Data-driven Dirichlet Sampling on Manifolds for Structural Health Monitoring
Journal of the Brazilian Society of Mechanical Sciences and Engineering(2024)
摘要
The practical limitation of applying machine learning to structural health monitoring (SHM) is the availability of sufficient experimental data for training. However, obtaining an extensive training database can be expensive or complicated. Incomplete datasets can lead to overfitting, incorrect classification, or poorly generalized results. Various approaches have been proposed to overcome this limitation, including data augmentation techniques based on numerical models or data-driven methods. This paper presents a novel data-driven strategy for improving feature-SHM classification, utilizing manifold sampling with a Dirichlet distribution. The proposed approach respects the underlying manifold structure of the original datasets of the features. Two examples illustrate the method’s application: the Z-24 bridge dataset and a three-story building structure dataset from the Los Alamos National Laboratory. In both cases, the technique efficiently generates samples with minimal computational effort, facilitating data augmentation to enhance the training of unsupervised and/or supervised methods for SHM purposes.
更多查看译文
关键词
Sampling on manifolds,Dirichlet distribution,Data-driven,Data augmentation,Damage detection
AI 理解论文
溯源树
样例
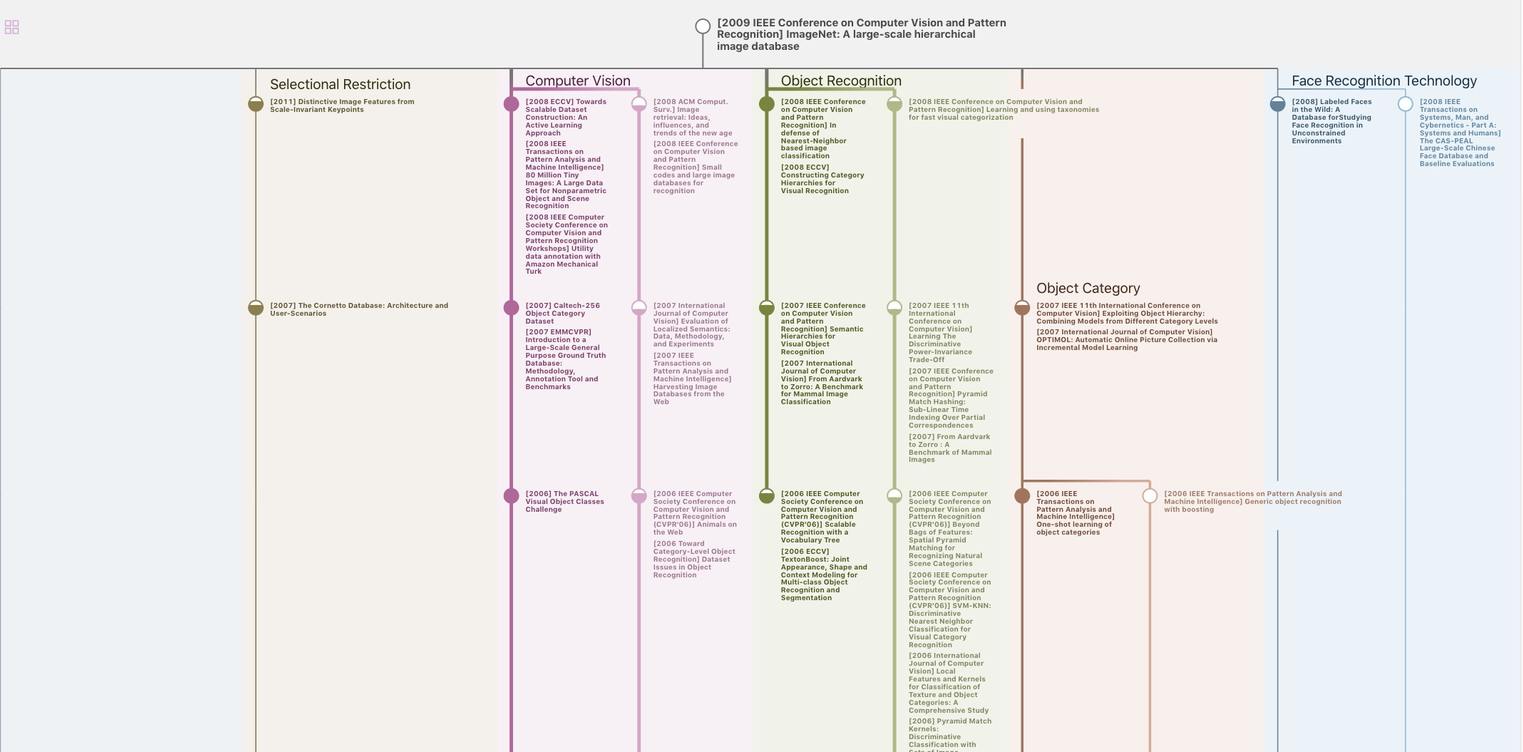
生成溯源树,研究论文发展脉络
Chat Paper
正在生成论文摘要