Gated Low-rank Adaptation for personalized Code-Switching Automatic Speech Recognition on the low-spec devices
CoRR(2024)
摘要
In recent times, there has been a growing interest in utilizing personalized large models on low-spec devices, such as mobile and CPU-only devices. However, utilizing a personalized large model in the on-device is inefficient, and sometimes limited due to computational cost. To tackle the problem, this paper presents the weights separation method to minimize on-device model weights using parameter-efficient fine-tuning methods. Moreover, some people speak multiple languages in an utterance, as known as code-switching, the personalized ASR model is necessary to address such cases. However, current multilingual speech recognition models are limited to recognizing a single language within each utterance. To tackle this problem, we propose code-switching speech recognition models that incorporate fine-tuned monolingual and multilingual speech recognition models. Additionally, we introduce a gated low-rank adaptation(GLoRA) for parameter-efficient fine-tuning with minimal performance degradation. Our experiments, conducted on Korean-English code-switching datasets, demonstrate that fine-tuning speech recognition models for code-switching surpasses the performance of traditional code-switching speech recognition models trained from scratch. Furthermore, GLoRA enhances parameter-efficient fine-tuning performance compared to conventional LoRA.
更多查看译文
关键词
automatic speech recognition,code-switching,parameter-efficient fine-tuning,personalized,on-device
AI 理解论文
溯源树
样例
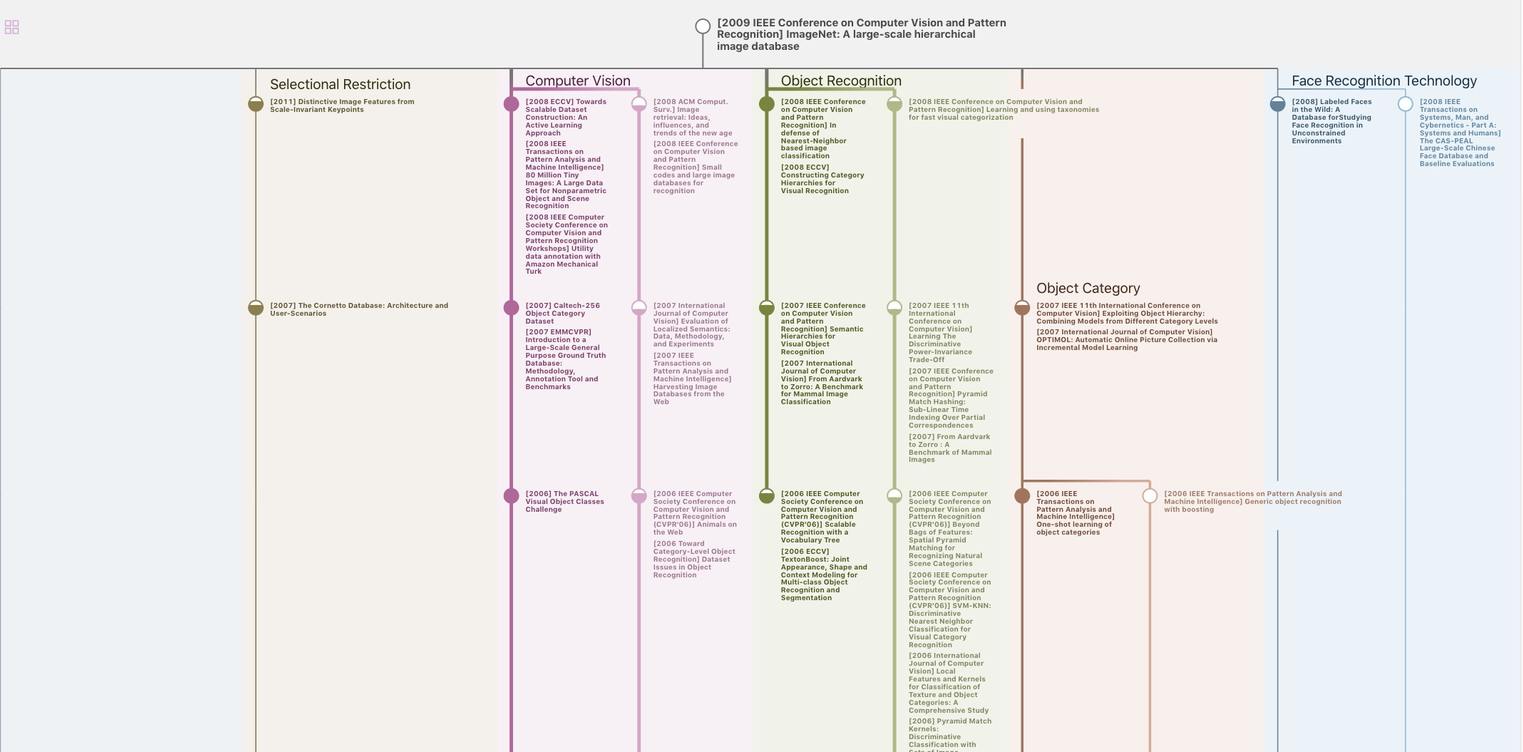
生成溯源树,研究论文发展脉络
Chat Paper
正在生成论文摘要