Interpretability of Language Models Via Task Spaces
Annual Meeting of the Association for Computational Linguistics(2024)
摘要
The usual way to interpret language models (LMs) is to test their performanceon different benchmarks and subsequently infer their internal processes. Inthis paper, we present an alternative approach, concentrating on the quality ofLM processing, with a focus on their language abilities. To this end, weconstruct 'linguistic task spaces' – representations of an LM's languageconceptualisation – that shed light on the connections LMs draw betweenlanguage phenomena. Task spaces are based on the interactions of the learningsignals from different linguistic phenomena, which we assess via a method wecall 'similarity probing'. To disentangle the learning signals of linguisticphenomena, we further introduce a method called 'fine-tuning via gradientdifferentials' (FTGD). We apply our methods to language models of threedifferent scales and find that larger models generalise better to overarchinggeneral concepts for linguistic tasks, making better use of their sharedstructure. Further, the distributedness of linguistic processing increases withpre-training through increased parameter sharing between related linguistictasks. The overall generalisation patterns are mostly stable throughouttraining and not marked by incisive stages, potentially explaining the lack ofsuccessful curriculum strategies for LMs.
更多查看译文
AI 理解论文
溯源树
样例
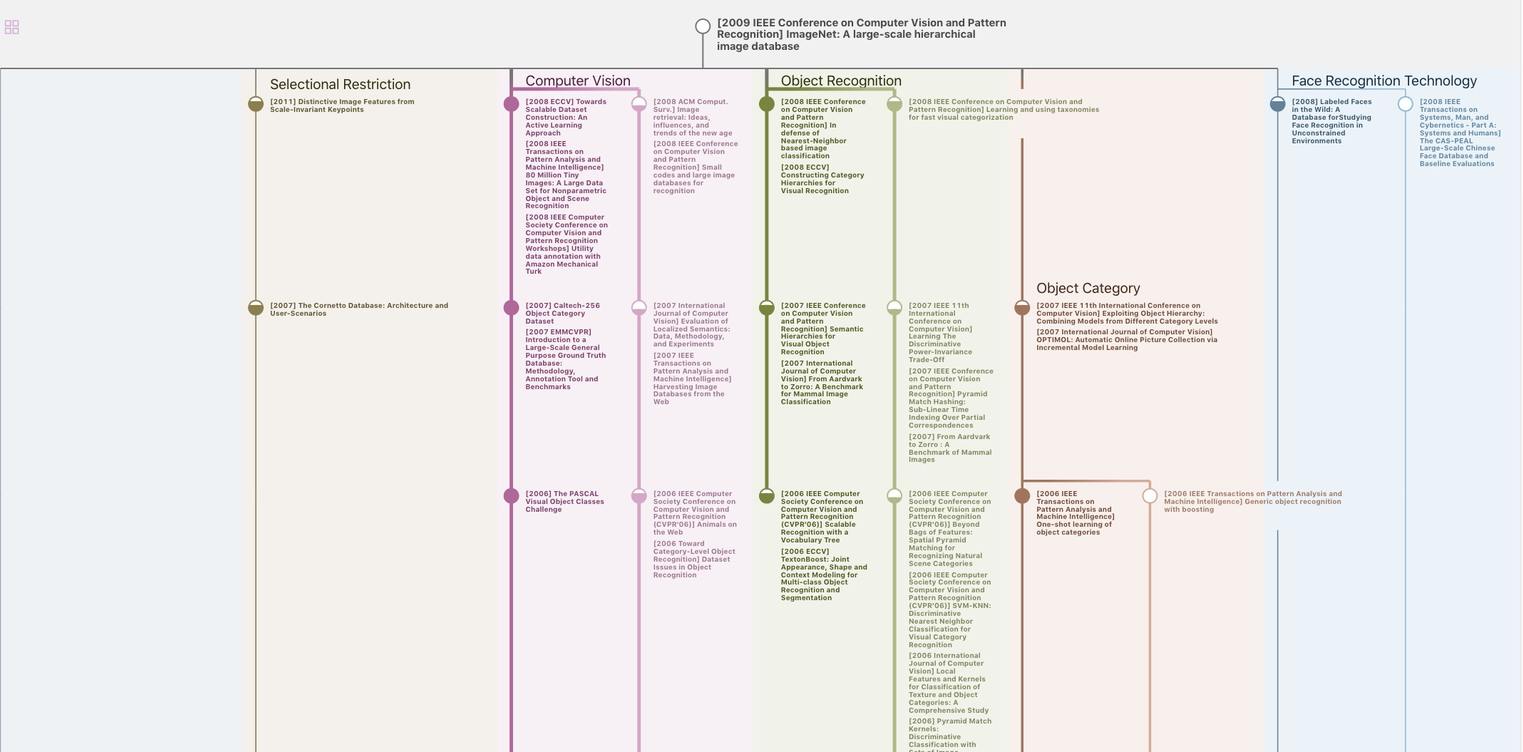
生成溯源树,研究论文发展脉络
Chat Paper
正在生成论文摘要