Adaptive augmentation framework for domain independent few shot learning
Knowledge-Based Systems(2024)
Abstract
Few-Shot learning is a research area of machine learning, which aims to develop a prediction model based on a limited set of training instances. In contrast to human learners, who are able to quickly learn and adapt to new tasks, machine learning models require large amounts of training instances in order to generalize efficiently. Image augmentation provides a potential solution to this challenge in few-shot learning by enlarging the training dataset. However, an excessive and uncontrollable enlargement of the initial dataset may potentially add noise, which could significantly impair the learning efficacy, especially in the few-shot context. Our motivation lies in the fact that since the least confident instances are the hardest to classify, performing targeted augmentations on these instances could efficiently enhance the limited representational sample space in a few-shot context. In this work, we propose a new augmentation-based prediction framework, which adaptively enlarges the few-shot training samples by performing targeted image augmentations for the hardest to identify instances. Given the inherently limited size of data, their proper identification is challenging. Therefore, we adopt a Least Confident Augmentation strategy based on the output confidences of an embedding-based estimator. In addition, we introduce an adaptive cleaning step in order to remove the potential noise added during the targeted augmentations. By this approach, we managed to achieve superior performance results compared to state-of-the-art approaches in few-shot learning tasks, validated under an experimental evaluation setup which adopts the challenging but realistic domain-independence scenario.
MoreTranslated text
Key words
Few-shot learning,Meta-learning,Image augmentation,Machine learning,Convolutional neural networks
AI Read Science
Must-Reading Tree
Example
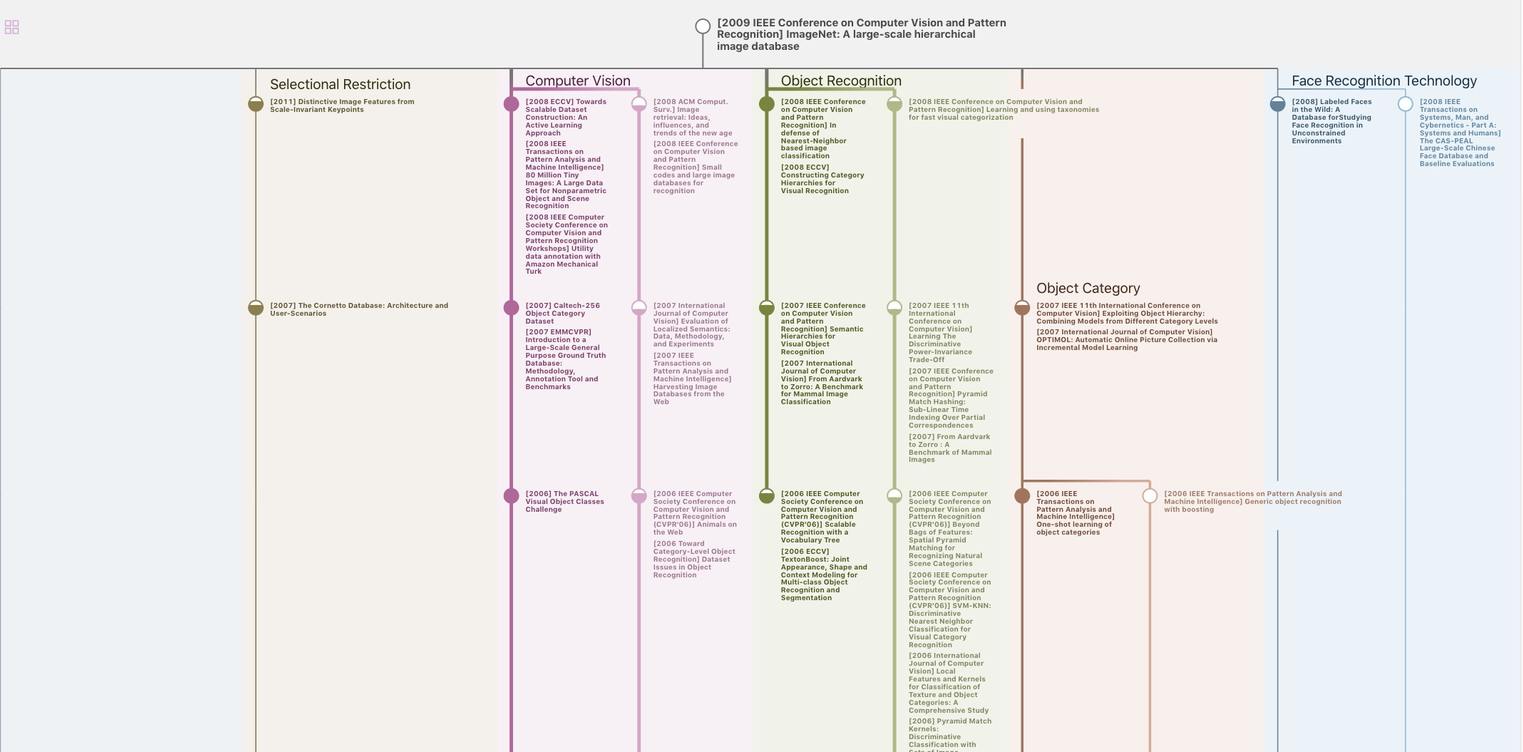
Generate MRT to find the research sequence of this paper
Chat Paper
Summary is being generated by the instructions you defined