Neural Disparity Refinement
IEEE Transactions on Pattern Analysis and Machine Intelligence(2024)
摘要
We propose a framework that combines traditional, hand-crafted algorithms and recent advances in deep learning to obtain high-quality, high-resolution disparity maps from stereo images. By casting the refinement process as a continuous feature sampling strategy, our neural disparity refinement network can estimate an enhanced disparity map at any output resolution. Our solution can process any disparity map produced by classical stereo algorithms, as well as those predicted by modern stereo networks or even different depth-from-images approaches, such as the COLMAP structure-from-motion pipeline. Nonetheless, when deployed in the former configuration, our framework performs at its best in terms of zero-shot generalization from synthetic to real images. Moreover, its continuous formulation allows for easily handling the unbalanced stereo setup very diffused in mobile phones.
更多查看译文
关键词
Stereo Matching,Deep Learning,Domain Generalization
AI 理解论文
溯源树
样例
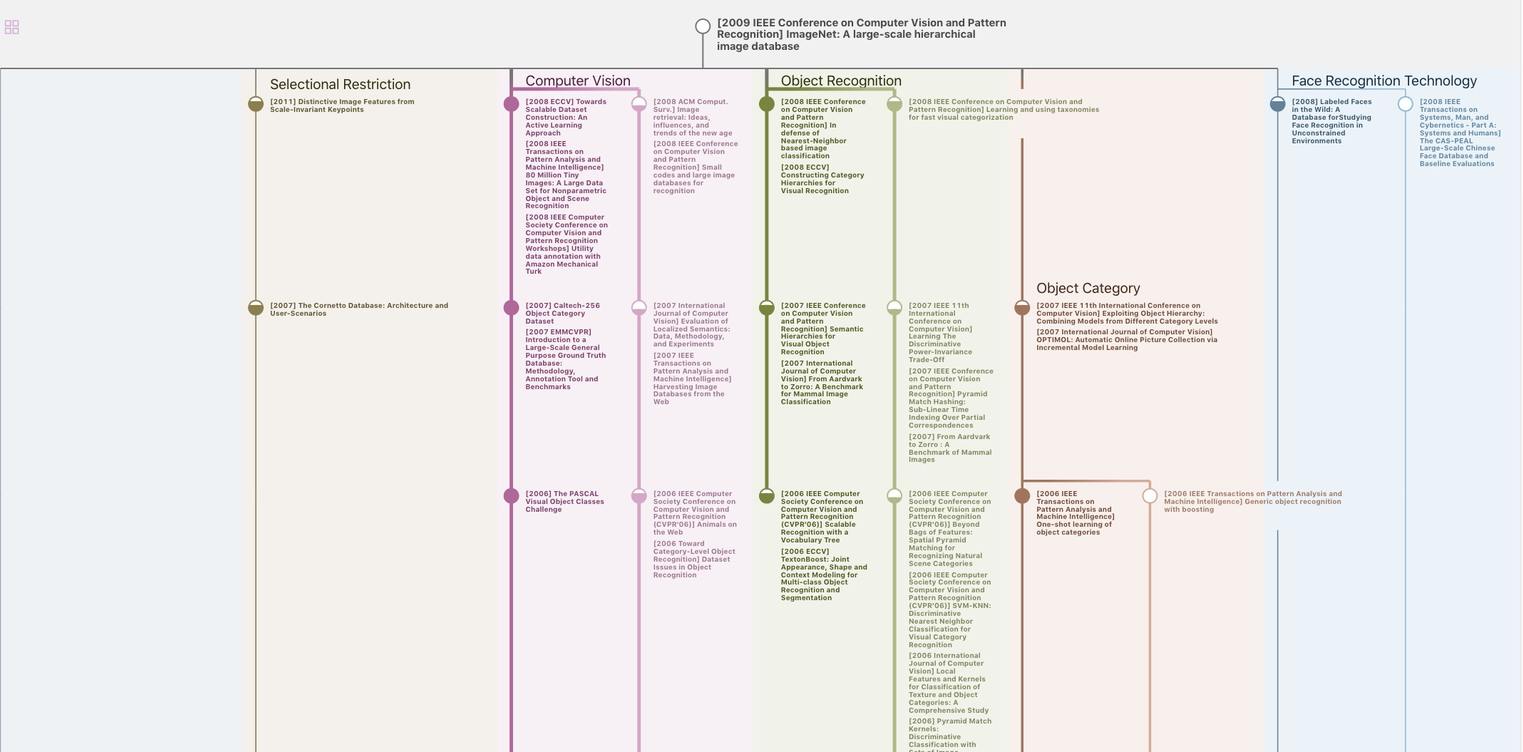
生成溯源树,研究论文发展脉络
Chat Paper
正在生成论文摘要