Time Phase Selection and Accuracy Analysis for Predicting Winter Wheat Yield Based on Time Series Vegetation Index
REMOTE SENSING(2024)
关键词
winter wheat,remote sensing,temporal selection,growth stages,interpolation,random forest,yield estimation
AI 理解论文
溯源树
样例
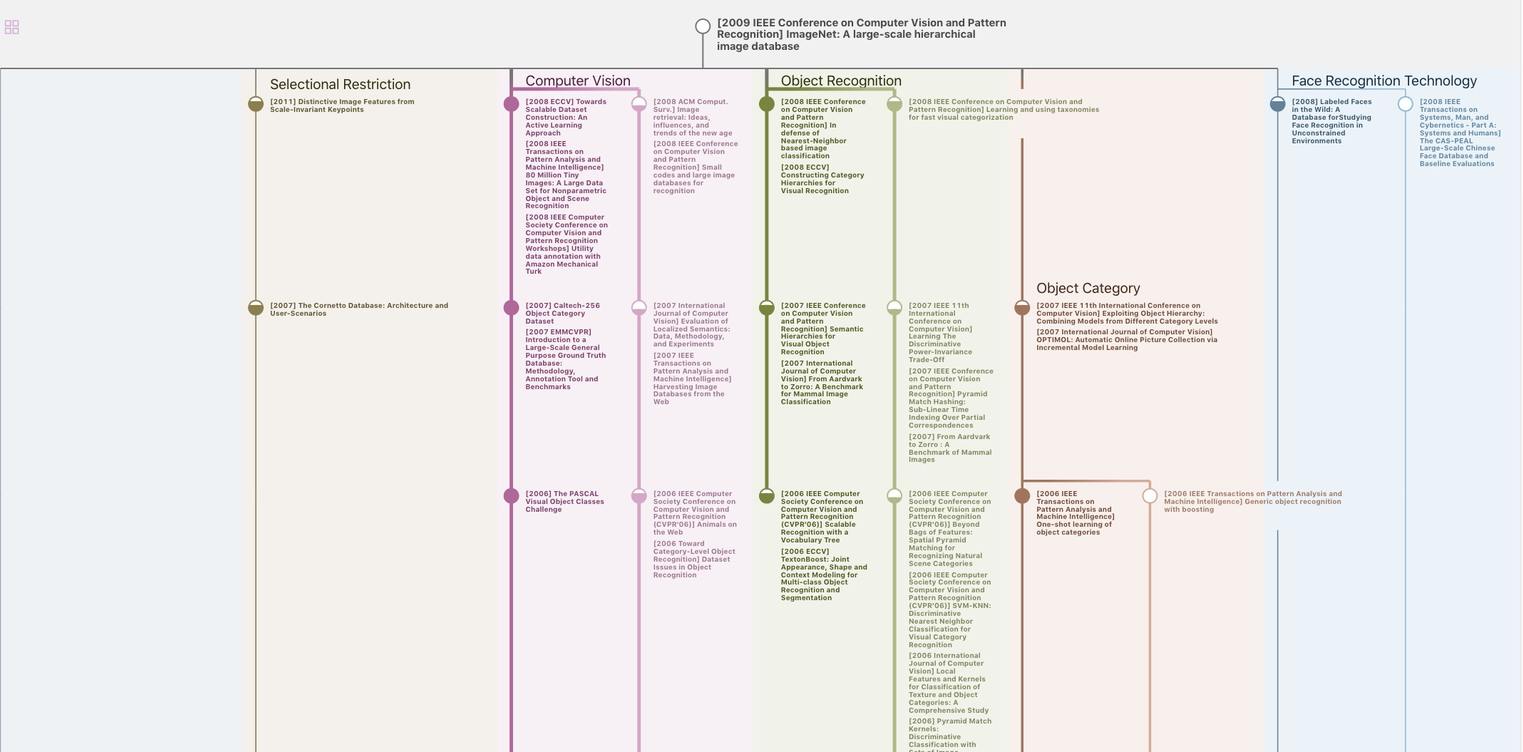
生成溯源树,研究论文发展脉络
Chat Paper
正在生成论文摘要