A Pathological Diagnosis Method for FUO Based on Multi-Path Hierarchical Classification: Model Design and Validation (Preprint)
crossref(2024)
摘要
Fever of Unknown Origin (FUO) is a significant challenge for the medical community due to its association with a wide range of diseases, the complexity of diagnosis, and the likelihood of misdiagnosis. Machine learning can extract valuable information from the extensive data of patient indicators, aiding doctors in diagnosing the underlying cause of FUO. A hierarchical classification method based on multipath feature selection was proposed to address the problem that the causes of FUO are diverse and difficult to diagnose accurately. The dataset was obtained from the clinical diagnostic records of patients with FUO who were admitted to the First Affiliated Hospital of Xi'an Jiaotong University between the years 2011 and 2020. The dataset contains 564 samples, with 5 broad categories of etiologies and subcategories, totaling 16 precise etiologies. An intelligent diagnostic algorithm for FUO was constructed based on hierarchical classification to identify 16 precise etiologies and two improvements were made: (1) A multi-path prediction model to minimize the probability of error propagation; (2) A utilization of L1,2 regularization constraint to effectively identify the most suitable subset of features to eliminate redundancy and interference in the hierarchical classification process. To confirm the proposed method's validity, we conducted ablation experiments and compared it with other flat and hierarchical classification algorithms. According to the ablation experiments, the proposed method achieves the optimal result when the intermediate path is 3, with an accuracy of 72.35%, FH of 85.01%, and FLCA of 83.29%. This performance is superior to the traditional single-path hierarchical classification, showing improvements of 5.69%, 2.89%, and 3.39%, respectively. The feature selection based on the L1,2 regularization constraint can further enhance the model's performance. The best results were achieved by filtering 25% of the features, with an accuracy of 76.08%, FH of 86.72%, and FLCA of 85.39%. Based on the comparison experiments, the proposed method outperforms the seven flat algorithms and four hierarchical classification algorithms that were compared. For example, the accuracy can be improved by at least 5.39% compared to ELM and up to 24.7% compared to KNN. The proposed algorithm greatly improves the classification performance compared to existing machine learning algorithms. It can better predict the cause of FUO, assisting physicians in their work.
更多查看译文
AI 理解论文
溯源树
样例
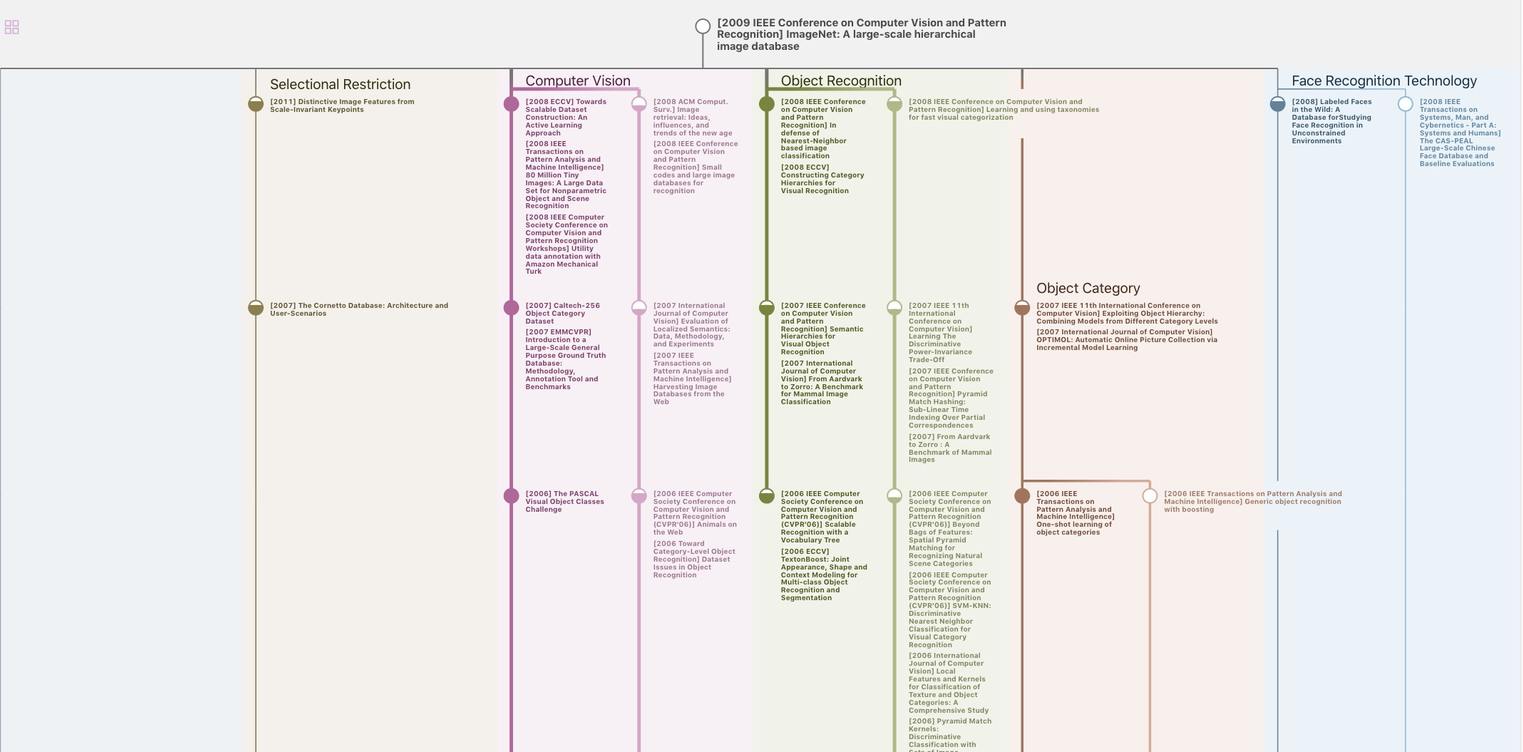
生成溯源树,研究论文发展脉络
Chat Paper
正在生成论文摘要