Emotion recognition using footstep-induced floor vibration signals
Proceedings of the 14th International Workshop on Structural Health Monitoring(2023)
摘要
Structural vibrations induced by human footsteps contain rich information that can be used for a wide range of applications, including occupant identification, localization, activity recognition, and health and emotional state estimation. Among these, emotion recognition holds great potential for improving smart buildings by enabling mental health monitoring and human-centric services. Existing emotion recognition approaches use cameras, wearables, and mobile devices to capture people’s changing gait patterns under various emotional states. However, these approaches come with corresponding drawbacks, such as being limited by visual obstructions and requiring users to carry devices that cause discomfort. To overcome these drawbacks, we introduce a new emotion recognition approach using footstep-induced structural vibration signals. The main intuition of this approach is that people’s gait patterns change under various emotions [1], thus inducing distinct structural vibration patterns as they walk. Compared to other methods, our approach is non-intrusive, insensitive to visual obstructions, and has fewer perceived-privacy concerns. The main research challenge in developing our approach is that emotions have both explicit and implicit effects on gait, making the explicit gait parameters insufficient to describe such a complex relationship. To this end, we develop a set of emotionsensitive features from the vibration signals, including gait parameters, sequential features, and time-frequency spectrum features to capture both the explicit and implicit effects of emotion on gait. To better integrate multiple types of features, we develop the fully-connected layer, the long-short-term-memory (LSTM) layer, and the convolutional layer to extract information from the features and a multilayer perceptron to estimate emotion. Our approach is evaluated in a real-world walking experiment involving 5 participants with over 100 minutes of footstep-induced floor vibration signals. Our results show that our approach achieves a mean absolute error of 1.33 for valence score estimation and 1.26 for arousal score estimation out of an overall score range of 1 to 9, which has an accuracy of 72% for High / Low valence classification and 82% for High / Low arousal for emotion classification.
更多查看译文
AI 理解论文
溯源树
样例
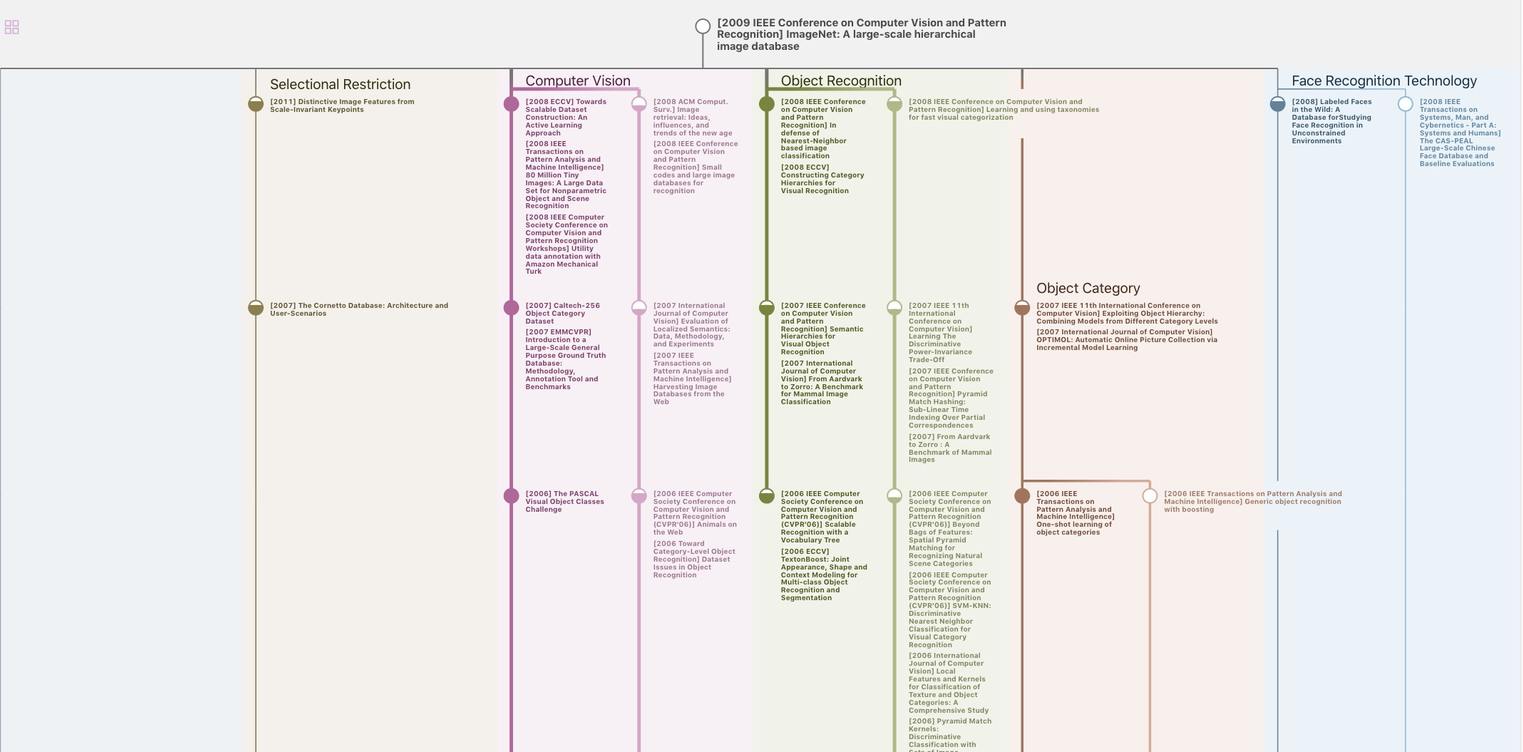
生成溯源树,研究论文发展脉络
Chat Paper
正在生成论文摘要