DAFNet: Dynamic Auxiliary Fusion for Sequential Model Editing in Large Language Models
Findings of the Association for Computational Linguistics ACL 2024(2024)
摘要
Recently, while large language models (LLMs) have demonstrated impressiveresults, they still suffer from hallucination, i.e., the generation of falseinformation. Model editing is the task of fixing factual mistakes in LLMs; yet,most previous works treat it as a one-time task, paying little attention toever-emerging mistakes generated by LLMs. We address the task of sequentialmodel editing (SME) that aims to rectify mistakes continuously. A DynamicAuxiliary Fusion Network (DAFNet) is designed to enhance the semanticinteraction among the factual knowledge within the entire sequence, preventingcatastrophic forgetting during the editing process of multiple knowledgetriples. Specifically, (1) for semantic fusion within a relation triple, weaggregate the intra-editing attention flow into auto-regressive self-attentionwith token-level granularity in LLMs. We further leverage multi-layer diagonalinter-editing attention flow to update the weighted representations of theentire sequence-level granularity. (2) Considering that auxiliary parametersare required to store the knowledge for sequential editing, we construct a newdataset named DAFSet, fulfilling recent, popular, long-tail and robustproperties to enhance the generality of sequential editing. Experiments showDAFNet significantly outperforms strong baselines in single-turn and sequentialediting. The usage of DAFSet also consistently improves the performance ofother auxiliary network-based methods in various scenarios
更多查看译文
AI 理解论文
溯源树
样例
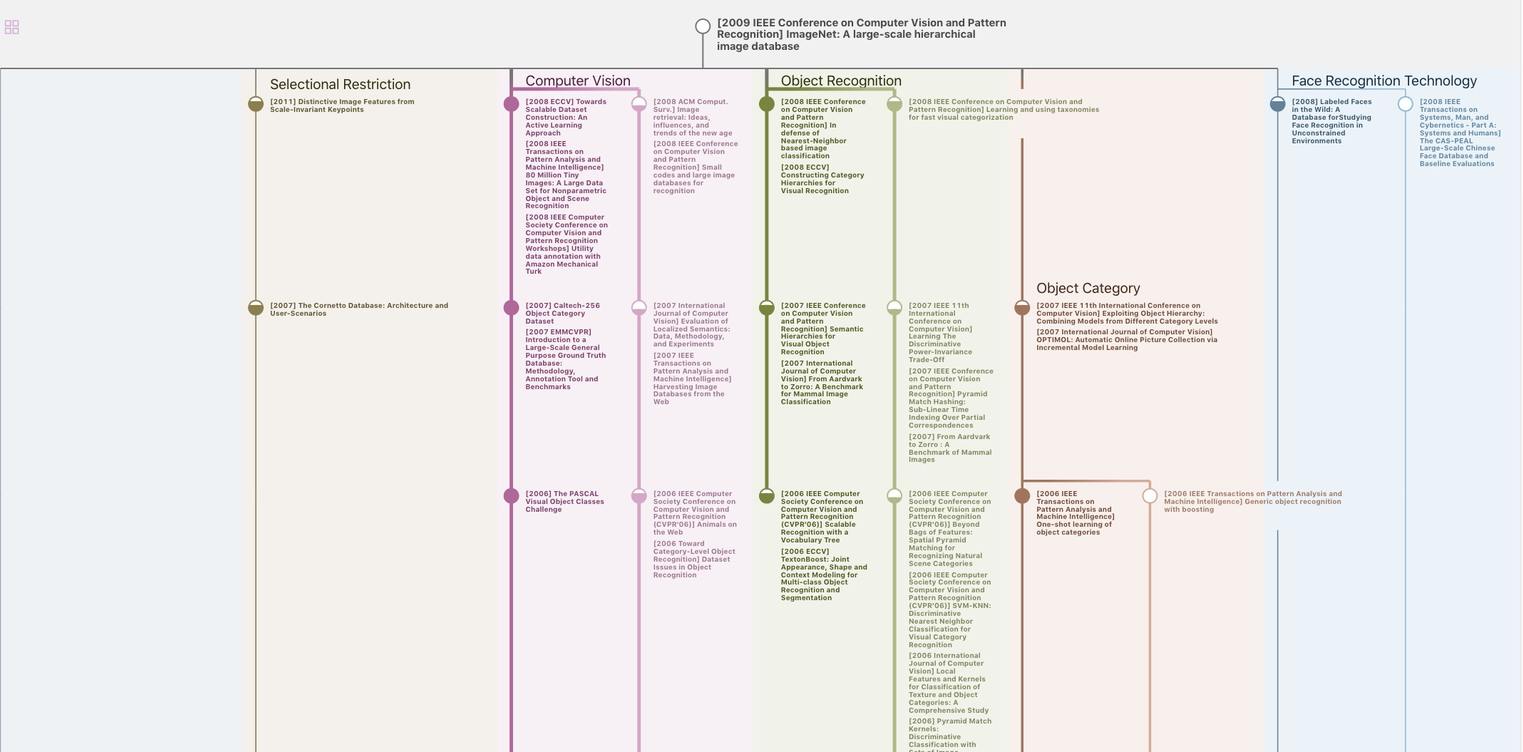
生成溯源树,研究论文发展脉络
Chat Paper
正在生成论文摘要