Predicting Land Cover Using a GIS-Based Markov Chain and Sea Level Inundation for a Coastal Area
Land(2024)
Univ North Carolina Wilmington
Abstract
New Hanover County, North Carolina, has been experiencing rapid population growth and is expected to continue this growth, leading to increased land use and development in the area. The county is also threatened by sea level rise (SLR) and its effects because of its coastal location and frequent occurrences of major storms and hurricanes. This study used a land change modeler to map the land cover change throughout the county over a period of 20 years, and predicted land cover distribution in the area in the years 2030 and 2050. Statistics revealed that the developed land in the area increased by 85 km2 between 2000 and 2010, and by 60 km2 between 2010 and 2020. Such land is predicted to increase by another 73 km2 by 2030, and 63 km2 by 2050. This increase in development is expected to occur mainly in the central area of the county and along the barrier islands. Modeling of SLR illustrated that the northwestern part of New Hanover County along the Cape Fear River, as well as the beach towns located on the barrier islands, are estimated be the most affected locations. Results indicate that sections of major highways throughout the county, including I-140 near downtown Wilmington and US-421 in Carolina Beach, may be inundated by SLR, which might delay residents during mandatory evacuations for emergency situations such as hurricanes. Some routes may be unusable, leading to traffic congestion on other routes, which may impede some residents from reaching safety before the emergency. Wrightsville Beach and Carolina Beach are estimated to have the highest levels of inundation, with 71.17% and 40.58% of their land being inundated under the most extreme SLR scenario of 3 m, respectively. The use of the present research approach may provide a practical, quick, and low-cost method in modeling rapidly growing urban areas along the eastern United States coastline and locating areas at potential risk of future SLR inundation.
MoreTranslated text
Key words
land cover,neural network,land change modeler,minimum noise fraction,digital elevation model,surface gradient,road network
求助PDF
上传PDF
View via Publisher
AI Read Science
AI Summary
AI Summary is the key point extracted automatically understanding the full text of the paper, including the background, methods, results, conclusions, icons and other key content, so that you can get the outline of the paper at a glance.
Example
Background
Key content
Introduction
Methods
Results
Related work
Fund
Key content
- Pretraining has recently greatly promoted the development of natural language processing (NLP)
- We show that M6 outperforms the baselines in multimodal downstream tasks, and the large M6 with 10 parameters can reach a better performance
- We propose a method called M6 that is able to process information of multiple modalities and perform both single-modal and cross-modal understanding and generation
- The model is scaled to large model with 10 billion parameters with sophisticated deployment, and the 10 -parameter M6-large is the largest pretrained model in Chinese
- Experimental results show that our proposed M6 outperforms the baseline in a number of downstream tasks concerning both single modality and multiple modalities We will continue the pretraining of extremely large models by increasing data to explore the limit of its performance
Upload PDF to Generate Summary
Must-Reading Tree
Example
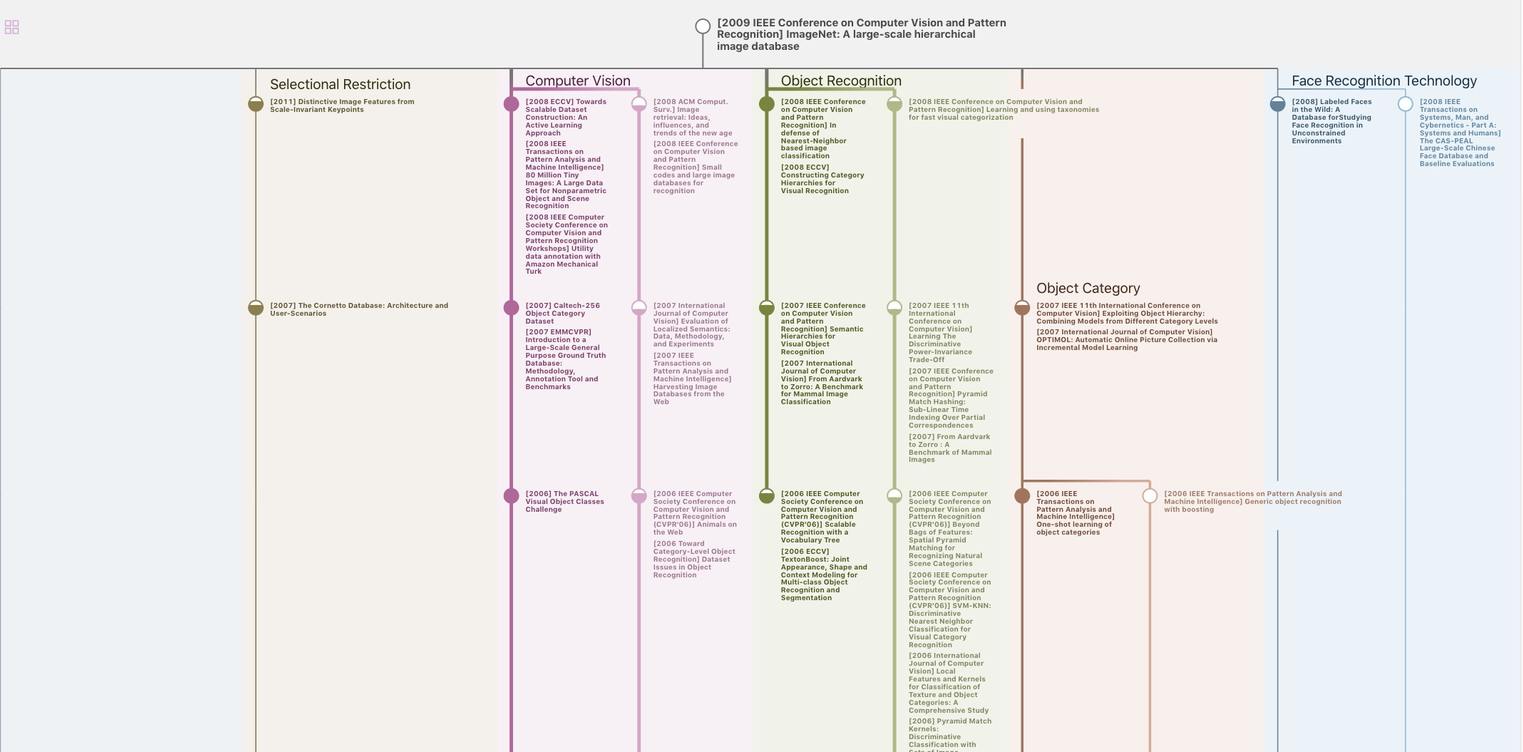
Generate MRT to find the research sequence of this paper
Related Papers
Utilizing DEMs derived from LIDAR data to analyze morphologic change in the North Carolina coastline
2003
被引用203 | 浏览
2007
被引用55 | 浏览
2018
被引用160 | 浏览
2018
被引用26 | 浏览
2018
被引用235 | 浏览
2021
被引用19 | 浏览
2021
被引用13 | 浏览
2021
被引用16 | 浏览
2017
被引用19 | 浏览
2022
被引用58 | 浏览
Data Disclaimer
The page data are from open Internet sources, cooperative publishers and automatic analysis results through AI technology. We do not make any commitments and guarantees for the validity, accuracy, correctness, reliability, completeness and timeliness of the page data. If you have any questions, please contact us by email: report@aminer.cn
Chat Paper