Optimizing Asphalt Mix Design Through Predicting Volumetric Properties Using Interpretable Machine Learning
Powder technology(2024)
摘要
Traditional asphalt mix design methods primarily rely on experience, consuming immense time and resources. Currently, advanced machine learning (ML) method can accelerate the asphalt mix design procedure. However, historical data on asphalt mix design exhibit high variability, and the outputs of ML models are often challenging to interpret. To address these issues, this study selectively utilized outlier detection algorithms for data preprocessing and employed interpretable ML to predict and explain the volumetric properties of the mixtures, achieving an automated and rapid mix design method. A database (1525 sets of data) of volumetric properties was established based on experimental data of the engineering site. Z-score, KDE (kernel density estimation) and LOF (local outlier factor) methods were employed to detect outliers of the database. Three ML models were trained to predict the volumetric properties of mixture (air void and voids in mineral aggregate), based on material proportion, gradation morphological and material performance features. The SHAP method was used to interpret the extreme gradient boosting (XGB) model, quantifying feature importance, and aiding in decision-making. Finally, an asphalt mix design framework was proposed. The results indicate that the combination of KDE and LOF methods for outlier detection enabled the machine learning model to achieve optimal performance. The XGB model had the best prediction effect on volumetric properties. According to the SHAP method results, the influence of the critical features on the results was explained by the physical laws. The method proposed effectively reducing the design time and costs. The feasibility of the proposed design method was proved by the case study.
更多查看译文
关键词
Interpretable machine learning,Asphalt mix design,Volumetric properties,Outlier detection,SHAP method
AI 理解论文
溯源树
样例
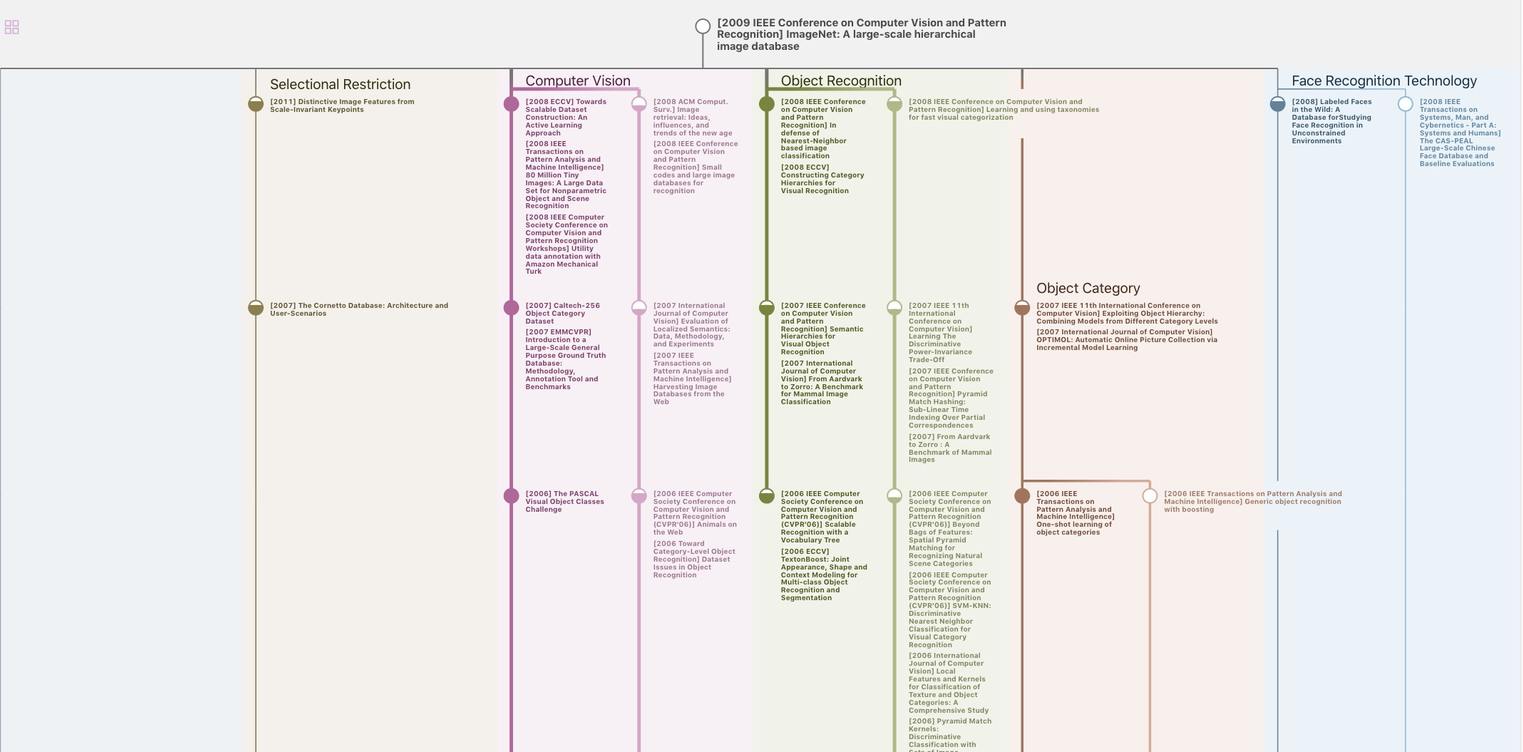
生成溯源树,研究论文发展脉络
Chat Paper
正在生成论文摘要