Enhancing Performance for Highly Imbalanced Medical Data Via Data Regularization in a Federated Learning Setting
AIiH (2)(2024)
摘要
The increased availability of medical data has significantly impactedhealthcare by enabling the application of machine / deep learning approaches invarious instances. However, medical datasets are usually small and scatteredacross multiple providers, suffer from high class-imbalance, and are subject tostringent data privacy constraints. In this paper, the application of a dataregularization algorithm, suitable for learning under high class-imbalance, ina federated learning setting is proposed. Specifically, the goal of theproposed method is to enhance model performance for cardiovascular diseaseprediction by tackling the class-imbalance that typically characterizesdatasets used for this purpose, as well as by leveraging patient data availablein different nodes of a federated ecosystem without compromising their privacyand enabling more resource sensitive allocation. The method is evaluated acrossfour datasets for cardiovascular disease prediction, which are scattered acrossdifferent clients, achieving improved performance. Meanwhile, its robustnessunder various hyperparameter settings, as well as its ability to adapt todifferent resource allocation scenarios, is verified.
更多查看译文
AI 理解论文
溯源树
样例
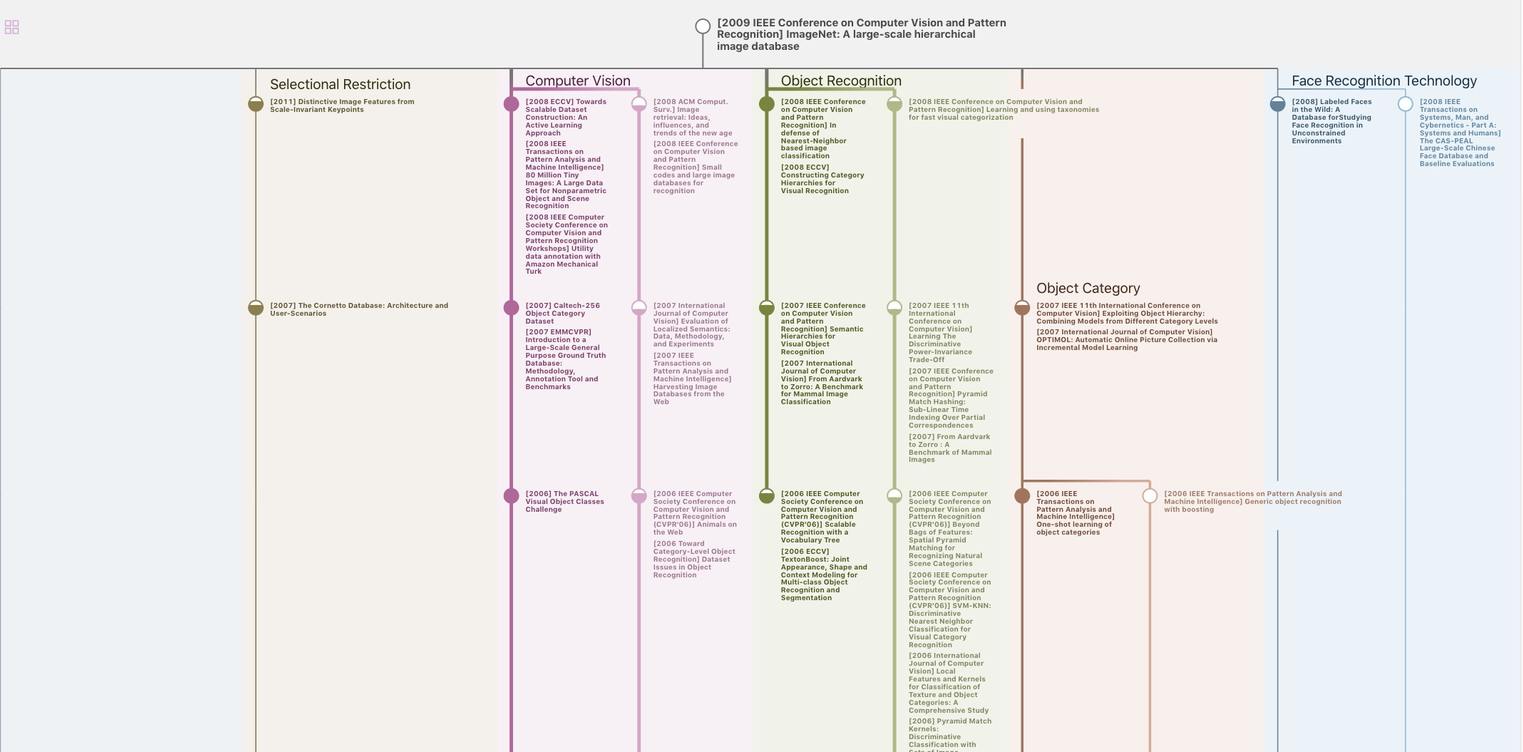
生成溯源树,研究论文发展脉络
Chat Paper
正在生成论文摘要