Keyword-Aware Relative Spatio-Temporal Graph Networks for Video Question Answering
IEEE TRANSACTIONS ON MULTIMEDIA(2024)
摘要
The main challenge in video question answering (VideoQA) is to capture and understand the complex spatial and temporal relations between objects based on given questions. Existing graph-based methods for VideoQA usually ignore keywords in questions and employ a simple graph to aggregate features without considering relative relations between objects, which may lead to inferior performance. In this paper, we propose a Keyword-aware Relative Spatio-Temporal (KRST) graph network for VideoQA. First, to make question features aware of keywords, we employ an attention mechanism to assign high weights to keywords during question encoding. The keyword-aware question features are then used to guide video graph construction. Second, because relations are relative, we integrate the relative relation modeling to better capture the spatio-temporal dynamics among object nodes. Moreover, we disentangle the spatio-temporal reasoning into an object-level spatial graph and a frame-level temporal graph, which reduces the impact of spatial and temporal relation reasoning on each other. Extensive experiments on the TGIF-QA, MSVD-QA and MSRVTT-QA datasets demonstrate the superiority of our KRST over multiple state-of-the-art methods.
更多查看译文
关键词
Cognition,Dogs,Feature extraction,Visualization,Semantics,Question answering (information retrieval),Task analysis,Video question answering,relative relation reasoning,spatial-temporal graph
AI 理解论文
溯源树
样例
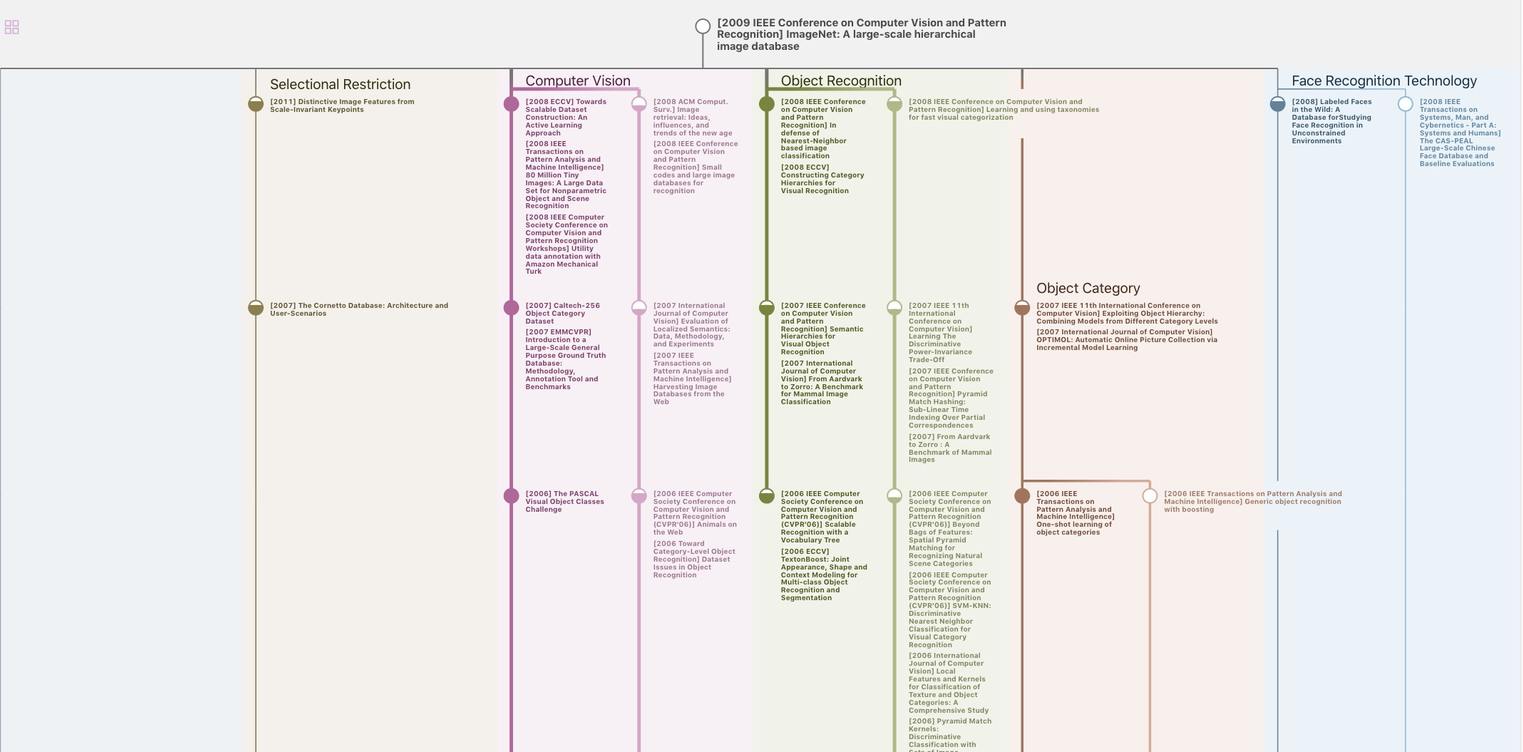
生成溯源树,研究论文发展脉络
Chat Paper
正在生成论文摘要