SWIR Based Estimation of TIR Emissivity of Bare Soil Surfaces Using Deep Conditional Generative Adversarial Network in Landsat Data
PLANT AND SOIL(2024)
摘要
Land surface emissivity (LSE) is an important variable in soil studies. Although there are various remote sensing methods to estimate LSE, accurately predicting LSE still remains a major challenge. Typically, the correlation between LSE and the Visible Near Infrared bands is employed for LSE estimation. However, some studies have raised some concerns about this correlation, especially in bare soil areas. Therefore, it is necessary to conduct further investigation to determine if there exists a nonlinear relationship between the LSE and other spectral bands, which was not detected by simple linear correlation/regression. In this study, firstly, a deep Auto-encoder network has been used to investigate the correlation between LSE and other spectral bands. Subsequently, we have applied a Conditional Generative Adversarial Network (CGAN) to estimate the LSE. The proposed CGAN was trained using the Landsat and ECOSTRESS satellite datasets. The performance of the developed network was then compared with NDVI-based method on satellite/simulated-based bare soil pixels. For satellite data, the RMSE (Root Mean Squared Error) and correlation coefficient (R) between the estimated LSE using proposed CGAN and ECOSTRESS LSE are 0.005 and 0.97, respectively. For the simulated data, the RMSE and R between the estimated LSE and the simulated one are 0.01 and 0.92, respectively. The results of the deep Auto-encoder show considerable relationship between the LSE and Short-Wave Infrared bands which not be seen using simple linear correlation. In cases of satellite/simulated data of bare soils, the developed network showed superior performance compared to NDVI-based method.
更多查看译文
关键词
Land Surface Emissivity,CGAN-based Autoencoder,Short-Wave Infrared (SWIR),Landsat
AI 理解论文
溯源树
样例
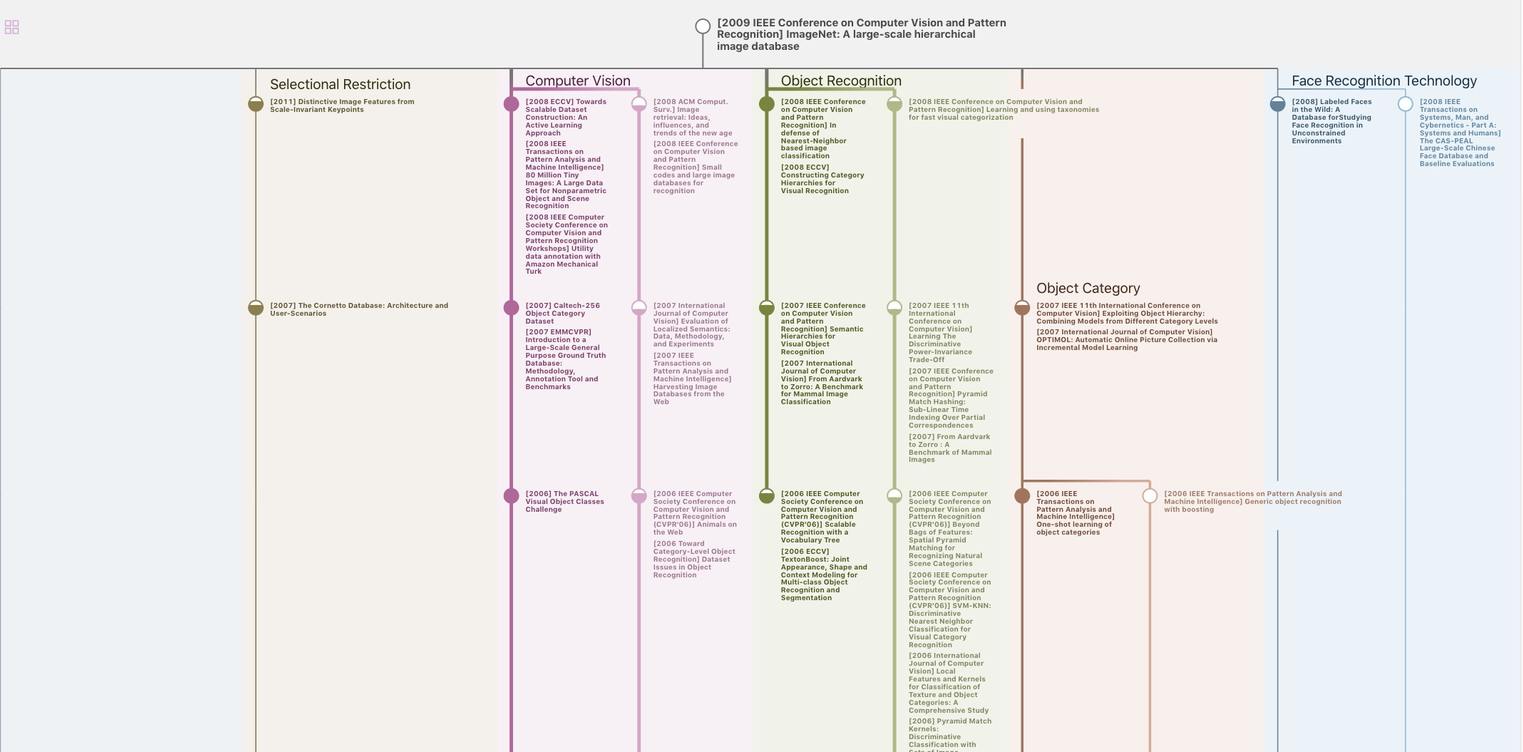
生成溯源树,研究论文发展脉络
Chat Paper
正在生成论文摘要