DiMO-Sparse: Differentiable Modeling and Optimization of Sparse CNN Dataflow and Hardware Architecture.
Design, Automation, and Test in Europe(2024)
摘要
Many real-world CNNs exhibit sparsity, a characteristic that has primarily been utilized in manual design processes and has received little attention in existing automatic optimization techniques. To the best of our knowledge, this paper presents the first systematic investigation of automatic dataflow and hardware optimization for sparse CNN computation. A differentiable PPA (Power Performance Area) model incorporating stochastic modeling of sparse CNN workloads is developed to enable fast nonlinear optimization solving and massively parallel local search-based discretization. Experimental results on public domain testcases demonstrate the efficacy of the proposed approach, achieving an average of 5× and 10× better PPA than the previous work for two different sparsity patterns.
更多查看译文
关键词
Convolutional Neural Network,Hardware Architecture,Sparse Convolutional Neural Networks,Sparsity Pattern,Automatic Optimization,Power Consumption,Weight Data,Exhaustive Search,Nonzero Elements,Sparse Representation,Constant Matrix,Power Estimation,Convolutional Neural Network Layers,Random Search,Buffer Size,Hardware Resources,Decrease In Latency,Loop Order,Total Latency,Simultaneous Selection,Sparse Format,Traffic Estimation,Hardware Constraints,Input Array,Integer Solution,Density Of Elements,One-hot Vector,Typical Convolutional Neural Network,Sparse Feature,Reduction In Runtime
AI 理解论文
溯源树
样例
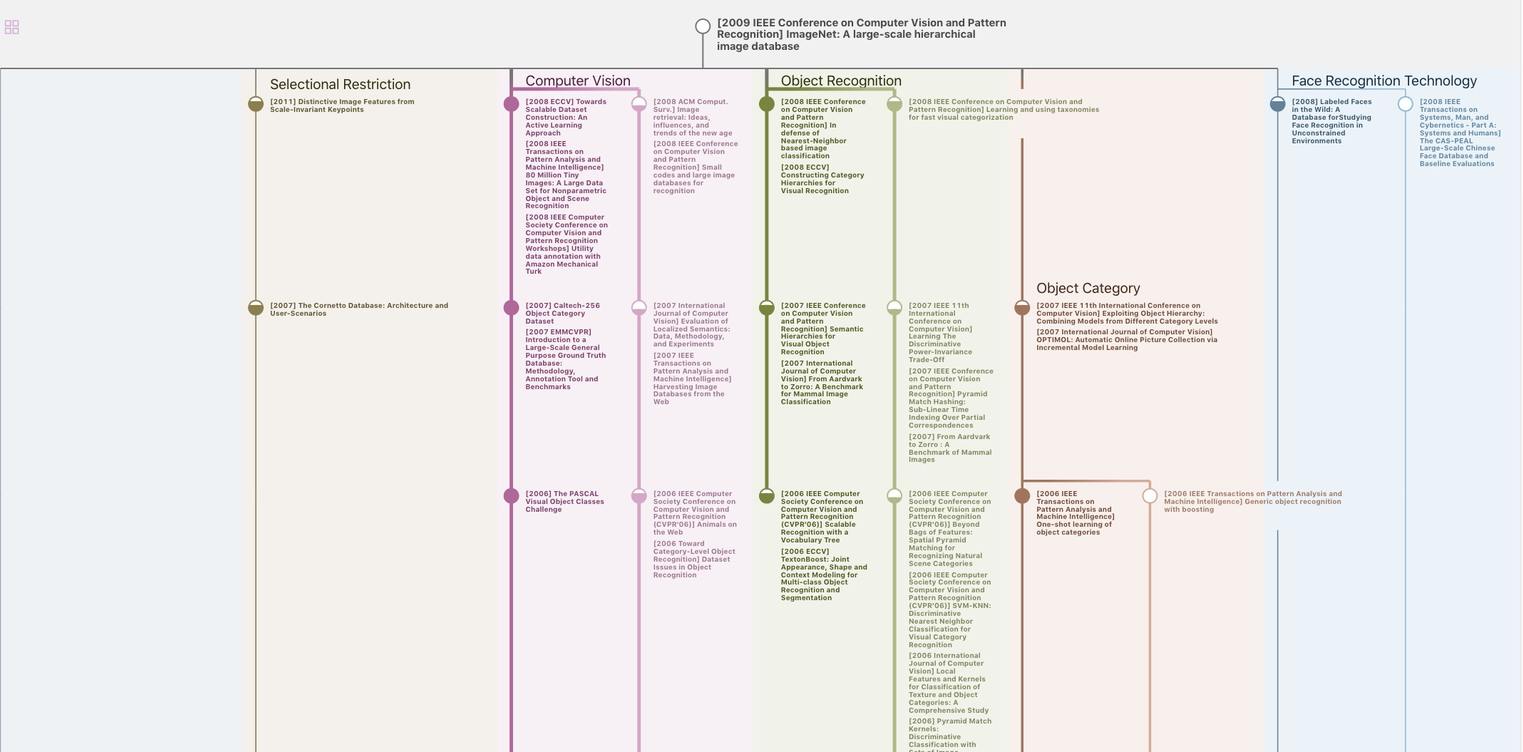
生成溯源树,研究论文发展脉络
Chat Paper
正在生成论文摘要