LET: a Local Enhancement Transformer for Low-Light Image Enhancement
JOURNAL OF ELECTRONIC IMAGING(2024)
摘要
Digital images captured under insufficient lighting conditions may suffer from issues such as low contrast and poor visual quality. However, transformers treat images as one-dimensional sequential data, lacking the modeling of local visual structures, thus resulting in a shortage of feature extraction for degraded low-light images. In addition, transformer-based methods require longer training schedules to achieve better performance. We introduce a novel method, named local enhancement transformer (LET). By incorporating convolutions into transformer blocks, we improve our model's capability to extract features from degraded low-light images. Furthermore, we propose a multi-level enhancement block to adaptively fuse features with learnable correlations among different levels. With the support of these two designs, LET can extract more useful features while requiring less training time. Experimental evaluations conducted on LOL and MIT-5K datasets prove that LET is superior to the state-of-the-art. (c) 2024 SPIE and IS&T
更多查看译文
关键词
transformer,gated mechanism,CNN,computer vision,image enhancement
AI 理解论文
溯源树
样例
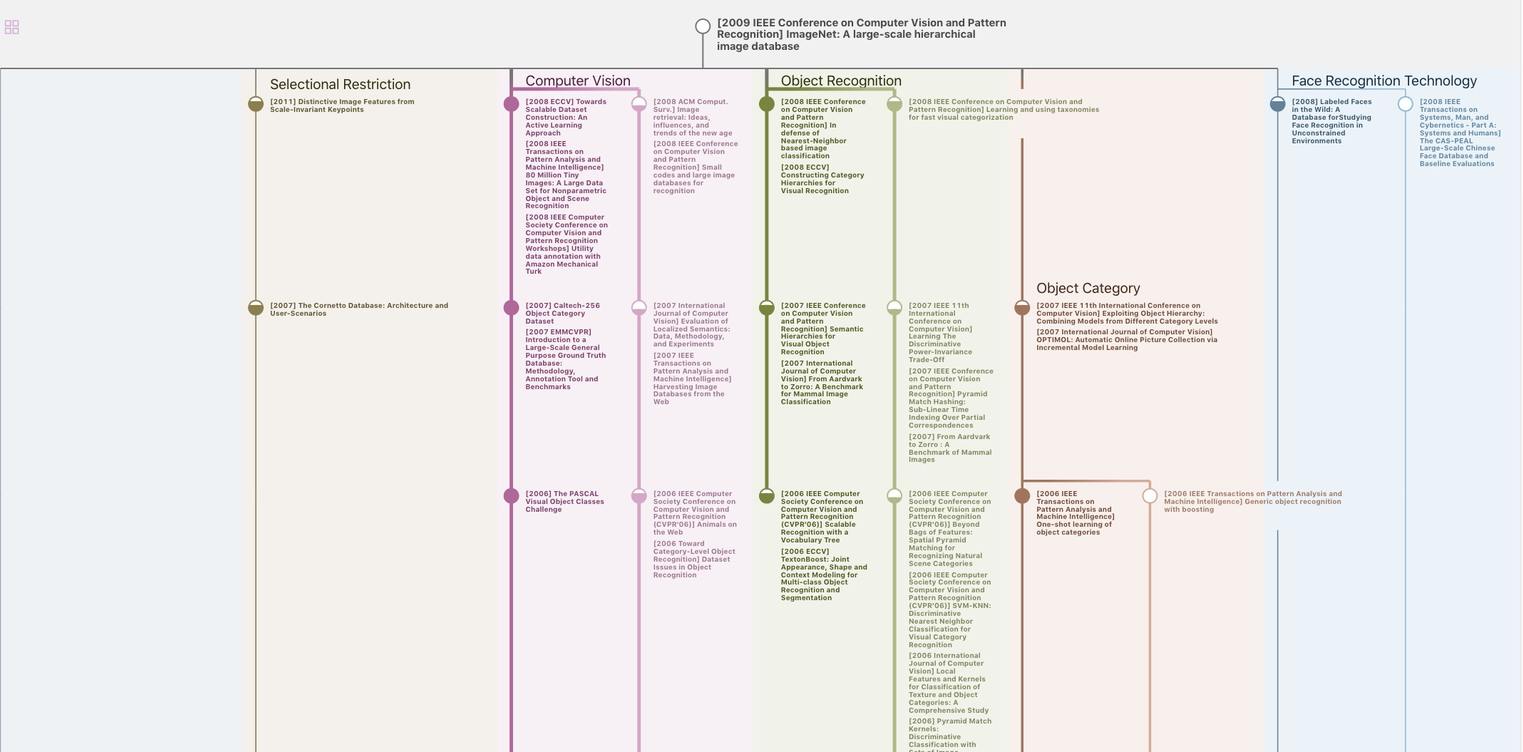
生成溯源树,研究论文发展脉络
Chat Paper
正在生成论文摘要