Enhancing Mix Proportion Design of Low Carbon Concrete for Shield Segment Using a Combination of Bayesian Optimization-Ngboost and NSGA-III Algorithm
Journal of cleaner production(2024)
摘要
The demand for segment concrete increases rapidly with the expansion of urban rail transit and underground space, which may lead to carbon emissions (CE). In the production of segment concrete, using supplement cementitious materials (SCM) can be used to reduce CE instead of cement. However, SCM contents are usually determined by many orthogonal experiments, which are often time-consuming and only consider the limited experimental variables. Therefore, a novel hybrid intelligent algorithm combining Bayesian optimization (BO), natural gradient boosting (NGBoost) and non-dominated sorting genetic algorithm (NSGA)-III is proposed, which overcomes these disadvantages. Firstly, NGBoost model after hyperparameter optimization using BO is used to establish a nonlinear mapping relationship between segment concrete mix proportion and performance, which was used as the fitness function of the non-dominated sorting genetic algorithm (NSGA-III). Secondly, the optimal Pareto solutions were obtained through NSGA-III, and the reasonable range of segment concrete mixing proportion was obtained. Finally, a segment concrete production case was used to verify the effectiveness of the proposed algorithm. The results showed that the proposed hybrid algorithm combining proposed scheme decision strategy can obtain an optimal design scheme for concrete mix proportion. In addition, the comprehensive optimization rate of the obtained optimal scheme compared to the experimental optimal group was 11.5%. Moreover, the amount of phosphorous slag (PS) and granulated blast furnace slag (GBFS) in the optimal scheme was 26%, with a mass ratio of 1:2. Compared to the experimental optimal group, the optimal scheme can reduce the cost and CE per cubic meter by 31.64 yuan and 31.04 kg, respectively. Furthermore, the hybrid algorithm proposed can accurately obtains an optimal amount of SCM, which could be used as a supporting tool to reduce the production cost and CE of segment concrete. Overall, this research contributes to combining machine learning algorithms with actual production processes, which overcomes the data limitations of traditional experiments. It also provides a new intelligent approach and reference cases for the low-carbon design and sustainable development of building materials such as concrete.
更多查看译文
关键词
Low-carbon concrete,Mix design,Concrete performance,Carbon emission,Multi-objective optimization,BO-NGBoost-NSGA-III
AI 理解论文
溯源树
样例
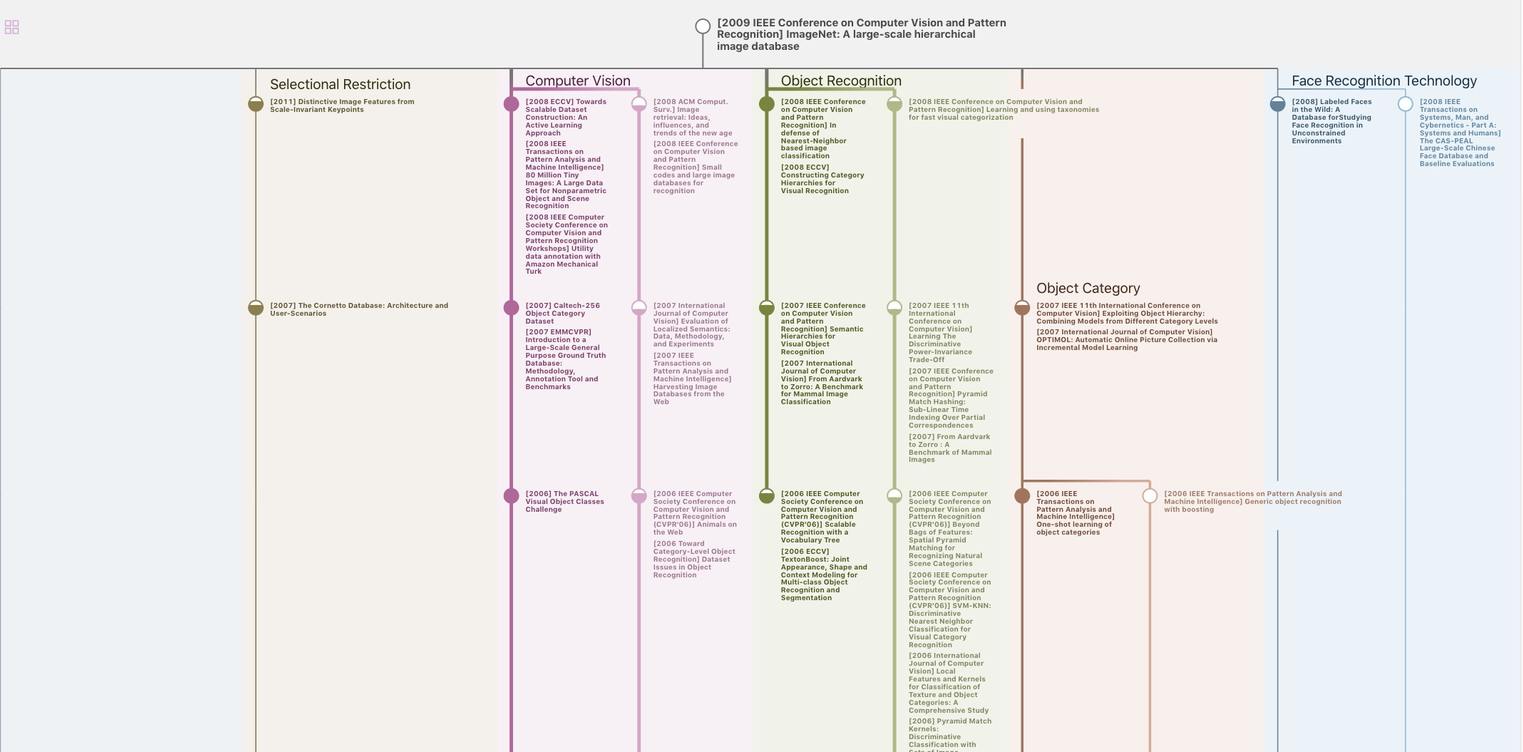
生成溯源树,研究论文发展脉络
Chat Paper
正在生成论文摘要