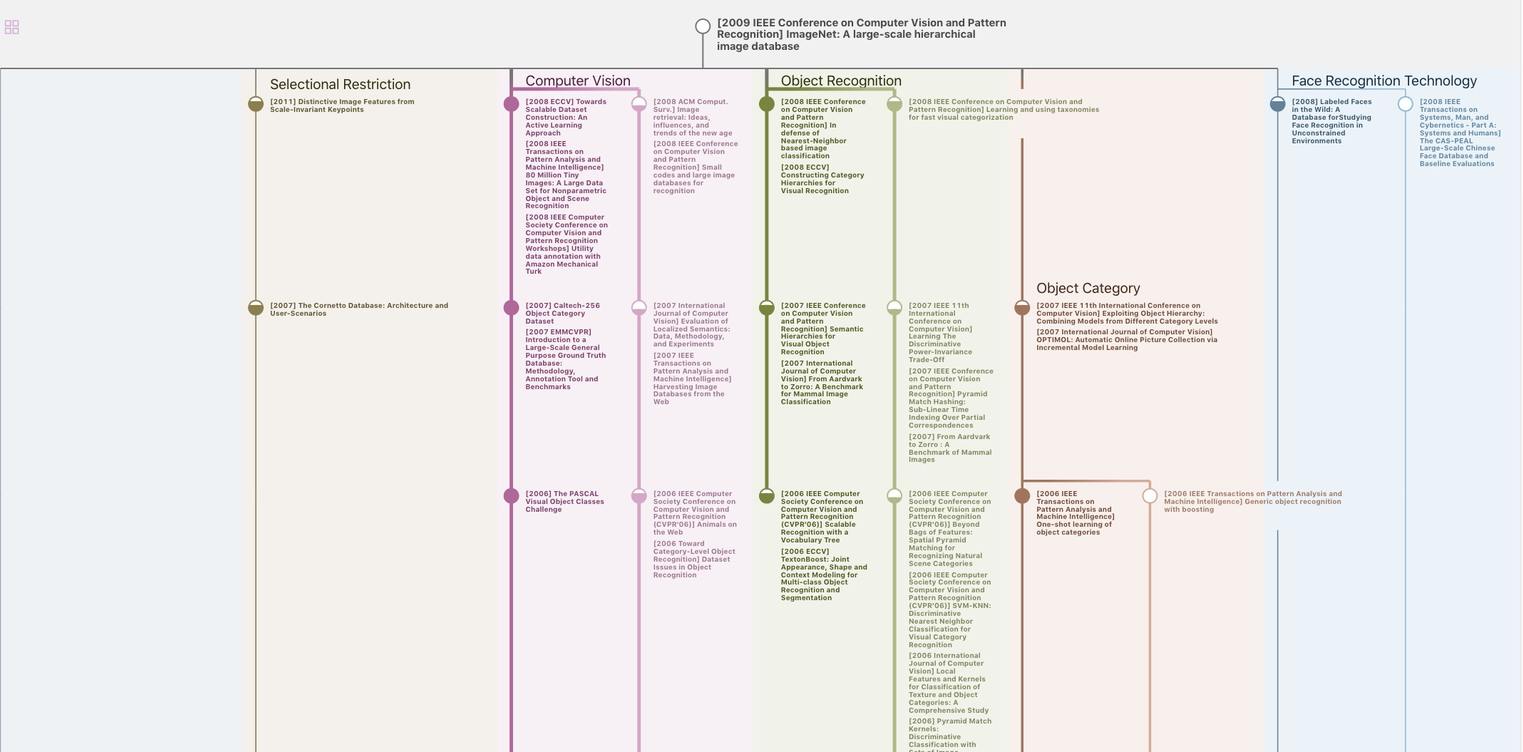
生成溯源树,研究论文发展脉络
Chat Paper
正在生成论文摘要